Machine Learning for Predictive Analytics in Engineering Failures
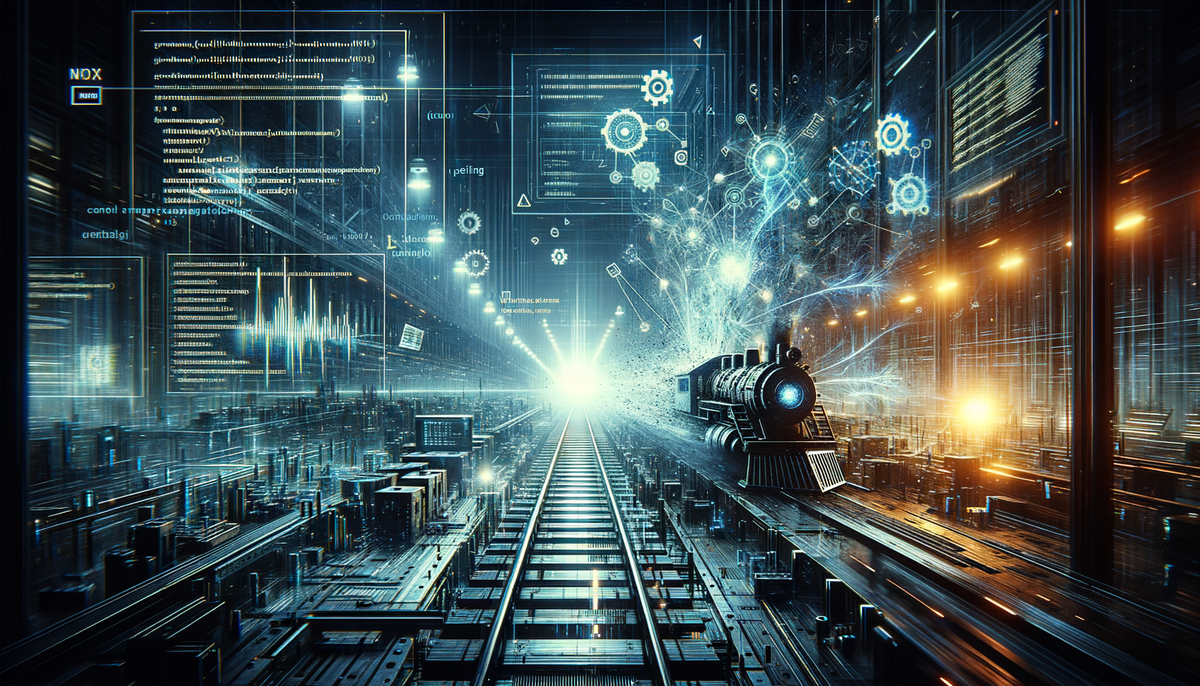
In recent years, machine learning has emerged as a potent tool in various industries for predictive analysis. Today, let's delve into how machine learning brings transformational change in identifying and preventing Engineering failures ahead of time.
The Power of Machine Learning in Predictive Analytics
Machine learning is a dynamic technology that facilitates computers to learn from prior data and predict future outcomes without human intervention. IT is instrumental in Enhancing the prediction accuracy of potential Engineering failures.
With machine learning, engineers can evaluate a large amount of data, identify patterns, and make accurate predictions. Machine learning, combined with predictive analysis, can anticipate failures based on historical data and warning signs.
Preventing failures with Predictive Analytics
Predictive analytics leverages machine learning algorithms to foresee outcomes within collected datasets. In the Engineering domain, IT is frequently used to detect and prevent probable issues before they arise. Predictive analytics can help detect minute anomalies or inefficiencies that could spell disaster if left unchecked.
Machine Learning Models for Engineering Failures
There are different machine learning models utilized for predicting Engineering failures. Some of these include:
-
Supervised Learning: This model learns from past data on which outcomes are known. IT then applies what IT has learnt to new data. IT is beneficial in manufacturing operations to identify defects in products and rectify them.
-
Unsupervised Learning: This model identifies hidden patterns or intrinsic structures in input data. IT is often used for anomaly detection in Industrial IoT, identifying subtle equipment patterns that might predict future breakdowns.
-
Reinforcement Learning: The model learns incrementally in real-time, constantly improving its recommendations based on feedback. IT is used to optimize operations, such as energy usage in smart grids.
case studies
Several industries have started leveraging machine learning for predictive analysis to mitigate Engineering failures. Some of them are:
-
Automotive: Companies like Tesla use machine learning to predict potential failures in their Electric Vehicles and proactively initiate Preventive Measures.
-
Aerospace: SpaceX uses machine learning algorithms to predict failures in rocket launches and take corrective measures before the event.
-
manufacturing: Manufacturers like Siemens use machine learning to predict failures in their production equipment and systems, ensuring optimum operational efficiency.
In conclusion, machine learning in predictive analysis provides a plethora of benefits in preventing Engineering failures. IT helps improve operational efficiency, safety, and reduces downtime, thus resulting in substantial cost savings. Given its potential, IT is no surprise that machine learning and predictive analytics are set to redefine the future of Engineering.