Predicting Employee Attrition With AI
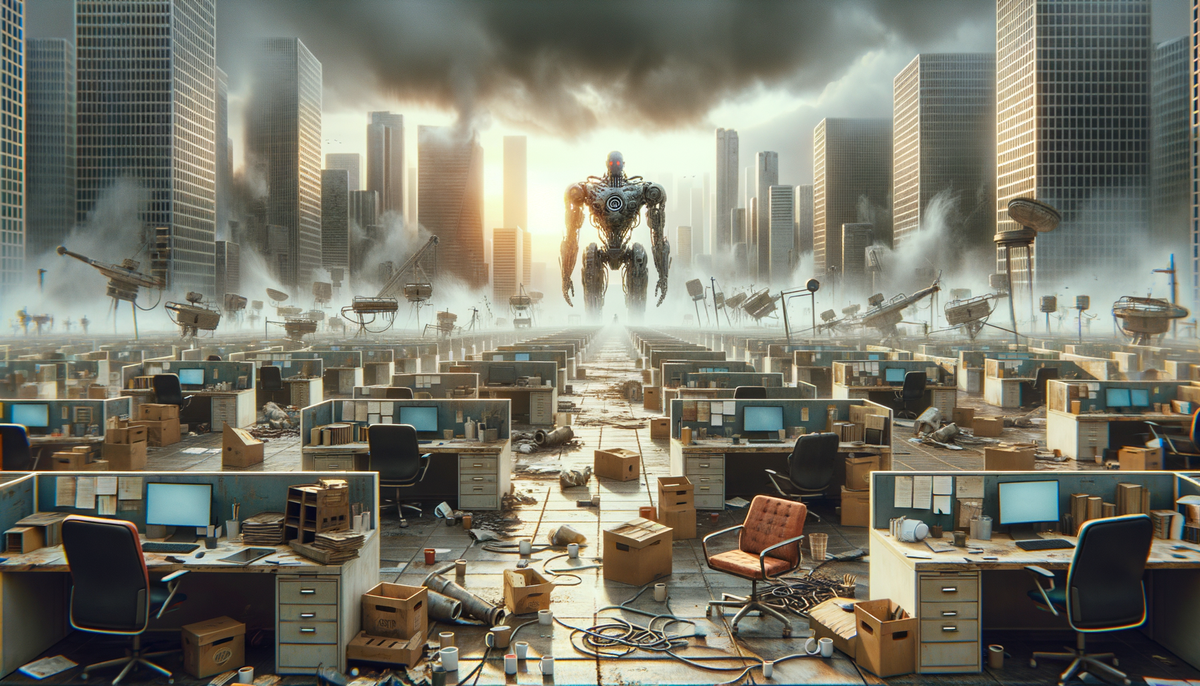
In an era where human resources are one of the most valuable assets of a company, predicting employee attrition has become crucial to business success. Employee turnover not only disrupts the workflow but also incurs significant costs associated with hiring and training new talent. With the advent of Artificial Intelligence (AI), organizations are now equipped with powerful Tools that help predict attrition trends more accurately and take Preventive Measures in advance. In this blog post, we will delve into how AI is transforming the landscape of workforce management by predicting employee attrition.
Understanding Employee Attrition
Employee attrition refers to the gradual reduction of a company's workforce due to various factors, such as resignation, retirement, or termination. Attrition can be voluntary, where employees choose to leave, or involuntary, where employees are let go by the employer. High attrition rates can lead to disruptions, increased costs, lower morale, and hindered productivity. Addressing the root cause of attrition is vital, and this is where AI steps in.
The Role of AI in Predicting Employee Attrition
AI, with its advanced machine learning and data analytics capabilities, is revolutionizing how companies approach employee attrition. By analyzing patterns and trends in employee behavior, AI can predict which employees may be at risk of leaving and identify the factors contributing to their decision. This predictive capability allows organizations to implement strategies to retain valuable employees proactively.
data Collection and analysis
The foundation of AI-based attrition prediction lies in collecting and analyzing vast amounts of employee data. Companies leverage a wide array of data sources, such as HR records, employee engagement surveys, performance metrics, and even social media activity, to get a comprehensive understanding of the workforce. AI algorithms process this data to identify patterns that signify an employee’s likelihood of leaving.
For instance, frequent short-lived engagements in different projects may indicate job dissatisfaction, while prolonged absence records might highlight personal issues affecting professional commitment. AI models can sift through these complexities efficiently and present insights that are often overlooked by traditional analytical methods.
Key Predictive Indicators
Several key indicators are often analyzed by AI to predict potential employee attrition:
-
Job Satisfaction: Lower job satisfaction is a strong predictor of attrition. AI models analyze employee feedback, surveys, and even sentiment analysis from emails or communication platforms to gauge satisfaction levels.
-
Engagement Levels: Employees who are actively engaged and contribute beyond their core responsibilities are typically less likely to leave. AI tracks engagement by analyzing participation in company events, interactions with team members, and voluntary project involvement.
-
Career Development opportunities: Employees seeking career growth may leave if they perceive limited opportunities. AI can detect patterns of upskilling and reskilling activities, interest in training programs, and internal promotion statistics.
-
work-life balance: Imbalance between personal and professional life is a common reason for attrition. AI evaluates metrics like overtime hours, frequency of remote work requests, and leave utilization to predict burnouts.
-
Compensation and Benefits: Competitive compensation packages are crucial in retaining talent. AI can benchmark compensation levels with market standards and identify discrepancies that may lead to attrition.
machine learning Models
AI employs various machine learning models to predict attrition. Some popular models include:
-
Decision Trees: These models split the data into branches to predict outcomes. They are intuitive and handle both categorical and numerical data.
-
Random Forests: An ensemble of decision trees that improves accuracy by reducing variance and avoiding overfitting.
-
Logistic Regression: A statistical model that predicts the probability of an event occurring, such as an employee leaving.
-
Neural Networks: These models mimic the human brain's neural paths, making them effective for complex pattern recognition.
Each model has its intricacies and needs to be selected based on the organization's unique data characteristics.
Benefits of AI-Driven Attrition prediction
-
cost savings: Reducing attrition minimizes hiring and training costs. AI-driven insights allow companies to focus on retaining high-performing employees, which, in turn, optimizes resource allocation.
-
Enhanced decision making: AI provides HR teams and managers with data-driven insights that improve decision-making regarding employee retention strategies and policy formulation.
-
Proactive Measures: predictive analytics enable companies to take proactive measures rather than reactive responses, potentially preventing valuable employees from leaving by addressing the issues beforehand.
-
Tailored Retention Strategies: With AI's precise insights, companies can create personalized retention strategies that align with individual employee motivations and needs.
Implementing AI Attrition Models
Implementing AI models to predict attrition involves several steps:
-
data Preparation: Collect and preprocess relevant data. IT's crucial to ensure data accuracy and completeness to build reliable models.
-
Model Selection: Choose the right machine learning models based on data characteristics and business requirements.
-
training and Testing: Train the models with historical data, followed by testing to evaluate the model's predictive accuracy. Fine-tune the model parameters for optimal performance.
-
integration: Integrate AI insights into HR systems and processes. Ensure seamless Collaboration between data scientists, HR professionals, and managers.
-
continuous monitoring: Regularly update and monitor the model's performance. As workforce dynamics change, recalibrating the models will ensure sustained accuracy.
Real-World Impact
Several successful implementations of AI in predicting employee attrition highlight its transformative potential. Companies such as IBM and Google have embraced AI for workforce analytics, significantly reducing their attrition rates. IBM's Watson Analytics has reportedly helped save millions by offering predictive analytics solutions that identify at-risk employees and suggest interventions.
challenges and Ethical Considerations
Despite its advantages, AI-based attrition prediction poses challenges, including data privacy concerns and algorithmic bias. IT's imperative for organizations to handle employee data ethically, ensuring transparency and consent in data collection. Additionally, AI models must be continually monitored to prevent biases that could lead to unfair decision-making.
Conclusion
The ability to predict employee attrition through AI is a game-changer for modern organizations. By unlocking insights buried within data, AI empowers companies to craft effective retention strategies, enhance workplace satisfaction, and ultimately secure their competitive edge. As AI continues to evolve, its integration in HR processes will undoubtedly become a staple, fostering a stable and engaged workforce.