Enhancing Retail Operations with AI
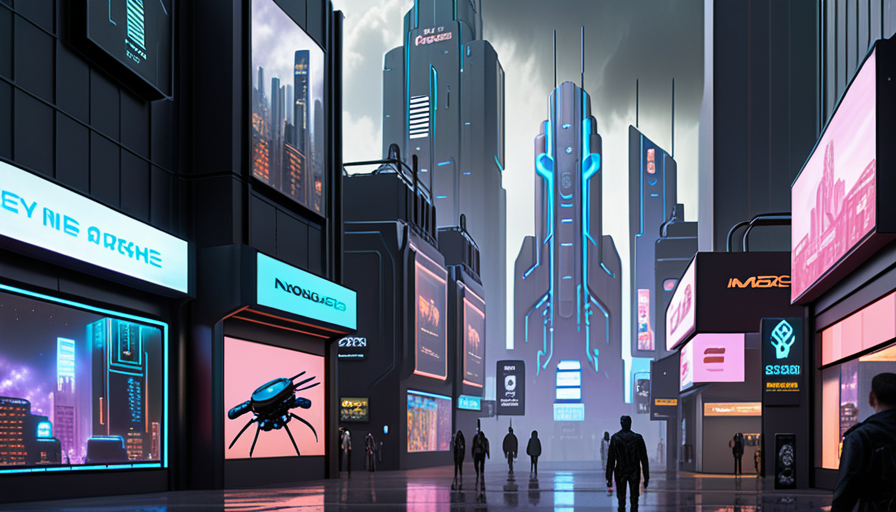
In today's rapidly evolving business landscape, Artificial Intelligence (AI) has become an indispensable tool for optimizing retail operations. By leveraging cutting-edge technologies such as machine learning, Natural Language Processing, and predictive analytics, retailers can revolutionize their business processes to meet the ever-increasing demands of modern consumers. This blog post delves into how AI can enhance various facets of retail operations, from Supply Chain management to customer experience, ultimately driving efficiency, personalization, and growth.
1. Streamlining inventory management
One of the most significant challenges faced by retailers is efficient inventory management. Overstocking leads to increased holding costs, while understocking results in lost sales opportunities. AI can address these issues through predictive analytics and demand forecasting. By analyzing historical data and identifying patterns, AI algorithms can predict future demand with remarkable accuracy.
Benefits:
- Reduced Stockouts: With precise predictions, retailers can maintain optimal stock levels to meet customer demands without overstocking.
- cost efficiency: Minimizing excess inventory reduces storage costs and frees up capital for other strategic investments.
- Automated Replenishment: AI-driven systems can automate ordering processes based on real-time data analysis.
Example:
Walmart utilizes AI to manage its vast inventory across thousands of stores, ensuring that shelves are always stocked with the right products at the right time. This capability has significantly enhanced their operational efficiency and customer satisfaction levels.
Implementation Steps:
- data Collection: Gather historical sales data, seasonal trends, and external factors like weather patterns.
- Algorithm Selection: Choose appropriate machine learning algorithms for demand forecasting (e.g., ARIMA, LSTM).
- Model training: Train the model using collected data to identify patterns and correlations.
- Real-Time analysis: Implement real-time data analysis to adjust inventory levels dynamically.
- automation: Integrate AI systems with existing inventory management software for automated replenishment.
challenges:
- data Quality: Ensuring accurate and comprehensive data collection is crucial for reliable predictions.
- Model accuracy: continuous monitoring and updating of models are necessary to maintain accuracy.
- integration: Seamless integration with existing systems can be complex and may require significant IT resources.
2. Enhancing Customer Experiences
AI-powered personalization Tools enable retailers to offer tailored shopping experiences, increasing customer satisfaction and loyalty. By analyzing customer behavior and preferences, AI can provide personalized product recommendations, targeted promotions, and customized content.
Benefits:
- increased sales: personalized recommendations drive higher conversion rates and average order values.
- Customer Loyalty: Tailored experiences foster stronger customer relationships and repeat business.
- Operational efficiency: Automated personalization reduces the need for manual intervention in marketing efforts.
Example:
Amazon’s recommendation engine uses AI to analyze customer browsing and purchase history, offering personalized product suggestions that drive significant sales.
Implementation Steps:
- Customer data Collection: Gather data on customer interactions, preferences, and behaviors.
- Segmentation: Segment customers based on demographics, purchasing patterns, and other relevant criteria.
- personalization Algorithms: Implement algorithms for real-time personalization (e.g., collaborative filtering, content-based filtering).
- Content customization: Use AI to customize website content, email campaigns, and in-store experiences.
- Feedback Loop: Continuously collect feedback and adjust personalization strategies accordingly.
challenges:
- data privacy: Ensuring customer data is handled securely and complies with privacy Regulations.
- Algorithm Bias: Avoiding biases in recommendation algorithms that could lead to unfair treatment of certain customer segments.
- Scalability: Managing large volumes of data and ensuring real-time personalization for all customers.
3. Optimizing Pricing Strategies
dynamic pricing, powered by AI, allows retailers to adjust prices in real-time based on market conditions, competitor actions, and customer demand. This strategy helps maximize revenue and stay competitive in a dynamic marketplace.
Benefits:
- Revenue Maximization: dynamic pricing ensures that products are priced optimally to capture the highest possible revenue.
- Competitive Advantage: Real-time price adjustments help retailers stay ahead of competitors.
- Customer Perception: Offering competitive prices can enhance customer satisfaction and attract price-sensitive shoppers.
Example:
Uber uses AI-driven dynamic pricing to adjust fares based on demand, ensuring optimal utilization of its fleet and maximizing revenue during peak times.
Implementation Steps:
- data Collection: Gather data on market trends, competitor pricing, and customer behavior.
- Pricing Algorithms: Develop algorithms for real-time price adjustments (e.g., machine learning models, rule-based systems).
- integration: Integrate dynamic pricing systems with existing e-commerce platforms and POS systems.
- Monitoring: Continuously monitor market conditions and adjust pricing strategies accordingly.
- Customer Communication: Clearly communicate pricing changes to customers to maintain transparency.
challenges:
- Market Volatility: Rapidly changing market conditions can make IT challenging to set optimal prices.
- Customer Perception: Frequent price changes may lead to customer dissatisfaction if not managed properly.
- Technical Complexity: Implementing dynamic pricing systems requires advanced technical expertise and robust infrastructure.
4. Improving Supply Chain Processes
AI can optimize Supply Chain operations by predicting demand, optimizing routes, and managing inventory levels across multiple locations. This results in reduced costs, improved efficiency, and enhanced customer satisfaction.
Benefits:
- cost reduction: Optimized logistics and inventory management lead to significant cost savings.
- efficiency: Streamlined processes reduce delays and improve overall Supply Chain performance.
- customer satisfaction: Reliable delivery times and accurate order fulfillment enhance customer trust.
Example:
DHL uses AI to optimize its logistics operations, predicting demand spikes and optimizing delivery routes to ensure timely deliveries.
Implementation Steps:
- data integration: Integrate data from various sources, including suppliers, warehouses, and distribution centers.
- demand forecasting: Use AI for accurate demand forecasting to plan inventory levels and production schedules.
- Route optimization: Implement algorithms for optimizing delivery routes (e.g., VRP - Vehicle Routing Problem).
- inventory management: Use AI-driven systems for real-time inventory tracking and automated replenishment.
- performance monitoring: Continuously monitor Supply Chain performance and make data-driven adjustments.
challenges:
- data Silos: Breaking down data silos to ensure seamless integration across the Supply Chain.
- Complexity: Managing complex logistics networks with multiple variables can be challenging.
- Scalability: Ensuring that AI systems can scale with business growth and changing market conditions.
5. Upgrading customer service
AI-powered chatbots and virtual assistants provide round-the-clock customer support, answering queries, resolving issues, and offering personalized recommendations. This enhances customer satisfaction and reduces the workload on human agents.
Benefits:
- 24/7 Support: Continuous availability of customer service improves overall customer experience.
- efficiency: Automated Responses reduce response times and handle a higher volume of inquiries.
- cost savings: Reduced need for human agents leads to significant cost savings in customer support operations.
Example:
Bank of America’s virtual assistant, Erica, uses AI to provide personalized financial advice and support to customers 24/7.
Implementation Steps:
- customer interaction data: Collect data on common customer queries and interactions.
- Natural Language Processing (NLP): Implement NLP algorithms for understanding and responding to customer inquiries.
- Chatbot Development: Develop AI-powered chatbots that can handle a wide range of customer service tasks.
- integration: Integrate chatbots with existing customer support systems, including CRM and helpdesk software.
- continuous improvement: Continuously train and update chatbots based on customer feedback and evolving needs.
challenges:
- accuracy: Ensuring that chatbots provide accurate and relevant responses to customer queries.
- customer trust: Building trust in AI-driven customer service solutions, especially for sensitive issues.
- integration: Seamless integration with existing systems can be complex and may require significant IT resources.
6. Leveraging predictive analytics
predictive analytics uses historical data and machine learning algorithms to forecast future trends, customer behavior, and market conditions. This enables retailers to make informed decisions and stay ahead of the competition.
Benefits:
- Informed decision-making: data-driven insights help in making strategic business decisions.
- risk management: Identifying potential risks and opportunities before they materialize.
- Competitive Edge: Staying ahead of market trends and customer preferences.
Example:
Walmart uses predictive analytics to forecast demand, optimize inventory levels, and plan marketing strategies based on customer behavior data.
Implementation Steps:
- data Collection: Gather historical data from various sources, including sales, customer interactions, and market trends.
- Model Development: Develop machine learning models for predicting future trends (e.g., time series analysis, regression models).
- integration: Integrate predictive analytics systems with existing Business intelligence Tools.
- Real-Time analysis: Implement real-time data analysis to provide timely insights and recommendations.
- continuous monitoring: Continuously monitor model performance and update algorithms as needed.
challenges:
- data Quality: Ensuring accurate and comprehensive data collection is crucial for reliable predictions.
- Model accuracy: continuous monitoring and updating of models are necessary to maintain accuracy.
- Technical Expertise: Requires advanced technical expertise in machine learning and data science.
7. Enhancing marketing strategies
AI-driven marketing Tools enable retailers to create targeted campaigns, optimize ad spend, and measure the effectiveness of marketing efforts. This results in higher ROI and more effective customer engagement.
Benefits:
- Targeted Campaigns: personalized marketing messages reach the right audience at the right time.
- cost efficiency: Optimizing ad spend ensures that marketing budgets are used effectively.
- Performance Measurement: Accurate measurement of campaign performance helps in making data-driven decisions.
Example:
Starbucks uses AI to analyze customer data and create personalized marketing campaigns, driving higher engagement and sales.
Implementation Steps:
- Customer data Collection: Gather data on customer interactions, preferences, and behaviors.
- Segmentation: Segment customers based on demographics, purchasing patterns, and other relevant criteria.
- Marketing Algorithms: Implement algorithms for targeted campaign creation (e.g., clustering, classification).
- Ad optimization: Use AI to optimize ad spend and placement across various channels.
- Performance Tracking: Continuously monitor campaign performance and adjust strategies accordingly.
challenges:
- data privacy: Ensuring customer data is handled securely and complies with privacy Regulations.
- Algorithm Bias: Avoiding biases in marketing algorithms that could lead to unfair treatment of certain customer segments.
- Scalability: Managing large volumes of data and ensuring real-time personalization for all customers.
AI offers numerous opportunities for retailers to enhance operations, improve customer experiences, and stay competitive. By leveraging AI-driven Tools and strategies, retailers can achieve significant cost savings, increase revenue, and build stronger customer relationships. However, implementing AI solutions requires careful planning, robust infrastructure, and continuous monitoring to ensure accuracy and effectiveness. With the right approach, AI can transform retail operations and drive long-term success.