Data Lakes vs. Data Warehouses: Key Differences and Use Cases
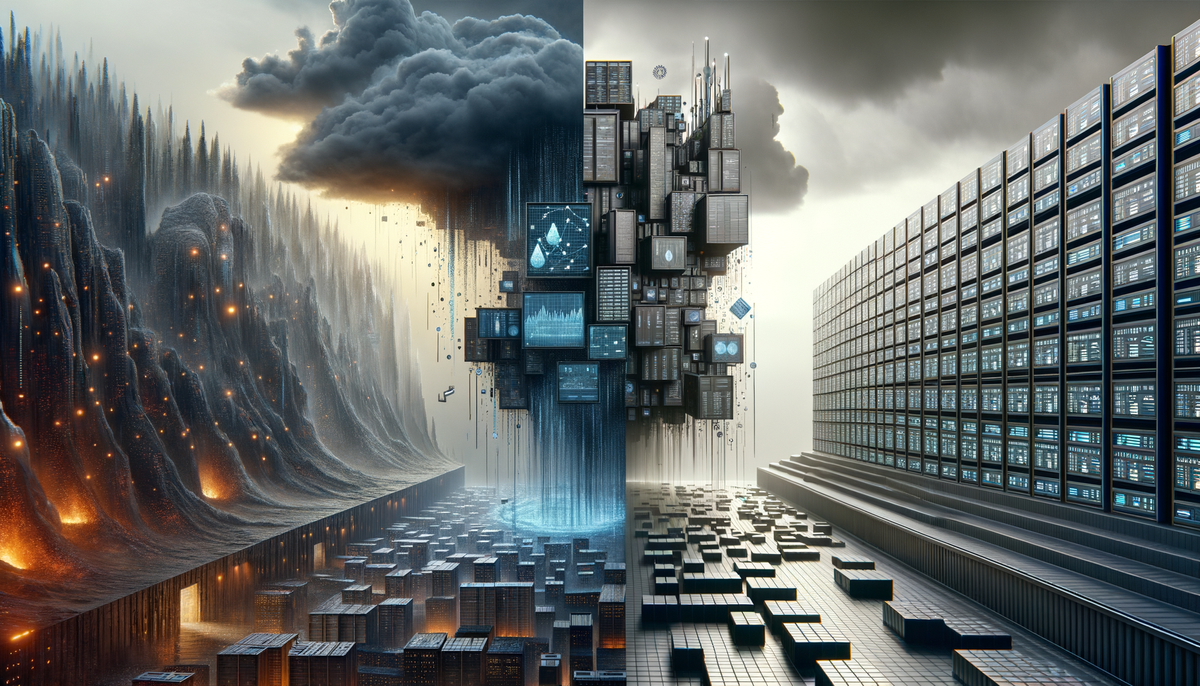
In the ever-evolving landscape of data management, organizations strive to harness the vast amounts of information at their disposal. Two primary solutions have emerged to meet these needs: data lakes and data warehouses. While both serve the overarching purpose of storing and processing data, they suit different needs and offer distinct advantages. Understanding the key differences between data lakes and data warehouses is crucial to selecting the best approach for your business.
Understanding data Lakes
Data lakes are a relatively recent Innovation in data storage. They are vast repositories that can store large volumes of raw data in its native format. data doesn't need to be pre-processed, which allows for quicker ingestion and storage. This capability makes data lakes highly scalable and flexible, ideal for collecting unstructured or semi-structured data such as images, videos, log files, sensor data, and more.
Advantages of data Lakes
- Scalability: Data lakes can handle immense volumes of data from various sources, making them extremely scalable.
- Flexibility: With the ability to store raw data, data lakes support diverse data types, aiding future analytics.
- cost-effective: Typically, data lakes use cheaper storage solutions as they don't require schema-on-write.
- machine learning and advanced analytics: Data lakes are well-suited for building machine learning models due to the availability of large datasets in their original format.
Exploring data Warehouses
Data warehouses have been around for decades and have established themselves as a cornerstone in business intelligence. They store data in a more structured format, meaning data is organized, cleaned, and transformed before loading. This transformation improves the efficiency and speed of data queries and computations, making data warehouses perfect for analytics and reporting.
Advantages of data Warehouses
- Performance: Since data is pre-processed and stored in a structured manner, querying is fast and efficient, thus supporting Real-time analytics.
- Consistency: Data warehouses ensure data consistency as IT is cleaned and transformed before storage, which reduces redundant data.
- security and compliance: With structured data storage, data warehouses can easily implement advanced security measures and compliance controls.
- business intelligence: Ideal for generating reports, dashboards, and other BI Tools, providing insights that drive decision-making.
Key Differences Between data Lakes and data Warehouses
- Storage and data Type: Data lakes support unstructured and semi-structured data, while data warehouses manage structured data.
- Processing Overhead: Data lakes require schema-on-read, meaning data is structured when accessed. In contrast, data warehouses apply schema-on-write, structuring data upon ingestion.
- Cost: Generally, IT is more cost-effective to store data in a data lake than in a data warehouse, due to lower infrastructure requirements.
- Use Cases: Data lakes suit environments with a focus on exploratory analytics and machine learning, while data warehouses are typically used for business reporting and analytics.
Use Cases for data Lakes
- Internet of Things (IoT): Collects vast amounts of streaming data from devices, sensors, etc.
- machine learning: A wealth of unstructured data provides rich grounds for training expansive models.
- research and Development: Enables data scientists to explore raw data for insights without having to wait for pre-processing.
Use Cases for data Warehouses
- Business Reporting: Generates high-performance reports and dashboards for strategic planning.
- Financial Analytics: Ensures consistent and accurate financial records by utilizing structured datasets.
- Sales and Marketing: Provides quick insight into sales trends and customer behavior, fostering data-driven strategies.
Conclusion
In today's data-driven world, deciding between a data lake and a data warehouse often depends on your organization's specific needs. Data lakes offer flexibility and Scalability, perfect for handling increasingly diverse data sources. On the other hand, data warehouses provide performance and structured query capabilities ideal for Real-time analytics and business intelligence. A hybrid approach, leveraging both systems, can offer the best of both worlds, supporting varied data initiatives and maximizing the value derived from data assets.