Optimizing Logistics and Fleet Management in Transportation with AI
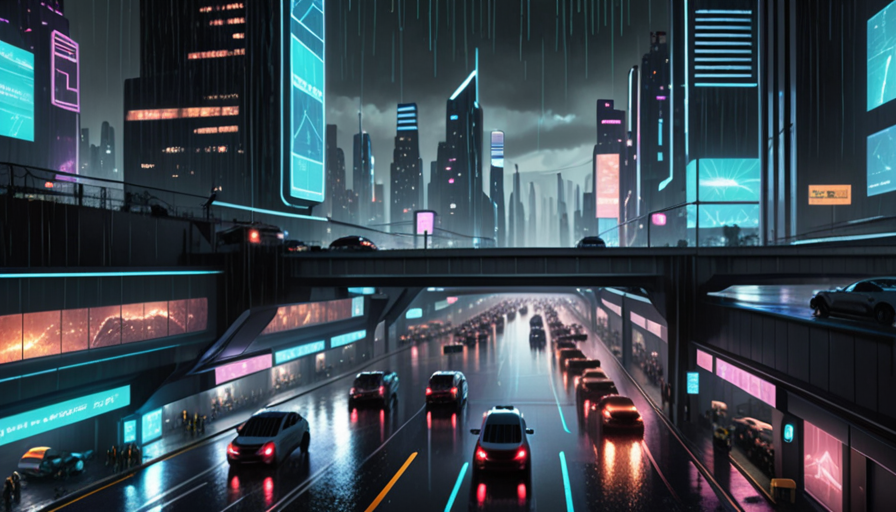
In today's fast-paced global economy, efficient logistics and fleet management are crucial for businesses looking to maintain a competitive edge. The Transportation sector is undergoing a significant transformation, driven by advancements in Artificial Intelligence (AI). By integrating AI into logistics and fleet management, companies can optimize operations, reduce costs, and improve service delivery. This comprehensive guide explores the ways AI is reshaping these critical areas, offering insights into best practices, case studies, and future trends.
Understanding Logistics and Fleet Management
What is Logistics?
Logistics involves planning, implementing, and controlling the efficient movement of goods from origin to destination. IT encompasses Transportation, warehousing, inventory management, and order fulfillment. Effective logistics ensure timely delivery, cost efficiency, and customer satisfaction. Key components of logistics include:
- Transportation Management: Coordinating the movement of goods via various modes like road, rail, air, and sea.
- Warehouse Management: Optimizing storage space, managing inventory levels, and ensuring efficient order picking and packing.
- inventory management: Tracking stock levels, forecasting demand, and maintaining optimal inventory to meet customer needs without excess holding costs.
- Order Fulfillment: Processing orders accurately and efficiently, from receipt to delivery.
What is fleet management?
fleet management refers to the administration of a company's vehicle fleet. IT includes tracking vehicle locations, managing maintenance schedules, monitoring fuel consumption, ensuring regulatory compliance, and optimizing route planning. A well-managed fleet enhances operational efficiency and reduces downtime. Key aspects of fleet management include:
- Vehicle Tracking: Using GPS and Telematics to monitor vehicle locations in real-time.
- Maintenance Management: Scheduling regular maintenance and repairs to keep vehicles in optimal condition.
- Fuel Management: Monitoring fuel consumption, identifying inefficiencies, and implementing strategies to reduce costs.
- Route Planning: Optimizing routes to minimize travel time, reduce fuel consumption, and ensure timely deliveries.
The Role of AI in Transforming Logistics
AI is revolutionizing logistics by automating processes, Enhancing decision-making, and improving accuracy. Here are some key ways AI is making an impact:
1. Predictive Analytics for Demand Forecasting
AI-driven predictive analytics helps businesses anticipate demand fluctuations with greater Precision. By analyzing historical data, market trends, and external factors like weather or economic conditions, AI models can forecast future demand patterns. This enables companies to adjust inventory levels proactively, minimizing stockouts and overstock situations.
Case Study: Amazon
Amazon uses AI-driven predictive analytics to forecast demand for millions of products. Their algorithms analyze vast amounts of data, including customer purchase history, browsing behavior, and seasonal trends, to predict which items will be in high demand. This allows Amazon to optimize inventory levels, reduce storage costs, and ensure timely delivery.
2. Automated Warehousing and Inventory Management
AI-powered robots and autonomous guided vehicles (AGVs) are transforming warehouse operations. These systems can handle tasks such as picking, packing, and sorting with speed and accuracy, reducing the need for human intervention.
Case Study: Ocado
Ocado, a UK-based online grocery retailer, uses AI-driven automation in its warehouses. Robots navigate the warehouse floor, retrieving items from storage and delivering them to packing stations. This automated system significantly increases efficiency, reduces errors, and allows Ocado to handle a high volume of orders during peak periods.
3. Intelligent Transportation Management
AI can optimize Transportation routes, reduce fuel consumption, and improve delivery times. By analyzing real-time traffic data, weather conditions, and vehicle performance, AI systems can dynamically adjust routes to avoid delays and minimize costs.
Case Study: UPS
UPS uses an AI-driven route optimization system called ORION (On-Road Integrated optimization and Navigation). ORION analyzes millions of possible routes and selects the most efficient one based on factors like traffic, weather, and delivery priorities. This has helped UPS reduce fuel consumption by millions of gallons annually and improve delivery times.
4. Enhanced customer experience
AI-driven logistics solutions enable businesses to offer personalized customer experiences. By analyzing data on consumer preferences and behaviors, companies can tailor delivery options and provide real-time updates on order status.
Case Study: DHL
DHL uses AI to enhance its customer service through predictive analytics and chatbots. Their AI systems analyze customer data to predict potential issues and proactively address them. chatbots provide 24/7 support, answering queries and resolving problems in real-time. This improves customer satisfaction and reduces the workload on human agents.
The Role of AI in Transforming fleet management
AI is also transforming fleet management by improving efficiency, reducing costs, and Enhancing safety. Here are some key ways AI is making an impact:
1. Predictive Maintenance
Predictive maintenance uses AI to monitor vehicle performance in real-time and predict when maintenance or repairs will be needed. By analyzing data from sensors and Telematics devices, AI systems can identify potential issues before they cause breakdowns.
Case Study: Daimler Trucks
Daimler Trucks uses predictive maintenance to keep its fleet of trucks running smoothly. Their AI system, called Fleetboard, monitors vehicle performance in real-time and predicts when maintenance will be required. This reduces downtime, extends the lifespan of vehicles, and improves overall fleet efficiency.
2. Fuel Management
AI can optimize fuel consumption by analyzing driving patterns, route efficiency, and vehicle performance. By identifying inefficiencies and implementing strategies to reduce fuel usage, companies can significantly lower their operating costs.
Case Study: Shell
Shell uses AI-driven fuel management solutions to help its customers reduce fuel consumption. Their system analyzes data from Telematics devices to identify inefficient driving behaviors and optimize routes. This has helped Shell's customers achieve significant fuel savings and reduce their carbon footprint.
3. Route optimization
AI can optimize Transportation routes, reducing travel time, fuel consumption, and delivery times. By analyzing real-time traffic data, weather conditions, and vehicle performance, AI systems can dynamically adjust routes to avoid delays and minimize costs.
Case Study: FedEx
FedEx uses an AI-driven route optimization system called Route optimization Engine (ROE). ROE analyzes millions of possible routes and selects the most efficient one based on factors like traffic, weather, and delivery priorities. This has helped FedEx reduce fuel consumption by millions of gallons annually and improve delivery times.
4. Driver safety
AI can enhance driver safety by monitoring driving behaviors, detecting potential hazards, and providing real-time alerts. By analyzing data from cameras, sensors, and Telematics devices, AI systems can identify risky behaviors and take corrective actions to prevent accidents.
Case Study: Lyft
Lyft uses AI-driven driver safety solutions to improve the safety of its rideshare service. Their system analyzes data from cameras and sensors to monitor driving behaviors in real-time and detect potential hazards. If a risk is identified, the system provides alerts to the driver and takes corrective actions to prevent accidents.
Implementing AI in Logistics and Fleet Management
Integrating AI into logistics and fleet management requires careful planning, strategic investment, and a commitment to continuous improvement. Here are some steps to successfully implement AI-driven solutions:
1. Assess Your Needs
Before implementing AI, IT's essential to assess your specific needs and identify areas where AI can provide the most significant benefits. Conduct a thorough analysis of your current operations, identify inefficiencies, and determine how AI can address these issues.
Example:
A logistics company might identify that route optimization is a critical area for improvement. By implementing an AI-driven route optimization system, they can reduce fuel consumption, minimize delivery times, and enhance overall efficiency.
2. Choose the Right technology
Selecting the right AI technology is crucial for successful implementation. Evaluate different AI solutions based on their features, Scalability, and compatibility with your existing systems. Consider factors like ease of integration, cost, and vendor support when making your decision.
Example:
A fleet management company might choose a predictive maintenance system that integrates seamlessly with their Telematics devices and provides real-time alerts for potential issues. This ensures minimal disruption to operations and maximizes the benefits of AI.
3. Train Your Team
training your team is essential for successful AI implementation. Ensure that your employees understand how to use the new technology, interpret its outputs, and make data-driven decisions. Provide comprehensive training programs and ongoing support to help your team adapt to the changes.
Example:
A logistics company might offer training sessions on using an AI-driven route optimization system. These sessions could cover topics like interpreting route recommendations, adjusting routes in real-time, and analyzing performance metrics.
4. Monitor and Optimize
continuous monitoring and optimization are crucial for maximizing the benefits of AI. Regularly review the performance of your AI systems, identify areas for improvement, and make necessary adjustments to enhance efficiency and effectiveness.
Example:
A fleet management company might monitor the performance of its predictive maintenance system by tracking key metrics like downtime, repair costs, and vehicle lifespan. By analyzing this data, they can identify trends, optimize maintenance schedules, and improve overall fleet efficiency.
challenges and Considerations
While AI offers numerous benefits for logistics and fleet management, there are also challenges and considerations to keep in mind:
1. data Quality
The effectiveness of AI systems depends on the quality and accuracy of the data they process. Ensuring high-quality data is essential for reliable AI outputs and informed decision-making.
Example:
A logistics company might invest in advanced sensors and Telematics devices to collect accurate and comprehensive data on vehicle performance, route efficiency, and delivery times. This ensures that their AI systems have access to reliable data for making optimal decisions.
2. integration
Integrating AI with existing systems can be complex and time-consuming. Ensuring seamless integration is crucial for minimizing disruption to operations and maximizing the benefits of AI.
Example:
A fleet management company might work closely with vendors to ensure that their predictive maintenance system integrates seamlessly with Telematics devices, vehicle diagnostics, and other relevant systems. This ensures minimal disruption to operations and maximizes the efficiency gains from AI.
3. security
AI systems handle sensitive data, making security a critical consideration. Implementing robust security measures is essential for protecting data integrity and preventing unauthorized access.
Example:
A logistics company might invest in advanced encryption technologies, secure data storage solutions, and regular security audits to protect their AI systems from cyber threats. This ensures that sensitive data remains secure and maintains the trust of customers and partners.
4. Ethical Considerations
AI raises ethical considerations related to privacy, bias, and transparency. Ensuring that AI systems are fair, transparent, and respectful of individual rights is crucial for building trust and maintaining Ethical Standards.
Example:
A fleet management company might implement policies to ensure that their predictive maintenance system does not discriminate against certain drivers or vehicles based on biased data. They might also provide clear explanations for AI-driven decisions to enhance transparency and build trust with stakeholders.
future trends in AI for Logistics and Fleet Management
The field of AI is rapidly evolving, with new technologies and applications emerging all the time. Here are some future trends to watch out for:
1. autonomous vehicles
autonomous vehicles have the potential to revolutionize logistics and fleet management by reducing human error, improving efficiency, and Enhancing safety.
Example:
Companies like Tesla and Waymo are developing autonomous trucks that can operate without human intervention. These vehicles use advanced AI algorithms to navigate roads, avoid obstacles, and optimize routes in real-time.
2. Blockchain integration
Blockchain technology can enhance the transparency and security of logistics operations by providing a decentralized ledger for tracking goods, managing contracts, and verifying transactions.
Example:
A logistics company might integrate Blockchain with its AI-driven Supply Chain management system to provide a transparent and secure record of all transactions. This ensures that data is tamper-proof, enhances trust among stakeholders, and improves overall efficiency.
3. advanced analytics
advanced analytics can provide deeper insights into logistics and fleet operations by analyzing large datasets and identifying complex patterns and trends.
Example:
A fleet management company might use advanced analytics to analyze vehicle performance data, identify inefficiencies, and optimize maintenance schedules. This ensures that vehicles are running at peak efficiency, reduces downtime, and minimizes repair costs.
4. Edge computing
Edge computing involves processing data closer to the source, reducing latency and improving response times. This is particularly useful for real-time applications in logistics and fleet management.
Example:
A logistics company might use edge computing to process route optimization data in real-time, allowing for dynamic adjustments based on changing conditions like traffic or weather. This ensures that deliveries are made efficiently and on time.
5. AI-Driven customer service
AI-driven customer service solutions can enhance the customer experience by providing personalized support, quick responses, and accurate information.
Example:
A logistics company might implement an AI-powered chatbot to handle customer inquiries, track shipments, and provide real-time updates. This ensures that customers receive prompt and accurate information, Enhancing their overall satisfaction with the service.
AI is transforming logistics and fleet management by providing advanced Tools for optimizing operations, improving efficiency, and Enhancing safety. From predictive maintenance and route optimization to autonomous vehicles and Blockchain integration, AI offers numerous benefits for companies looking to stay competitive in a rapidly evolving market. However, implementing AI also comes with challenges related to data quality, integration, security, and ethical considerations. By addressing these challenges and staying abreast of future trends, companies can maximize the benefits of AI and achieve sustainable growth.
As AI continues to evolve, IT will undoubtedly play an even more significant role in shaping the future of logistics and fleet management. Companies that embrace this technology and adapt to its advancements will be well-positioned to thrive in a competitive landscape.
Also read: