AI in Predictive Healthcare Analytics: Transforming the Future of Medicine
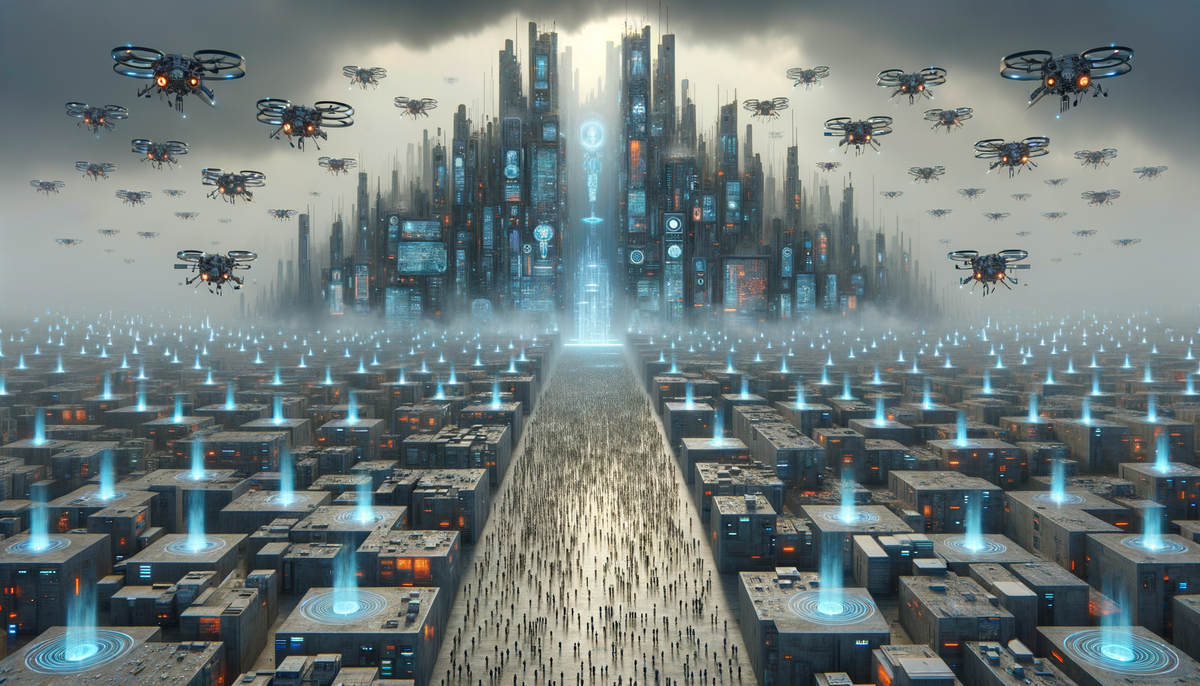
The healthcare industry is currently undergoing a transformational shift, powered by Artificial Intelligence (AI) in predictive analytics. By harnessing the vast amounts of data generated in healthcare settings, AI is set to revolutionize how we understand, diagnose, and treat diseases. This blog post delves into how AI is impacting predictive healthcare analytics, its benefits, challenges, and future prospects.
Understanding Predictive healthcare Analytics
Predictive healthcare analytics employs data mining, predictive modeling, and machine learning Techniques to predict future health outcomes. At its core, predictive analytics leverages historical data to forecast potential health events, enabling healthcare providers to take preemptive measures.
By integrating AI, predictive analytics systems become more sophisticated, capable of processing massive datasets in real-time to identify patterns and correlations that might be invisible to human experts.
The Role of AI in predictive analytics
1. Enhanced data Processing
healthcare generates an enormous amount of data from multiple sources including electronic health records (EHRs), Medical Imaging, genomic data, and wearable devices. AI algorithms can efficiently process these diverse data sets, extracting meaningful insights that drive predictive analytics.
2. Improved accuracy
AI systems are designed to learn and adapt. Over time, they improve their predictive accuracy by learning from new data. This is particularly useful in healthcare where medical insights are crucial for life-saving decisions. For instance, AI can predict patient deterioration in intensive care units (ICUs) with greater Precision.
3. Personalized Medicine
AI-powered predictive analytics plays a significant role in personalized medicine by identifying individual risk profiles and tailoring treatment plans accordingly. Through the analysis of genetic, environmental, and lifestyle data, AI systems can forecast disease susceptibility and treatment responses, allowing for customized healthcare interventions.
applications of AI in Predictive healthcare Analytics
early disease detection
AI algorithms can identify early warning signs of diseases like cancer, cardiovascular conditions, and diabetes. By analyzing EHRs and correlating symptoms with historical patterns, AI can alert providers to patients at risk, significantly improving survival rates.
Hospital Readmission Reduction
predictive analytics can forecast patients who are at risk of readmission after discharge. By identifying these individuals, healthcare systems can implement targeted interventions to prevent readmissions, optimizing patient care and reducing costs.
Drug Discovery and Development
The pharmaceutical industry benefits from AI-driven analytics in predicting drug efficacy and safety. By simulating biological interactions and analyzing patient data, AI can streamline the drug discovery process, reducing time and expenses associated with clinical trials.
resource allocation
AI can predict patient influx and resource needs in healthcare facilities, helping in the effective allocation of staff, equipment, and beds. This ensures that hospitals are prepared to meet patient demands, improving service delivery.
Benefits of AI in Predictive healthcare Analytics
Improved Patient Outcomes
By enabling Early Diagnosis and personalized treatment plans, AI in predictive analytics enhances patient outcomes. Patients receive timely interventions tailored to their specific needs, which translates to better health and survival rates.
cost efficiency
predictive analytics allows healthcare providers to optimize resources and reduce wasteful expenditures. By predicting health events, unnecessary tests and procedures can be minimized, and resources can be allocated more efficiently, leading to significant cost savings.
Proactive healthcare Management
With predictive insights, healthcare providers can move from a reactive to a proactive stance, focusing on prevention and early intervention rather than treatment after the fact. This shift not only enhances patient care but also alleviates the burden on healthcare systems.
challenges and Considerations
data privacy and security
The integration of AI in healthcare raises concerns about data privacy and security. healthcare data is highly sensitive, and ensuring its protection against breaches is paramount. Regulatory compliance, such as with the Health Insurance Portability and accountability Act (HIPAA), is critical.
ethical concerns
AI-driven decisions must align with Ethical Standards. Ensuring transparency and explainability in AI models is crucial to avoid biases that could affect patient care. Ethical considerations in predictive analytics encompass the fair and just use of AI systems in healthcare settings.
integration with Existing Systems
Integrating AI into existing healthcare infrastructure could be challenging. Compatibility issues, cost of deployment, and the need for skilled personnel to interpret AI outputs are potential barriers to adoption.
accuracy and Trust
The reliability of AI predictions is vital. Building trust in AI systems requires proving their accuracy over time and demonstrating tangible benefits in patient outcomes and process efficiencies.
The Future of AI in Predictive healthcare Analytics
The potential for AI in predictive healthcare analytics is immense. As technology continues to evolve, we can expect AI to become more deeply integrated into healthcare operations, driving Innovation across diagnostics, treatment, and patient management.
Advances in Machine Learning and Deep Learning
Future developments in machine learning and Deep Learning will enhance the sophistication of predictive analytics models, allowing for more precise and timely predictions with comprehensive datasets.
interoperability and Collaboration
Increased interoperability between healthcare systems and technological platforms will enable seamless integration of AI-driven predictive analytics, facilitating collaborative efforts for holistic patient care.
Adoption of Wearable technology
Wearables and IoT devices will generate continuous health data, bolstering AI's capability to provide real-time health insights, predicting potential health issues and prompting timely interventions.
Emphasis on training and Education
As the adoption of AI in healthcare grows, there will be a greater need for training programs to equip healthcare professionals with the skills to interpret and apply predictive analytics in clinical practice.
Conclusion
AI in predictive healthcare analytics signifies a paradigm shift towards a data-driven, patient-centered approach. Although challenges exist, the benefits of improved patient outcomes, cost savings, and proactive healthcare far outweigh the hurdles. As technological advancements continue to unfold, AI will indisputably shape the future of healthcare, transforming IT into a more efficient and personalized experience.