Understanding Machine Learning for Structural Engineering: Predicting Failures
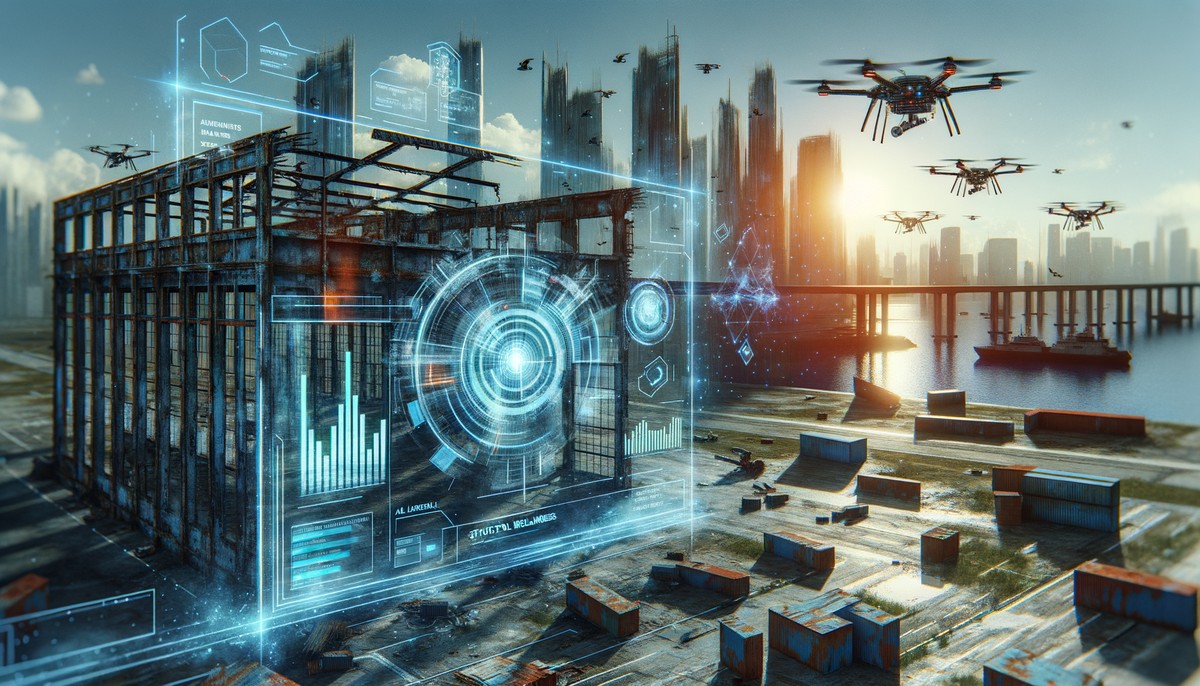
There are few areas where predicting failure is as crucial as in structural engineering. The consequences of unforeseen problems in structures, such as bridges, buildings or dams, can be catastrophic, affecting not only fiscal aspects but also the very lives of people. As a result, structural engineering has always found IT important to develop better ways to anticipate and counter potential failures. Enter Machine Learning (ML).
Machine Learning is a subset of artificial intelligence (AI) that focuses on developing algorithms that enable computers to learn from and make decisions based on data. Its potential applications in structural engineering are vast, notably in predicting structural failures. Here we shall delve into how Machine Learning applies to structural engineering to predict failures.
Basics of Machine Learning in the context of Structural Engineering
machine learning models can learn from structural health monitoring data, patterns of previous failures, and other related information to Predict Failure. This involves processing vast amounts of raw data and then using algorithms to identify patterns and relationships that human engineers might easily miss.
There are two general types of ML models relevant to structural engineering: supervised learning and unsupervised learning. Supervised learning models learn from labelled data, meaning the input data paired with correct output. They are especially effective in predicting failures based on past data. Unsupervised learning models, on the other hand, function without labelled data. They identify patterns purely based on the input.
Machine Learning's Role in Predicting Structural Failure
ML models can predict structural failure in several ways. They can be used to forecast deterioration in aging infrastructure, helping to schedule maintenance and prevent structural failure. They can also analyze new designs, identifying potential weak points and areas prone to failures.
In addition, these models are highly scalable. Engineers can use the same analytic model on a wide variety of structures, from small bridges to massive dams or skyscrapers.
Case study: Predicting Concrete Strength Using Machine Learning
Concrete is a common material in structural engineering, yet its strength can fluctuate due to several factors, like mixture proportions, air content, and curing time.
Machine Learning can be used here to predict concrete strength based on these variables. An ML model is trained on existing data to understand the relationships between the input variables (mixture proportions, air content, and curing time) and the output (concrete strength). This model can then be used to predict the strength of future concrete batches, substantially improving the structural planning process.
Potential challenges and solutions
While the potential of machine learning in structural engineering is vast, realizing that potential isn't without challenges. The accuracy of the ML models is dependent on the quality and abundance of data. Obtaining a sufficient and relevant dataset may prove difficult in some scenarios.
Furthermore, while machine learning can help identify patterns and make predictions, IT cannot replace the nuanced judgment of experienced human engineers. Therefore, IT is crucial to use machine learning as a tool to aid decision-making, rather than IT being the sole decision-maker.
Conclusion
The application of Machine Learning in Structural Engineering, particularly in predicting failures, represents a significant step forward for the sector. IT could help proactively prevent disasters, save costs, and improve overall structural integrity. Like any tool, IT's not without its challenges, but with thoughtful implementation, machine learning holds great promise for the future of structural engineering.