What Role Does AI Play in Predictive Maintenance for IT Infrastructure?
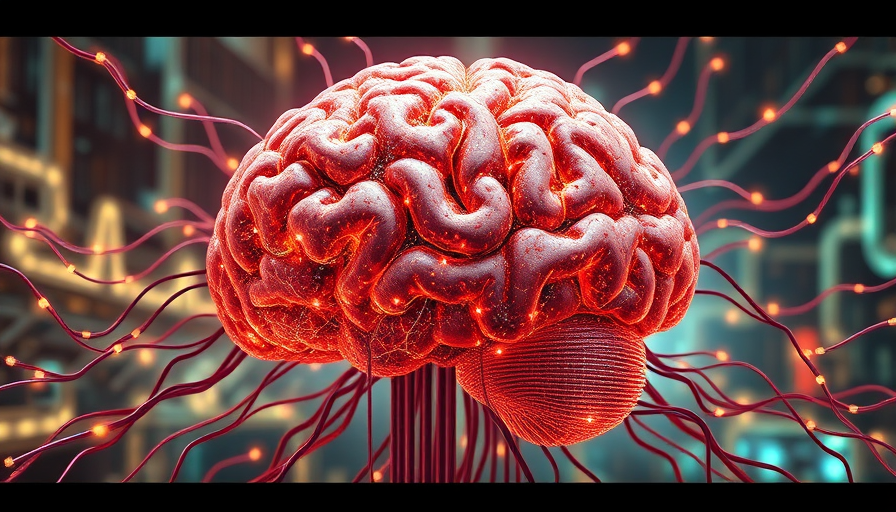
In today's rapidly evolving technological landscape, ensuring the reliability and efficiency of IT infrastructure is more crucial than ever. As organizations increasingly rely on digital systems for their core operations, any downtime can lead to significant financial losses and operational disruptions. This has ushered in a new era where predictive maintenance, powered by Artificial Intelligence (AI), plays a pivotal role in maintaining robust IT infrastructures.
Understanding Predictive Maintenance
Predictive maintenance is a Proactive approach that involves using data-driven insights to predict when an IT system or component might fail, allowing for timely intervention before any disruption occurs. Unlike traditional reactive maintenance, which addresses issues after they have arisen, predictive maintenance aims to foresee potential problems and mitigate them preemptively.
The integration of AI into this domain has revolutionized how organizations manage their IT infrastructure. AI algorithms analyze vast amounts of data generated by systems in real-time, identifying patterns and anomalies that could indicate impending failures.
The Role of AI in Predictive Maintenance
data Collection and analysis
AI excels at processing large volumes of data quickly and accurately. In the context of IT infrastructure, this involves collecting data from various sources such as network logs, server performance metrics, application usage statistics, and more. machine learning models can then analyze this data to identify trends and detect anomalies that might suggest potential issues.
Types of data Collected
- Network Logs: These logs provide insights into network traffic patterns, latency, packet loss, and other critical metrics that can indicate network health.
- Server Performance Metrics: CPU usage, memory utilization, disk I/O, and temperature readings are essential for understanding server performance and potential bottlenecks.
- Application Usage Statistics: Monitoring application performance helps identify issues related to software bugs, resource allocation, and user experience.
- Environmental sensors: data from sensors measuring humidity, temperature, and other environmental factors can help predict hardware failures due to adverse conditions.
pattern recognition
One of AI's strengths is its ability to recognize patterns within complex datasets. By continuously monitoring IT systems, AI algorithms can learn what normal operation looks like and flag deviations from the norm. This capability is crucial for identifying subtle signs of wear or impending failure that might be missed by human analysts.
Techniques Used in pattern recognition
- Clustering: Grouping similar data points together to identify patterns and outliers.
- Classification: Categorizing data into predefined classes based on learned patterns.
- Regression analysis: Predicting continuous values based on historical data trends.
anomaly detection
AI-driven anomaly detection goes beyond simple pattern recognition. Advanced machine learning models, such as Neural Networks and Deep Learning algorithms, can identify complex patterns indicative of potential failures. These models are trained on historical data to understand what constitutes normal behavior and can alert IT teams when something unusual occurs.
Methods for anomaly detection
- statistical methods: Using statistical measures like mean, standard deviation, and z-scores to detect deviations from the norm.
- Machine Learning Models: Employing algorithms such as Support Vector Machines (SVM), Random Forests, and Autoencoders to identify anomalies.
- Deep Learning Techniques: Utilizing Convolutional Neural Networks (CNNs) and Recurrent Neural Networks (RNNs) for more complex anomaly detection tasks.
predictive modeling
predictive modeling involves using AI to forecast future events based on current and historical data. In predictive maintenance, this means estimating the remaining useful life of IT components or predicting when a system might fail. By doing so, organizations can schedule maintenance activities at optimal times, minimizing downtime and extending the lifespan of their infrastructure.
Key Components of predictive modeling
- Time Series analysis: Analyzing sequential data points to identify trends and make future predictions.
- Survival analysis: Estimating the time until an event (e.g., system failure) occurs based on historical data.
- Monte Carlo Simulations: Using probabilistic modeling to simulate various scenarios and predict outcomes.
Automated decision-making
AI can also automate decision-making processes in predictive maintenance. For instance, if an AI model predicts that a server is likely to fail within the next 24 hours, IT can automatically trigger a maintenance request or even initiate a failover process to ensure Business Continuity.
Benefits of Automated decision-making
- Reduced Response Time: Immediate actions based on real-time data reduce the time between detection and resolution.
- Consistency: Automated systems follow predefined rules, ensuring consistent decision-making processes.
- Scalability: AI can handle large volumes of data and make decisions across multiple systems simultaneously.
Use Cases of AI in Predictive Maintenance
Server Monitoring
Servers are the backbone of IT infrastructure, and their failure can have catastrophic consequences. AI-powered predictive maintenance can monitor server performance metrics in real-time, detect anomalies, and predict potential failures before they occur.
Example Scenario
A data center uses AI to monitor CPU usage, memory utilization, and disk I/O on its servers. The AI system detects a sudden spike in CPU usage on Server A, which is unusual for that time of day. IT also notes that the server's temperature has been rising steadily over the past hour. Based on historical data, the AI predicts an 80% chance of failure within the next two hours. IT automatically triggers a maintenance request and initiates a failover to another server, ensuring continuous operation.
network management
Network issues can disrupt communication, data transfer, and overall system performance. AI can help predict network failures by analyzing traffic patterns, latency, packet loss, and other critical metrics.
Example Scenario
A telecommunications company uses AI to monitor its network infrastructure. The AI system detects an unusual increase in packet loss on a specific route, which is accompanied by higher-than-normal latency. Based on historical data, the AI predicts a 75% chance of a network outage within the next hour. IT automatically reroutes traffic through alternative paths and notifies the network operations team to investigate the issue.
Application Performance
applications are the frontline of user interaction with IT systems. Ensuring their performance is crucial for maintaining user satisfaction and Business Continuity. AI can monitor application usage statistics, detect anomalies, and predict potential issues.
Example Scenario
An e-commerce platform uses AI to monitor its web application's performance. The AI system detects a sudden increase in response times and a spike in error rates during peak shopping hours. Based on historical data, the AI predicts a 90% chance of a complete application crash within the next 30 minutes. IT automatically scales up server resources and notifies the development team to address the underlying issue.
environmental monitoring
environmental factors such as temperature, humidity, and air quality can significantly impact IT infrastructure. AI can monitor these factors in real-time, detect anomalies, and predict potential hardware failures due to adverse conditions.
Example Scenario
A cloud service provider uses AI to monitor environmental sensors in its data centers. The AI system detects a sudden drop in temperature in one of the Server Rooms, which is accompanied by increased humidity levels. Based on historical data, the AI predicts a 60% chance of hardware failure within the next hour due to condensation. IT automatically adjusts the HVAC settings and notifies the facilities management team to investigate the issue.
challenges and Considerations
While AI offers numerous benefits for predictive maintenance in IT infrastructure, there are several challenges and considerations to keep in mind.
data Quality and Availability
AI models rely on high-quality data to make accurate predictions. Ensuring data accuracy, completeness, and timeliness is crucial for effective predictive maintenance.
Strategies for Improving data Quality
- data Cleaning: Removing or correcting inaccurate, incomplete, or irrelevant data.
- data integration: Combining data from multiple sources to provide a comprehensive view of the system.
- Real-Time Monitoring: Continuously updating data to reflect current conditions and trends.
Model accuracy and Validation
Ensuring the accuracy of AI models is essential for reliable predictions. Regular validation and updating of models are necessary to maintain their effectiveness.
Techniques for Improving Model accuracy
- Cross-Validation: Using a subset of data to train the model and another subset to validate its performance.
- Hyperparameter Tuning: Adjusting model parameters to optimize performance.
- continuous learning: Updating models with new data to adapt to changing conditions.
Human Expertise
While AI can automate many aspects of predictive maintenance, human expertise remains essential. IT professionals are needed to interpret AI-generated insights, validate predictions, and implement appropriate actions.
Roles of Human Experts
- data Scientists: Developing and refining AI models for predictive maintenance.
- IT Professionals: Implementing maintenance actions based on AI predictions.
- Facilities Managers: Addressing environmental issues identified by AI systems.
Ethical Considerations
The use of AI in predictive maintenance raises ethical considerations, such as data privacy, security, and the potential for job displacement.
Addressing ethical concerns
- data privacy: Ensuring that personal data is protected and used responsibly.
- security: Implementing robust security measures to protect AI systems from cyber threats.
- job displacement: Providing training and support for employees whose roles may be impacted by AI.
future trends in AI-Powered Predictive Maintenance
The field of AI-powered predictive maintenance is rapidly evolving, with several emerging trends poised to shape its future.
Edge Computing
Edge computing involves processing data closer to where IT is collected, reducing latency and improving response times. This approach can enhance the effectiveness of AI-powered predictive maintenance by enabling real-time decision-making.
Benefits of Edge Computing
- Reduced latency: Faster response times for critical issues.
- Improved reliability: Reduced dependence on centralized systems.
- enhanced security: data is processed locally, reducing the risk of cyber threats.
Internet of Things (IoT)
The IoT involves connecting physical devices to the internet, enabling them to collect and share data. This technology can provide valuable insights for predictive maintenance by monitoring equipment performance in real-time.
Benefits of IoT
- Real-Time Monitoring: Continuous data collection and analysis.
- Enhanced Visibility: Comprehensive view of system performance.
- Improved efficiency: Automated detection and resolution of issues.
Artificial General Intelligence (AGI)
AGI refers to AI systems that can understand, learn, and apply knowledge across a wide range of tasks, similar to human intelligence. While still in the early stages of development, AGI has the potential to revolutionize predictive maintenance by enabling more sophisticated and adaptive decision-making.
Potential Benefits of AGI
- advanced analytics: More accurate predictions and insights.
- adaptive learning: continuous improvement based on new data.
- Autonomous decision-making: Enhanced ability to make complex decisions without human intervention.
AI-powered predictive maintenance offers significant benefits for IT infrastructure, including improved reliability, Reduced Downtime, and enhanced performance. By leveraging advanced analytics, real-time monitoring, and automated decision-making, organizations can proactively address potential issues before they impact operations. However, achieving these benefits requires careful consideration of data quality, model accuracy, human expertise, and ethical concerns. As the field continues to evolve, emerging technologies such as edge computing, IoT, and AGI hold promise for even greater advancements in predictive maintenance.
By embracing AI-powered predictive maintenance, organizations can ensure the reliability, efficiency, and performance of their IT infrastructure, ultimately driving business success and Innovation.