Natural Language Generation: Transforming Data into Narratives
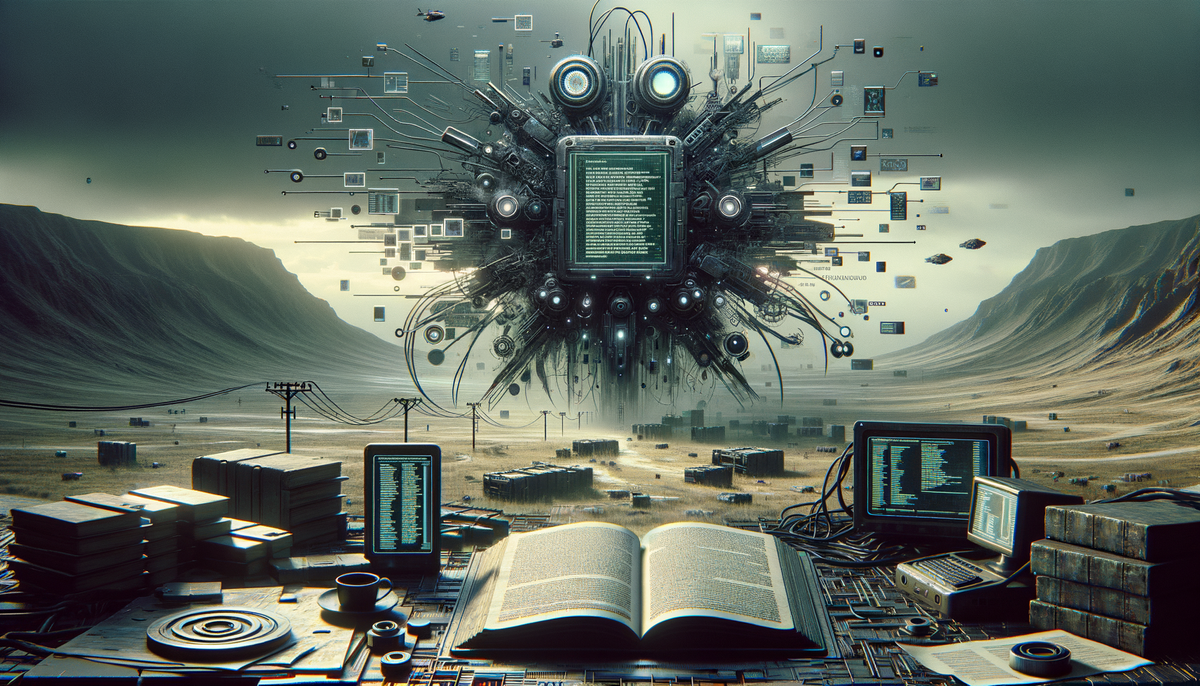
In the era of big data, where information is abundant and often overwhelming, the ability to convert raw data into coherent, engaging narratives is invaluable. This is where Natural Language Generation (NLG) steps in. NLG is a fascinating branch of artificial intelligence that focuses on transforming structured data into human-readable text. Not only does IT enhance data accessibility, but IT also makes data actionable by weaving stories that resonate with users.
Understanding Natural Language Generation
NLG involves the automated production of text through computational means. IT’s a subset of Natural Language Processing (NLP), which encompasses all mechanisms allowing machines to understand and produce human language. While NLP deals with language understanding, NLG focuses specifically on language production. The goal is to enable machines to articulate insights derived from data in a manner akin to how humans would communicate them.
How Does NLG Work?
NLG involves several stages that transform data into narratives:
-
Content Determination: This phase involves determining what information from the dataset is relevant for the narrative. IT requires understanding the user’s needs and the context within which the data will be presented.
-
Document Structuring: Once the content is determined, IT is structured into a logical sequence. This process is akin to creating an outline for an essay.
-
Sentence Aggregation: Here, information is grouped into sentences. The goal is to make the narrative concise and easy to understand.
-
Lexicalization: This involves choosing the right words to convey the desired meaning. IT requires a good understanding of the context and audience.
-
Referring Expression Generation: This step involves generating phrases that refer back to entities or concepts introduced earlier in the text.
-
Linguistic Realization: Finally, grammatical rules are applied to ensure that sentences are well-formed and convey the intended meaning correctly.
applications of NLG
The applications of NLG are vast and varied, reaching into numerous industries and sectors. Here are a few noteworthy examples:
-
Business intelligence: NLG converts complex analytics data into simple textual summaries, making IT easier for decision-makers to grasp insights and take action.
-
financial services: IT’s used to generate reports from numerical data, saving time and reducing the potential for human error.
-
healthcare: Doctors and medical practitioners utilize NLG to convert patient data into succinct reports, facilitating better patient care and management.
-
e-commerce: Product descriptions, which can be very tedious to write at scale, are often generated using NLG to engage potential buyers effectively.
-
media and entertainment: NLG is leveraged to draft sports reports, news articles, and other narrative forms that require quick, reliable publication.
Benefits of Natural Language Generation
NLG offers numerous benefits that enhance how businesses and individuals engage with data:
-
Scalability: One of its most significant advantages is the ability to produce content at scale. Organizations can generate reports, summaries, articles, and more, without the constraints of time and human resources.
-
Consistency and accuracy: Automated narratives remove potential biases and errors inherent in human writing, ensuring that all generated content is consistent, accurate, and of high quality.
-
cost efficiency: Companies can save substantial resources by implementing NLG solutions instead of dedicating manual labor to content creation.
-
personalization: NLG systems can tailor narratives to specific audiences, crafting personalized messages that enhance user engagement and satisfaction.
-
Improved Decision Making: By transforming complex data into understandable narratives, decision-makers can better comprehend information, leading to more informed decisions.
challenges in NLG
Despite its potential, NLG is not without challenges:
-
data Quality: Poor quality or unstructured data can impede the NLG process, leading to inaccurate or misleading narratives.
-
context understanding: Machines still struggle with comprehending nuances and context in language, which can affect the accuracy and relevance of the generated content.
-
creativity and Style: While machines can mimic human language to an extent, reproducing the creative and stylistic elements of human writing remains a challenge.
-
Ethical Considerations: The ability to generate human-like narratives raises ethical questions, particularly concerning misinformation and content authenticity.
The Future of Natural Language Generation
The future of NLG looks promising, with ongoing advancements seeking to address current challenges and expand its capabilities. Key trends to watch include:
-
integration with Other AI technologies: NLG is increasingly integrated with machine learning and AI systems for more sophisticated applications, such as chatbots and virtual assistants.
-
Improved contextual understanding: As NLP and machine learning evolve, so will the ability of NLG systems to understand context, leading to more accurate and detailed narratives.
-
Greater personalization and customization: Future NLG solutions will likely offer more customization options, allowing businesses to fine-tune narratives closely aligning with their brand voice and audience needs.
-
Enhanced data Visualization: NLG will work alongside data visualization Tools, not just to tell stories with data but to present them in engaging, multi-dimensional formats.
In conclusion, Natural Language Generation is revolutionizing how we interact with data, turning raw numbers and facts into meaningful stories that inform and inspire. As technology progresses, the narratives generated by machines will become increasingly indistinguishable from those crafted by humans, opening new realms of possibility in communication and storytelling.