Machine Learning for Enhancing Cloud Storage Solutions
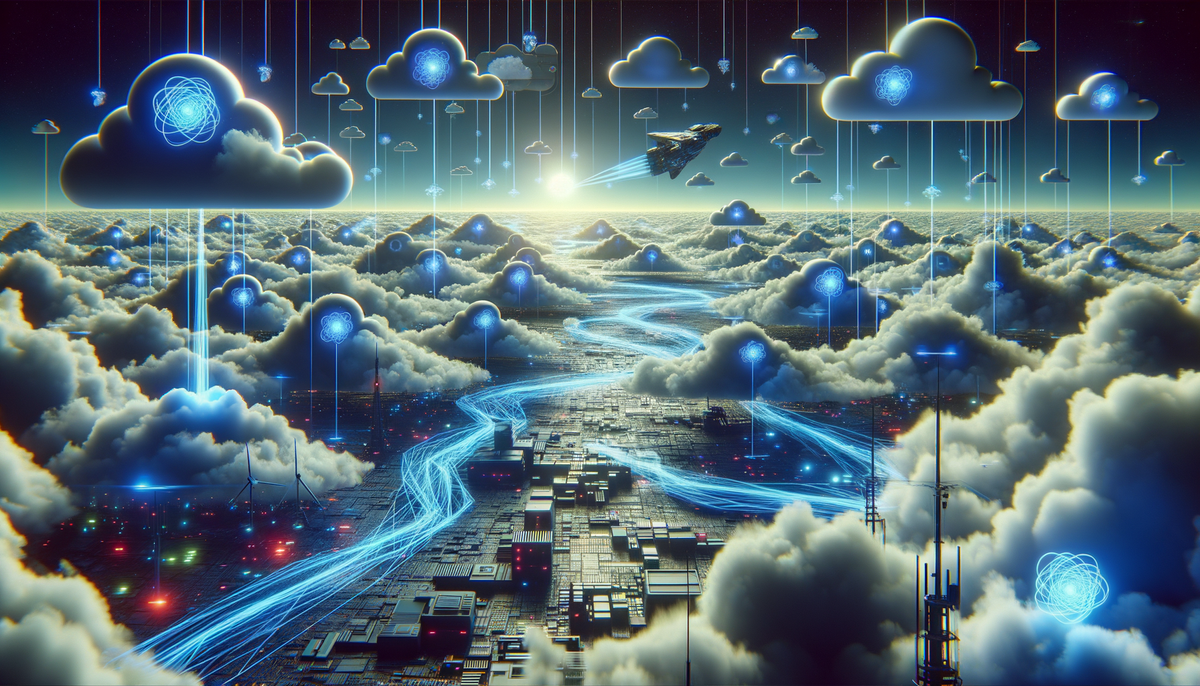
cloud storage solutions have revolutionized the way businesses store and manage data. The paradigm shift towards remote storage offered by cloud technology is juxtaposed with the increasing need for efficient data management, security, and Cost-Effectiveness. This is where Machine Learning (ML) comes into play, acting as a catalyst for Enhancing cloud storage solutions.
The Role of Machine Learning in cloud storage
1. Intelligent Data Management
Gone are the days when data management was a cumbersome task involving manual sorting and indexing. Machine Learning algorithms can now parse through vast amounts of data, recognizing patterns and compartmentalizing them in a way that boosts retrieval efficiency. This intelligent data sorting ensures that frequently accessed data is readily available while archiving less-utilized data, making the entire process seamless and fast.
2. Predictive Maintenance
cloud storage infrastructure comprises sophisticated hardware that needs regular maintenance. Machine Learning models can analyze trends and predict failures before they occur. By identifying potential hardware malfunctions in advance, ML ensures minimal downtime and reduces maintenance costs significantly. Predictive maintenance employs anomaly detection algorithms that scrutinize operational data to foresee system disruptions, thereby maintaining a high uptime rate.
3. enhanced security Mechanisms
One of the primary concerns for businesses using cloud storage is data security. Machine Learning can provide robust security protocols by detecting unusual patterns indicative of cyber threats. anomaly detection models and Real-time analytics contribute to a fortified cloud storage Environment, driving off potential breaches and ensuring data integrity.
4. Cost Optimization
Machine Learning algorithms excel at analyzing usage patterns, which can be used to forecast storage needs and optimize resource allocation. By predicting future data storage requirements, companies can dispense with the need for redundant hardware. Furthermore, this level of foresight allows for better planning and budgeting, significantly lowering the overall cost of cloud storage solutions.
5. Improved Data Retrieval
Implementing Machine Learning can substantially enhance data retrieval times. Natural Language Processing (NLP) and semantic search capabilities enable users to find the exact data they need with simple queries. For instance, a user looking for financial reports from Q3 2021 can do so effortlessly by entering a straightforward search query, thanks to ML-enhanced search functionalities.
ML Techniques for cloud storage Enhancement
A. Supervised Learning
Supervised learning algorithms are used to create Predictive Models based on historical data. This helps in understanding data usage trends and future storage requirements. Regression models can predict storage growth, enabling better capacity planning.
B. Unsupervised Learning
Unsupervised learning algorithms, such as clustering, can segment data into meaningful groups without prior labeling. This helps in organizing data based on similarities and usage patterns, which can be instrumental in tiered storage solutions.
C. Reinforcement Learning
Reinforcement Learning (RL) can optimize resource allocation in real-time. An RL model can be trained to manage storage resources more efficiently, balancing between speed and cost to ensure optimal performance.
D. Deep Learning
Deep Learning models, particularly Convolutional Neural Networks (CNNs) and Recurrent Neural Networks (RNNs), can provide enhanced data categorization and prediction capabilities. These models can analyze large streams of unstructured data, deriving meaningful insights that can be used to improve storage solutions.
Real-world applications
1. Google Cloud and AutoML
Google Cloud uses AutoML to enable users to create customized ML models that can analyze and sort data, improve search functionalities, and build predictive analytics solutions without needing deep expertise in machine learning.
2. Amazon Web Services (AWS) and SageMaker
AWS SageMaker offers a suite of Tools designed to incorporate ML into cloud storage management. IT facilitates everything from data labeling to model training and deployment, making IT easier for businesses to adopt ML-driven storage solutions.
3. Microsoft Azure and Azure Machine Learning
Azure's Machine Learning services provide robust Tools for developing Predictive Models that can enhance data security and optimize storage utilization, ensuring a streamlined and secure cloud storage Environment.
Conclusion
Machine Learning continues to play an instrumental role in transforming cloud storage solutions. From predictive maintenance and enhanced security to cost optimization and better data retrieval, ML's impact is profound and far-reaching. As technology evolves, the symbiotic relationship between Machine Learning and cloud storage is only expected to grow stronger, delivering more sophisticated and efficient solutions for businesses worldwide.