Exploring the Limits of AI in Predictive Science and Engineering
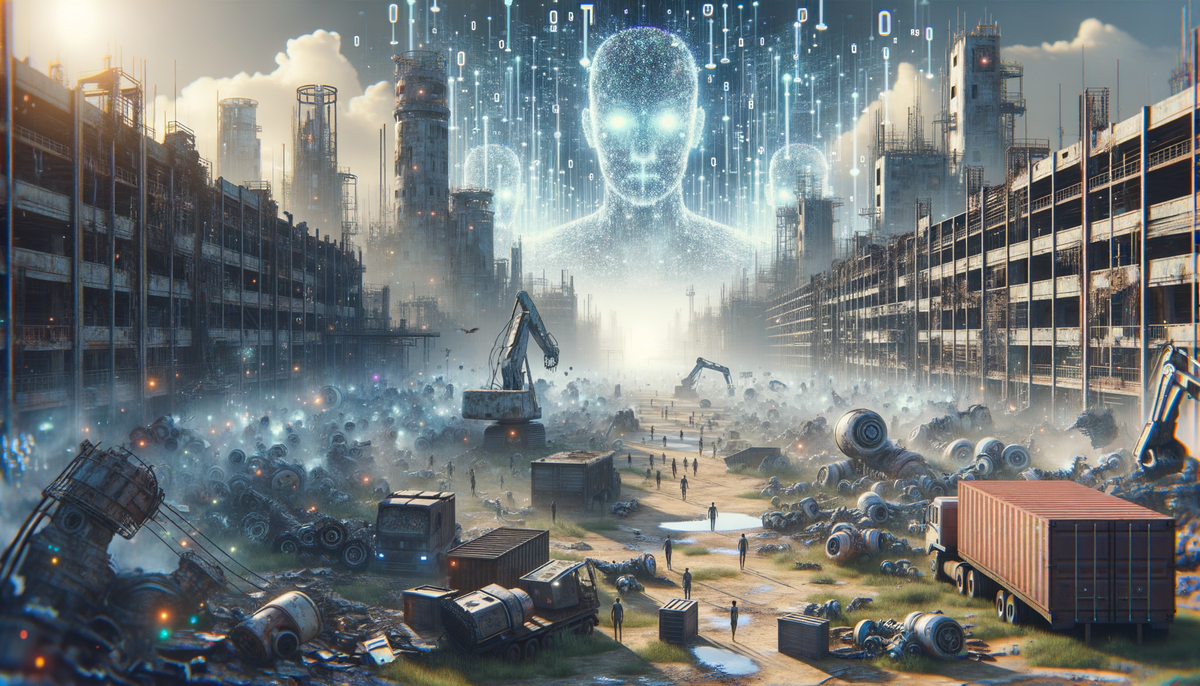
AI, or Artificial Intelligence, is one of the most incredible innovations of our time. In the last few years, IT has taken a dominant position in various fields, including Predictive Science and Engineering. However, like any other technologies, AI too has its own limitations and constraints.
The debate around the potential and limitations of AI in predictive science and engineering has piqued a lot of interest. With AI's capability to process vast amounts of data and derive insights for future predictions, the potential seems limitless. Yet, the more we delve into the space, the more we realize that there are still boundaries and complexities yet to be understood.
At the core of predictive science and engineering lie Simulations that attempt to predict future occurrences based on historical data. Machine learning, an important arm of AI, can analyze extensive data sets to predict trends and patterns, playing a crucial role in these Simulations.
machine learning algorithms develop models that are able to recognize patterns in historical data, allowing these models to make predictions about future events. Yet, these AI models are only as good as the data they are trained on. If the data is biased, incomplete, or not diverse, the AI's predictions would not be accurate.
In engineering, AI and machine learning aid in predicting and solving complex engineering tasks, like choosing the best materials for manufacturing or anticipating the breakdown of machinery. However, these systems need to undergo constant training and fine-tuning to make accurate predictions.
Moreover, AI is challenged by the Interpretation of the data IT analyzes. AI does not understand context, emotion, or contradictions. If there's a sharp contradiction in the data set, AI can falter. This can be a limiting factor in the application of AI in predictive science and engineering, where contextual understanding can be crucial.
Uncertainty and predictive inaccuracies remain other limitations. Futuristic predictions involve variables and contingencies that are often difficult for AI to comprehend. Despite being programmed to handle complex data and draw insights, AI still struggles in situations with too many variables and unknowns.
The field of AI is still evolving and developing. While AI has proven its potential in predictive science and engineering, IT is essential to keep exploring its limitations. These challenges and limitations also mark the areas where research and development can further hone the application of AI in predictive science and engineering.
In conclusion, while AI does have its limitations in predictive science and engineering, IT's awe-inspiring how far we've come and how much potential lies ahead. With increased understanding, ongoing research, and continuous advancements, the influence and effectiveness of AI in predictive science and engineering are only bound to grow.
As the world inches closer to a future dominated by AI and technology, understanding these nuances and bridging the gaps becomes more critical. The journey may be filled with potential hiccups and roadblocks, but IT also promises an exhilarating exploration to the limits of AI in predictive science and engineering.