Predicting and Responding to Crises: How AI is Revolutionizing Disaster Management
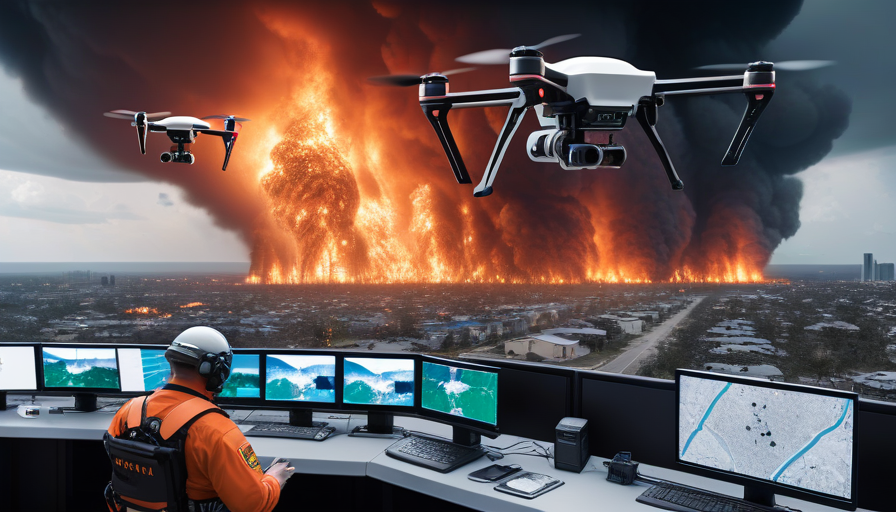
In an era marked by increasing environmental volatility, the necessity for robust disaster management strategies has never been more pronounced. Natural disasters such as hurricanes, earthquakes, floods, and wildfires are becoming more frequent and intense due to climate change. Traditional methods of crisis prediction and response often fall short in terms of speed, accuracy, and effectiveness. However, with the advent of artificial intelligence (AI), there is a new frontier in disaster management that promises unprecedented advancements.
AI: A Game Changer for Crisis prediction
Predicting crises effectively involves analyzing vast amounts of data to identify potential threats before they escalate into full-blown disasters. This is where AI comes into play. By leveraging machine learning algorithms, Natural Language Processing (NLP), and advanced analytics, AI systems can sift through terabytes of data from various sources such as satellite imagery, social media feeds, weather patterns, and historical records to detect early warning signs.
Machine Learning for pattern recognition
machine learning models are capable of recognizing complex patterns that elude human analysts. For instance, by training on historical data, these algorithms can predict the likelihood of a natural disaster occurring in specific regions with remarkable accuracy. In earthquake-prone areas, AI systems analyze seismic activity to forecast potential quakes. Similarly, for hurricanes and floods, machine learning models evaluate weather data and oceanic conditions to anticipate storm paths and intensities.
Case Study: earthquake prediction
One notable example is the use of machine learning in predicting earthquakes. Traditional seismology relies heavily on historical data and expert analysis, which can be time-consuming and sometimes inaccurate. machine learning algorithms, however, can process real-time seismic data from numerous sensors deployed across a region. By identifying subtle patterns and anomalies that precede an earthquake, these models can provide early warnings, giving people precious minutes to evacuate or take protective measures.
Case Study: Hurricane Tracking
Hurricane tracking is another area where machine learning has shown significant promise. Traditional methods involve analyzing satellite images and weather data manually, which can be slow and prone to human error. machine learning models, on the other hand, can process vast amounts of data in real-time, predicting storm paths with high accuracy. For example, during Hurricane Dorian in 2019, AI-driven models provided timely updates on the storm's trajectory, helping authorities evacuate vulnerable areas and deploy resources more effectively.
The Role of Satellite Imagery
Satellite imagery provides a bird’s-eye view of the Earth's surface, capturing real-time changes that can indicate impending disasters. AI algorithms process this imagery to detect anomalies such as unusual cloud formations or land movements. These insights allow for timely alerts about potential natural disasters like wildfires or landslides, facilitating quicker response times and potentially saving lives.
Case Study: Wildfire Detection
Wildfires are a growing concern in many regions due to climate change and human activities. Satellite imagery combined with AI can detect the early signs of wildfires, such as smoke plumes or heat signatures, before they become uncontrollable. For instance, in California, AI systems have been deployed to monitor forest areas continuously. When an anomaly is detected, alerts are sent to firefighters, enabling them to respond swiftly and contain the fire before IT spreads.
Social Media Analytics
Social media platforms like Twitter and Facebook can provide valuable real-time data during disasters. People often share updates, photos, and videos from affected areas, creating a wealth of information that AI systems can analyze. NLP algorithms can sift through this data to identify trends, gauge the severity of the situation, and even locate individuals in need of assistance.
Case Study: Flood Response
During floods, social media analytics can play a crucial role in coordinating rescue efforts. For example, during the 2017 Hurricane Harvey in Texas, AI systems analyzed tweets to identify areas with high water levels and people trapped in their homes. This information was relayed to emergency responders, who could then prioritize their efforts and save lives.
Enhancing Response Strategies with AI
While predicting disasters is a critical aspect of disaster management, the response strategies are equally important. AI can enhance response efforts by providing real-time data, optimizing resource allocation, and improving communication among stakeholders.
Real-Time data integration
During a crisis, having access to real-time data is crucial for making informed decisions. AI systems can integrate data from various sources, such as sensors, drones, and social media, to provide a comprehensive view of the situation. This enables emergency responders to assess the damage, identify priority areas, and deploy resources more effectively.
Case Study: Tsunami Response
In coastal regions prone to tsunamis, real-time data integration can save lives. AI systems can monitor seismic activity and ocean currents in real-time, providing early warnings to residents. Additionally, drones equipped with cameras and sensors can survey affected areas, helping responders assess the damage and plan their response.
Optimizing resource allocation
Efficient resource allocation is essential during a disaster. AI can optimize this process by analyzing data on available resources, such as personnel, equipment, and supplies, and matching them with the needs on the ground. This ensures that resources are used effectively and that critical areas receive the support they need.
Case Study: Earthquake Relief
After an earthquake, the immediate response involves search and rescue operations, medical assistance, and providing shelter to affected populations. AI systems can analyze data on available resources and prioritize their deployment based on the severity of the situation. For example, in Nepal's 2015 earthquake, AI-driven models helped coordinate relief efforts by matching available resources with areas of greatest need.
Improving Communication
Effective communication is vital during a disaster. AI can enhance communication among stakeholders by providing real-time updates, facilitating coordination, and ensuring that information is disseminated accurately and quickly. For instance, chatbots powered by NLP can answer frequently asked questions, provide updates on the situation, and offer guidance to affected individuals.
Case Study: Hurricane Response
During hurricanes, communication can be disrupted due to power outages and damaged infrastructure. AI-powered systems can help maintain communication channels by providing real-time updates via mobile apps or social media platforms. For example, during Hurricane Maria in Puerto Rico, AI-driven chatbots provided information on evacuation routes, shelter locations, and emergency services.
Ethical Considerations and challenges
While AI offers numerous benefits for disaster management, IT also presents ethical considerations and challenges that must be addressed to ensure its responsible use.
bias and fairness
AI systems are only as unbiased as the data they are trained on. If the training data is biased or incomplete, the AI system may produce unfair outcomes. For example, if an earthquake prediction model is trained primarily on data from urban areas, IT may not perform well in rural regions, leading to disparities in response efforts.
Addressing Bias
To address bias, IT is essential to use diverse and representative datasets for training AI models. Additionally, transparency in the development process can help identify and mitigate biases. Regular audits of AI systems can also ensure that they are performing fairly across different demographics.
privacy Concerns
AI systems often rely on large amounts of data, including personal information from social media or other sources. This raises concerns about privacy and data protection. During disasters, people may be more vulnerable to privacy breaches, as they share sensitive information in the hope of receiving assistance.
Protecting privacy
To protect privacy, IT is crucial to implement robust data protection measures. Anonymizing personal data before analysis can help safeguard individuals' identities. Additionally, clear guidelines on data usage and consent can ensure that people's privacy rights are respected.
accountability and transparency
AI systems can make decisions that have significant impacts on people's lives. IT is essential to hold these systems accountable for their actions and ensure transparency in their decision-making processes. This includes providing explanations for AI-driven recommendations and ensuring that stakeholders understand how the system works.
Ensuring accountability
To ensure accountability, IT is important to establish clear guidelines for the use of AI in disaster management. Regular audits and evaluations can help identify any issues with the system's performance or fairness. Additionally, involving stakeholders in the development process can enhance transparency and build trust.
Future Directions and Innovations
The field of AI in disaster management is rapidly evolving, with new technologies and approaches emerging all the time. Some of the most promising areas for future Innovation include:
integration with IoT and Blockchain
Integrating AI with the Internet of Things (IoT) and Blockchain technology can enhance its capabilities further. IoT devices can provide real-time data from affected areas, while Blockchain ensures secure and transparent sharing of information among stakeholders.
Case Study: Smart Cities
In Smart Cities, IoT sensors can monitor various aspects of urban life, such as traffic flow, air quality, and energy consumption. During a disaster, these sensors can provide valuable data on the situation, helping responders assess the damage and plan their response. Blockchain technology can ensure that this data is shared securely and transparently among stakeholders.
advanced analytics and Machine Learning
advanced analytics and machine learning Techniques can improve AI's ability to predict disasters and optimize response efforts. For example, Deep Learning algorithms can analyze complex datasets to identify patterns and make accurate predictions about future events.
Case Study: Flood prediction
Flood prediction is a critical aspect of disaster management. Deep Learning models can analyze historical data on rainfall, river levels, and other factors to predict the likelihood of flooding in specific areas. This information can help authorities prepare for potential floods and take Preventive Measures.
Human-AI Collaboration
Effective disaster management requires Collaboration between humans and AI systems. By leveraging each other's strengths, they can achieve better outcomes than either could alone. For example, AI can provide real-time data and recommendations, while human responders use their expertise to make informed decisions.
Case Study: search and rescue Operations
In search and rescue operations, AI-powered drones can survey affected areas and identify potential survivors. Human responders can then use this information to plan their rescue efforts more effectively. This Collaboration ensures that both the technology's capabilities and human expertise are utilized to save lives.
AI has the potential to revolutionize disaster management by Enhancing prediction accuracy, optimizing response strategies, and improving communication among stakeholders. However, IT also presents ethical considerations and challenges that must be addressed to ensure its responsible use. By integrating AI with other technologies, such as IoT and Blockchain, and fostering human-AI Collaboration, we can create more effective and resilient disaster management systems.
As the field continues to evolve, IT is essential to stay informed about new developments and innovations in AI for disaster management. This will enable us to leverage these technologies effectively and build a safer, more resilient world for all.