How AI is Shaping the Future Trends in Software Engineering
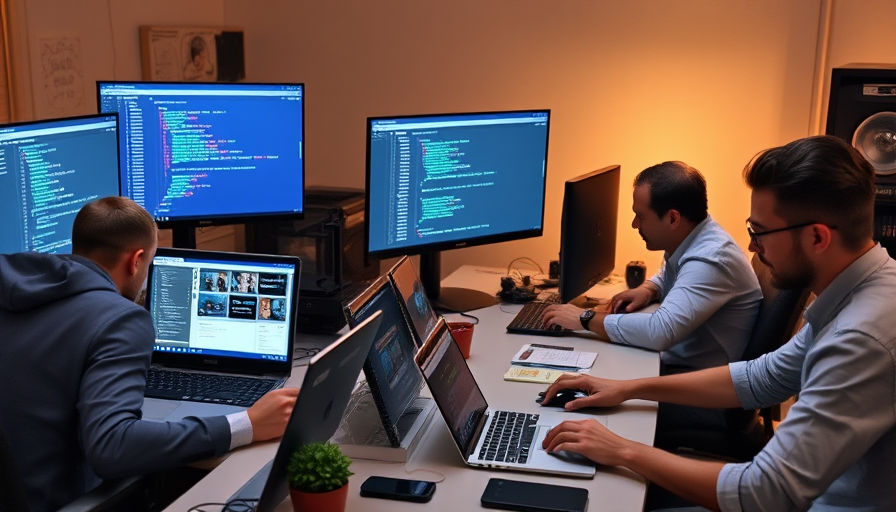
The integration of Artificial Intelligence (AI) into software Engineering has revolutionized the way developers approach problem-solving, Innovation, and efficiency. As technology continues to evolve at an unprecedented pace, understanding how AI influences future trends in software Engineering becomes crucial for staying ahead in this dynamic and competitive field.
The Impact of AI on software development
Artificial Intelligence is fundamentally altering the landscape of software development by automating repetitive tasks, Enhancing code quality, and accelerating project timelines. By leveraging machine learning algorithms, developers can predict potential issues early in the development cycle, ensuring a smoother and more efficient process.
automation in Coding
One of the most significant trends in AI-driven software Engineering is automation. Tools powered by AI can generate code snippets, identify bugs, and suggest optimizations. This not only reduces the workload on developers but also minimizes human error. For instance, Tools like GitHub Copilot use machine learning models to assist in writing code, making IT faster and more accurate.
code generation
AI-powered code generation Tools analyze patterns in existing codebases to generate new code snippets. These Tools can suggest entire functions or classes based on the context provided by the developer. For example, if a developer is working on a Python project and starts typing a function to sort a list, an AI tool might suggest using the built-in sorted()
function or provide an implementation of a custom sorting algorithm.
Bug Detection
AI can also play a crucial role in identifying bugs and vulnerabilities in code. Tools like DeepCode use machine learning algorithms to analyze code for potential issues, such as security vulnerabilities, performance bottlenecks, and logical errors. By catching these problems early in the development process, developers can save time and effort that would otherwise be spent on debugging and fixing issues later.
Code optimization
AI-driven optimization Tools can suggest improvements to existing code, making IT more efficient and performant. For example, a tool might analyze a loop and suggest using a more efficient algorithm or data structure. These optimizations can lead to significant performance gains, especially in resource-intensive applications like games or scientific Simulations.
Enhanced Code Quality
AI systems are capable of analyzing vast amounts of data to identify patterns and potential vulnerabilities in software. This capability allows for proactive measures against security breaches and performance bottlenecks. Additionally, AI-driven testing Tools can simulate various scenarios to ensure that the software behaves as expected under different conditions, thereby improving overall code quality.
Static Code analysis
Static code analysis Tools use AI to analyze code without executing IT. These Tools can identify potential issues like null pointer exceptions, resource leaks, and dead code. By catching these problems early, developers can improve code quality and reduce the likelihood of bugs in production.
Dynamic Code analysis
Dynamic code analysis involves analyzing code while IT is executing. AI-driven dynamic analysis Tools can detect issues that are difficult to find with static analysis alone, such as race conditions and memory leaks. These Tools can provide insights into how the software behaves in real-world scenarios, helping developers identify and fix performance and stability issues.
Continuous Integration and Continuous Deployment (CI/CD)
AI is also playing a significant role in CI/CD pipelines. AI-driven Tools can automatically test and deploy code changes, ensuring that new features and fixes are integrated smoothly into the existing codebase. These Tools can also provide feedback on the quality of code changes, helping developers identify and address potential issues before they reach production.
Predictive Maintenance
Predictive maintenance is another area where AI shines. By analyzing historical data, AI models can predict when software systems are likely to fail or require updates. This predictive capability allows for timely interventions, reducing downtime and ensuring that applications remain robust and reliable.
anomaly detection
AI-powered anomaly detection Tools can identify unusual patterns in software behavior that may indicate potential issues. For example, a tool might detect a sudden increase in CPU usage or memory consumption, which could signal a performance problem or security breach.
predictive analytics
predictive analytics Tools use machine learning algorithms to forecast future trends and behaviors based on historical data. In the context of software Engineering, these Tools can predict when a system is likely to experience high load or require maintenance, allowing developers to proactively address potential issues before they impact users.
future trends in Software Engineering
As we look towards the future, several trends driven by AI stand out:
Increased Use of machine learning
machine learning is set to become even more integral to software Engineering. Developers will increasingly rely on ML algorithms to create intelligent systems capable of adapting and improving over time. This trend will lead to the development of smarter applications that can handle complex tasks with minimal human intervention.
Adaptive Systems
Adaptive systems use machine learning to learn from user behavior and adjust their functionality accordingly. For example, a recommendation engine might learn from a user's browsing history and purchase patterns to provide more relevant suggestions over time.
Personalized User Experiences
AI-driven personalization allows applications to tailor their functionality and content to individual users. For instance, a streaming service might use machine learning algorithms to analyze a user's viewing habits and recommend shows and movies that align with their preferences.
AI-Powered Development Tools
The rise of AI-powered development Tools will continue, providing developers with more intuitive and efficient ways to build software. These Tools will offer features like automated code reviews, smart code completion, and real-time debugging assistance, making the development process faster and more accurate.
Automated code reviews
AI-driven code review Tools can analyze code changes and provide feedback on potential issues, such as style violations, security vulnerabilities, and performance bottlenecks. These Tools can help developers maintain high code quality standards and catch problems early in the development process.
Smart Code Completion
Smart code completion Tools use AI to suggest code snippets based on the context provided by the developer. For example, if a developer is working on a Java project and starts typing a method call, a smart code completion tool might suggest the correct method signature or provide a list of available methods.
Real-Time Debugging Assistance
AI-powered debugging Tools can help developers identify and fix issues in real-time. These Tools can analyze stack traces, log files, and other diagnostic data to provide insights into the root cause of problems, helping developers resolve issues more quickly and efficiently.
Focus on Ethical AI
As AI becomes more pervasive in software Engineering, there is a growing emphasis on ethical considerations. Ensuring that AI systems are fair, transparent, and unbiased will be a critical focus area. Developers will need to incorporate ethical guidelines into their workflows to build trustworthy and responsible AI-driven solutions.
Bias Detection
AI systems can inadvertently perpetuate or amplify biases present in the data they are trained on. Tools that detect and mitigate bias in AI models are essential for building fair and equitable systems. For example, a tool might analyze a dataset used to train a machine learning model and identify patterns of discrimination based on race, gender, or other protected characteristics.
transparency and Explainability
transparency and explainability are crucial for building trust in AI systems. Developers should strive to create AI models that are transparent in their decision-making processes and can provide clear explanations for their outputs. This is particularly important in fields like healthcare and finance, where the stakes are high, and users need to understand how AI-driven decisions are made.
Privacy-Preserving AI
privacy-preserving AI Techniques aim to protect user data while still allowing for effective machine learning. For example, differential privacy adds noise to data to prevent individual records from being identified while preserving the overall statistical properties of the dataset. This technique can be used to train machine learning models on sensitive data without compromising user privacy.
integration with IoT
The Internet of Things (IoT) is another field where AI's impact will be profound. AI-powered software can analyze data from IoT devices in real-time, enabling smarter and more responsive applications. This integration will lead to the development of innovative solutions in healthcare, smart homes, and industrial automation.
Real-Time Data Analysis
AI can process and analyze data from IoT devices in real-time, allowing for immediate insights and actions. For example, a smart home system might use AI to monitor energy consumption and adjust heating and cooling systems to optimize efficiency and comfort.
Predictive Maintenance for IoT Devices
Predictive maintenance Techniques can be applied to IoT devices to anticipate and prevent failures before they occur. AI models can analyze sensor data from IoT devices to detect anomalies that may indicate impending issues, allowing for timely maintenance and reducing downtime.
Continued Innovation
The fusion of AI with traditional software Engineering practices is driving continuous Innovation. Developers are exploring new ways to leverage AI for tasks such as natural language processing, computer vision, and robotics. These advancements promise to create groundbreaking applications that were previously unimaginable.
Natural Language Processing (NLP)
Natural Language Processing (NLP) enables machines to understand and generate human language. AI-driven NLP Tools can be used to build chatbots, virtual assistants, and other applications that interact with users in natural language. For example, a customer service chatbot might use NLP to understand user queries and provide accurate and helpful responses.
Computer Vision
Computer vision involves training machines to interpret and make decisions based on visual input from the world. AI-powered computer vision Tools can be used for tasks like object recognition, facial recognition, and autonomous driving. For example, a self-driving car might use computer vision to detect and avoid obstacles in its path.
Robotics
AI is playing a significant role in advancing robotics, enabling robots to perform complex tasks with greater Precision and efficiency. AI-driven robots can be used in manufacturing, healthcare, and other industries to automate repetitive tasks, improve safety, and increase productivity. For example, a surgical robot might use AI to assist surgeons in performing complex procedures with greater accuracy and Precision.
case studies: Real-World applications of AI in Software Engineering
healthcare
In the healthcare industry, AI is being used to develop innovative solutions that improve patient outcomes and streamline operations. For example, AI-powered diagnostic Tools can analyze medical images and other data to detect diseases like cancer at early stages, enabling timely treatment and improving survival rates.
early disease detection
AI algorithms can analyze medical images, such as X-rays and MRIs, to detect anomalies that may indicate the presence of disease. For instance, an AI system might identify patterns in a mammogram that suggest the presence of breast cancer, allowing for Early Diagnosis and treatment.
Personalized Treatment Plans
AI can also be used to create personalized treatment plans based on a patient's genetic information, medical history, and other factors. For example, a machine learning model might analyze a patient's genome to identify mutations that are responsive to specific drugs, enabling more effective and targeted treatments.
Finance
In the finance sector, AI is transforming how institutions manage risk, detect fraud, and provide personalized services to customers. AI-powered Tools can analyze vast amounts of financial data to identify trends, predict market movements, and make informed investment decisions.
Fraud Detection
AI systems can detect unusual patterns in transaction data that may indicate fraudulent activity. For example, a bank might use an AI model to monitor credit card transactions and flag those that deviate from a customer's normal spending behavior, allowing for timely intervention and prevention of financial loss.
Algorithmic Trading
Algorithmic trading involves using AI to make rapid and informed investment decisions based on market data. machine learning algorithms can analyze historical price movements, news sentiment, and other factors to predict future trends and execute trades automatically.
retail
In the retail industry, AI is Enhancing customer experiences, optimizing supply chains, and driving sales growth. AI-powered recommendation engines can analyze customer behavior and preferences to provide personalized product suggestions, increasing the likelihood of purchase.
personalized recommendations
AI-driven recommendation systems can analyze a customer's browsing and purchase history to suggest products that align with their interests. For example, an e-commerce platform might use machine learning algorithms to recommend books, movies, or other items based on a customer's past purchases and ratings.
inventory management
AI can optimize inventory management by predicting demand for products and adjusting stock levels accordingly. For instance, a retailer might use AI models to analyze sales data and external factors like weather patterns to forecast demand for seasonal items, ensuring adequate supply and minimizing waste.
manufacturing
In manufacturing, AI is automating processes, improving quality control, and increasing efficiency. AI-powered robots can perform repetitive tasks with Precision and consistency, reducing the need for human intervention and minimizing errors.
quality control
AI systems can inspect products for defects and ensure they meet quality standards. For example, a camera equipped with computer vision technology might analyze images of manufactured parts to detect flaws like cracks or misalignments, allowing for timely corrections and improvements in product quality.
Predictive Maintenance
Predictive maintenance Techniques can be applied to manufacturing equipment to anticipate and prevent failures before they occur. AI models can analyze sensor data from machinery to detect anomalies that may indicate impending issues, enabling timely maintenance and reducing downtime.
AI is undeniably shaping the future trends in software Engineering. From automating coding tasks to Enhancing code quality and predictive maintenance, AI's influence is transforming how we approach development. As the technology continues to advance, we can expect even more exciting innovations and breakthroughs that will redefine what’s possible in software Engineering.
By embracing these advancements and incorporating ethical considerations into their practices, developers can build robust, efficient, and responsible AI-driven solutions that meet the needs of users and drive Progress in various industries. The future of software Engineering is bright, and AI is at the forefront of this exciting journey.