How AI is Optimizing Resource Allocation in Cloud Workloads
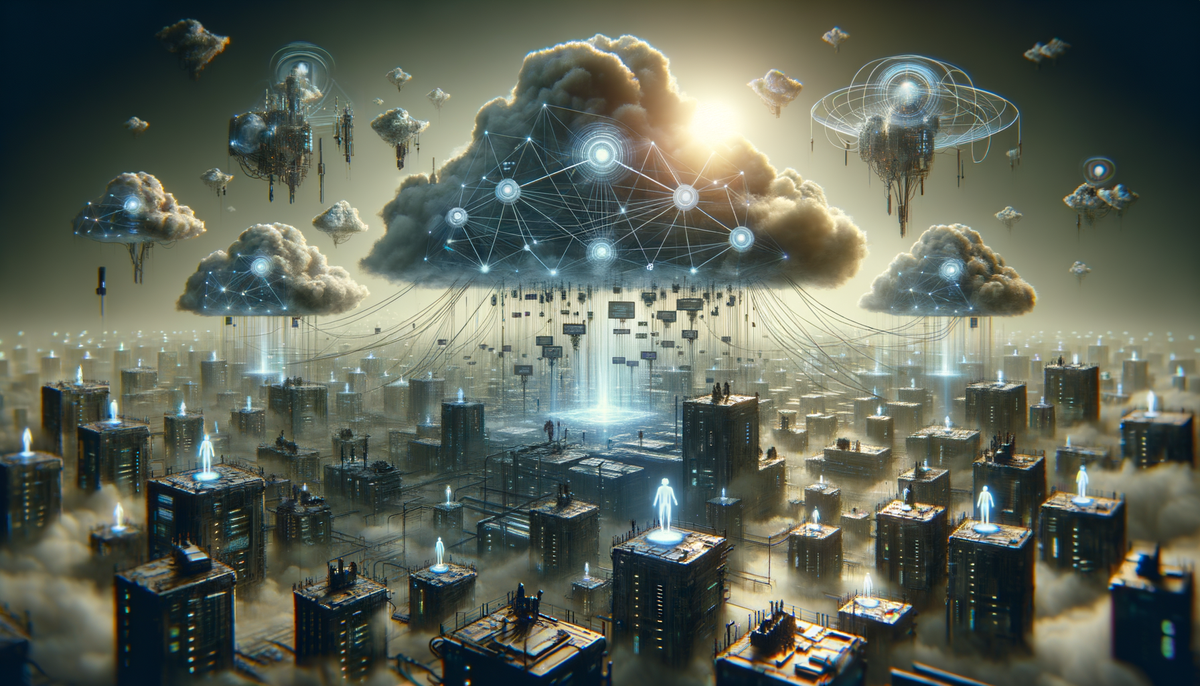
The Cloud Computing landscape has revolutionized the way businesses operate, offering unprecedented scalability, flexibility, and efficiency. However, with these advantages comes a significant challenge: optimizing resource allocation in cloud workloads. As organizations increasingly rely on cloud infrastructure to power their Digital Operations, there is a pressing need to manage cloud resources effectively to ensure Cost-Efficiency, performance stability, and operational agility. Enter Artificial Intelligence (AI), which is reshaping the way businesses optimize resource allocation in cloud workloads, making them more efficient, economical, and adaptive to changing demands.
Understanding Resource Allocation in Cloud Workloads
Resource allocation in Cloud Computing refers to the process of distributing available computing resources—such as CPU, memory, storage, and network bandwidth—among various cloud-based applications. The goal is to ensure that each application receives the right amount of resources to perform optimally without unnecessary expenditure or over-provisioning.
Traditional resource allocation methods often rely on static provisioning and manual adjustments, which can result in inefficient use of resources, increased costs, and reduced application performance. In contrast, AI-driven solutions bring a dynamic, data-driven approach to resource allocation, enabling real-time adjustments based on workload demands and predictive analytics.
The Role of AI in Cloud Resource optimization
AI in cloud computing leverages machine learning (ML) algorithms and data analytics to make intelligent decisions about resource allocation. Here’s how AI technologies are transforming this process:
1. Predictive Analytics for demand forecasting
AI models analyze historical data to predict future resource demands. These predictive analytics help in proactively scaling resources up or down in response to anticipated changes in workload demand. By forecasting resource requirements with high accuracy, businesses can avoid under-provisioning or over-provisioning, thereby optimizing their cloud infrastructure costs.
2. Real-Time Monitoring and Autonomous Resource Management
AI-driven systems continuously monitor cloud workloads in real-time, using advanced ML algorithms to analyze resource consumption patterns, application performance metrics, and user behavior data. Based on this analysis, AI systems autonomously allocate or deallocate resources, ensuring that workloads have sufficient resources while minimizing costs and avoiding outages.
3. Anomaly Detection and Fault prediction
AI enables advanced anomaly detection, helping identify irregularities or potential issues in cloud workloads such as sudden spikes in resource usage or unexpected failures. By predicting fault conditions before they occur, AI systems can trigger preemptive actions to mitigate risks, Enhancing application reliability and availability.
4. optimization Algorithms for Efficient Resource Utilization
AI employs sophisticated optimization algorithms to analyze various resource allocation strategies and select the one that maximizes efficiency. These algorithms can consider multiple factors such as cost, performance, and energy consumption to identify the optimal distribution of resources across cloud workloads.
Benefits of AI-Optimized Resource Allocation
Implementing AI in cloud resource allocation offers a multitude of benefits:
cost efficiency
By accurately predicting resource needs and optimizing resource distribution, AI minimizes wastage and reduces unnecessary cloud expenses. Organizations can maximize their return on investment by only paying for the resources they actually use.
enhanced performance
AI ensures that applications run smoothly by adjusting resources to meet real-time demands. This results in improved application performance and user experience, as workloads always have the necessary resources to operate optimally.
Scalability and Flexibility
AI enables automatic scaling of resources, allowing businesses to adapt quickly to changing workload demands. This scalability ensures that organizations can handle traffic spikes without manual intervention, maintaining high levels of service availability and responsiveness.
Improved Security and compliance
AI's real-time monitoring capabilities enhance security by identifying and responding to potential threats or vulnerabilities promptly. Moreover, AI can assist in ensuring compliance with regulatory requirements by maintaining consistent security and data protection measures.
AI technologies Reshaping Resource Allocation
Several AI technologies are at the forefront of transforming resource allocation in Cloud Computing:
Machine Learning and Deep Learning
Machine learning (ML) algorithms analyze vast datasets to identify patterns and make informed predictions, while deep learning models, a subset of ML, utilize Neural Networks to process complex and high-dimensional data, offering even greater predictive accuracy.
Reinforcement Learning
Reinforcement Learning algorithms enable AI systems to learn adaptively by interacting with the cloud Environment and receiving feedback through rewards or penalties. This approach allows AI to autonomously improve its resource allocation strategies over time based on past experiences.
Natural Language Processing
While less directly related to resource allocation, natural language processing (NLP) can enhance Cloud Management interfaces by enabling users to interact with cloud services using natural language commands, streamlining management tasks and improving user experience.
case studies: AI in Action
Several organizations are already leveraging AI for efficient resource allocation in their cloud environments:
Netflix
Netflix utilizes AI algorithms to optimize the distribution of its vast array of content across its global infrastructure. By predicting viewer demand patterns, Netflix ensures that its content is cached and delivered efficiently, reducing latency and maintaining a seamless streaming experience for its users.
Google Cloud
Google Cloud’s AI-driven solutions assist clients in optimizing their cloud workloads through intelligent Resource Management. Their automation Tools leverage ML to predict usage patterns and adjust resources dynamically, enabling customers to achieve cost-effective scalability and enhanced performance.
AWS (Amazon Web Services)
AWS incorporates AI into its management Tools to provide users with recommendations on the most efficient resource allocation setups. With AI-powered anomaly detection and automation features, AWS helps users maintain optimal cloud performance and security compliance.
challenges and Future Directions
While AI in cloud resource allocation presents significant advantages, IT also poses certain challenges:
data privacy and security
The reliance on large datasets to train and deploy AI models raises concerns about data privacy and security. Ensuring that AI systems operate within stringent data protection guidelines is paramount to maintaining trust and compliance.
Model accountability and transparency
AI models can sometimes act as black boxes, making IT difficult to understand or justify their decision-making processes. Enhancing model transparency and interpretability is crucial for aligning AI-driven decisions with organizational goals.
Resource Interdependencies
Managing interdependencies between diverse resources and applications remains a complex challenge for AI systems. Continuous advancements in AI algorithms are necessary to address these intricate interdependencies effectively.
Developing AI-Ready Cloud Infrastructures
To fully benefit from AI-driven resource allocation, organizations must ensure their cloud infrastructures are AI-ready, with compatible systems and robust data management practices.
The Future of AI-Optimized Resource Allocation
The future of AI in cloud resource allocation is promising. As AI technologies evolve, we can expect even more precise Predictive Capabilities, refined automation processes, and greater user empowerment. By continuously learning and adapting, AI will further enhance cloud resource management, driving Innovation, efficiency, and resilience in business operations.
In conclusion, AI is a game-changer in optimizing resource allocation for cloud workloads. By employing AI-driven Techniques, businesses can achieve cost savings, improved performance, and unmatched scalability, ensuring they remain competitive in the digital age. As AI continues to evolve and mature, its role in cloud resource optimization will only grow, offering exciting possibilities for transforming the Cloud Computing landscape.