Enhancing Business Intelligence with AI
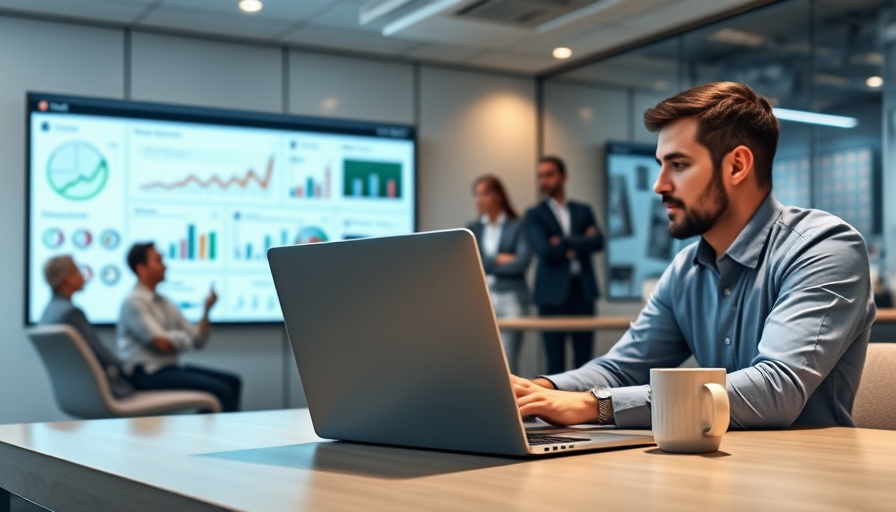
Business intelligence (BI) has long been a cornerstone for organizations seeking to make data-driven decisions. By leveraging data analysis and business analytics Tools, BI helps companies understand their operations, identify trends, and make informed strategic choices. However, the integration of artificial intelligence (AI) is revolutionizing BI by providing deeper insights, automating repetitive tasks, Enhancing overall efficiency, and enabling Predictive Capabilities. In this comprehensive post, we'll explore how AI can significantly enhance business intelligence strategies, delve into key concepts, and provide detailed examples to illustrate these advancements.
Understanding Business Intelligence
Business intelligence encompasses a variety of Tools, applications, and methodologies that allow organizations to collect, analyze, and present data to make informed decisions. Traditional BI relies on structured data and predefined queries to generate reports and dashboards. However, with the advent of AI, BI has evolved to handle unstructured data, provide predictive analytics, and offer more dynamic and interactive insights.
Key Components of Business Intelligence
- data Warehousing: Centralized repositories where data from various sources is stored and managed.
- ETL Processes: Extract, Transform, Load processes that cleanse and integrate data from different sources.
- Reporting and Querying: Tools that allow users to generate reports and run queries on the data.
- Online Analytical Processing (OLAP): Multidimensional data analysis that enables users to interactively explore data from various angles.
- data Visualization: Graphical representations of data, such as charts and graphs, to make information more understandable.
The Role of AI in Enhancing Business Intelligence
AI can transform business intelligence in several ways, addressing some of the limitations of traditional BI and opening up new possibilities for data analysis and decision-making.
1. Predictive Analytics
Predictive analytics involves using statistical algorithms and Machine Learning Techniques to identify patterns in historical data and predict future trends or behaviors. AI-driven predictive analytics can provide several benefits:
- forecasting: Anticipating future sales, market trends, or customer behavior.
- Risk Management: Identifying potential risks and taking proactive measures.
- Churn prediction: Predicting which customers are likely to churn and implementing retention strategies.
Example: A retail company uses predictive analytics to forecast demand for its products. By analyzing historical sales data, seasonal trends, and external factors like weather patterns, the AI model can predict which products will be in high demand during specific periods. This allows the retailer to optimize inventory levels, reduce stockouts, and minimize excess inventory.
2. natural language processing (NLP)
natural language processing (NLP) is a branch of AI that enables computers to understand, interpret, and generate human language. In the context of BI, NLP can enhance data interaction and accessibility:
- Conversational Interfaces: Allowing users to query data using natural language, making BI Tools more accessible to non-technical users.
- Sentiment analysis: Analyzing customer feedback or social media posts to gauge public sentiment towards products or services.
- Text Mining: Extracting valuable insights from unstructured text data, such as emails, documents, or customer reviews.
Example: A financial services company implements an NLP-powered chatbot that allows employees to ask questions about financial performance in plain language. The chatbot can understand queries like What were the total sales last quarter? and provide instant answers by querying the underlying data warehouse.
3. Automated data Preparation
data preparation is often a time-consuming and error-prone process in traditional BI. AI can automate this task by identifying patterns, cleaning data, and integrating disparate sources more efficiently:
- data Cleaning: Automatically detecting and correcting errors or inconsistencies in the data.
- data integration: Combining data from multiple sources, even if they have different formats or structures.
- Feature Engineering: Creating new variables or features that improve the performance of Predictive Models.
Example: A healthcare organization receives patient data from various sources, including electronic health records (EHRs), lab results, and wearable devices. AI-powered data preparation Tools can automatically clean and integrate this data, handling missing values, resolving inconsistencies, and ensuring data quality for accurate analysis.
4. Real-Time Analytics
AI algorithms can process vast amounts of data in real-time, providing instantaneous insights. This capability is crucial for industries where timely decision-making is vital:
- fraud detection: Identifying and preventing fraudulent transactions as they occur.
- Operational Monitoring: Tracking key performance indicators (KPIs) in real-time to optimize operations.
- customer personalization: Delivering personalized recommendations or offers based on real-time customer behavior.
Example: An e-commerce platform uses real-time analytics to monitor user activity and provide personalized product recommendations. As a customer browses the site, AI algorithms analyze their behavior, such as clicked products and time spent on pages, to suggest relevant items instantly.
5. Enhanced data Visualization
Advanced AI Techniques can create more intuitive and interactive visualizations, making complex data easier to understand:
- Dynamic Dashboards: Automatically updating visualizations based on real-time data.
- interactive Charts: Allowing users to explore data by drilling down into specific details or filtering results.
- AI-Generated Insights: Highlighting key trends, anomalies, or patterns in the data.
Example: A marketing agency uses AI-enhanced data visualization Tools to create interactive dashboards for their clients. These dashboards display campaign performance metrics, such as click-through rates and conversion rates, in real-time. Clients can interact with the visualizations, filtering data by date range, channel, or demographic segment to gain deeper insights.
Real-World applications
Several industries are already leveraging AI-enhanced BI to drive innovation and improve outcomes:
retail
- Inventory optimization: Predicting inventory needs based on sales trends, seasonal patterns, and external factors.
- customer segmentation: Identifying customer segments with similar behaviors or preferences for targeted marketing campaigns.
- Price optimization: Determining the optimal pricing strategy based on competitor analysis, demand elasticity, and customer willingness to pay.
Example: A large retail chain uses AI-powered BI Tools to optimize inventory levels across its stores. By analyzing sales data, weather patterns, and local events, the AI model can predict which products will be in high demand and adjust inventory accordingly. This reduces stockouts and excess inventory, leading to improved customer satisfaction and reduced Operational Costs.
Finance
- fraud detection: Identifying and preventing fraudulent transactions in real-time using anomaly detection algorithms.
- Credit Scoring: Assessing the creditworthiness of loan applicants based on a wide range of data points, including traditional credit history and alternative data sources.
- Algorithm Trading: Executing trades based on predefined rules and real-time market data to maximize profits and minimize risks.
Example: A bank implements an AI-driven fraud detection system that analyzes transaction patterns in real-time. The system can identify unusual or suspicious activities, such as unexpected large transactions or transactions from new locations, and flag them for further investigation. This helps the bank prevent fraudulent activities and protect its customers' assets.
healthcare
- Predictive Diagnostics: Analyzing patient data to predict disease outbreaks or personalize treatment plans.
- Operational efficiency: Optimizing resource allocation, such as staffing levels or equipment usage, based on patient demand and historical data.
- Population Health Management: Identifying at-risk populations and implementing Preventive Measures to improve overall health outcomes.
Example: A hospital uses AI-enhanced BI Tools to predict patient admissions and optimize bed management. By analyzing historical admission data, seasonality, and external factors like flu outbreaks, the AI model can forecast peak demand periods and allocate resources accordingly. This ensures that the hospital has sufficient capacity to handle patient influxes and provides better care.
manufacturing
- Supply Chain optimization: Improving Supply Chain efficiency by predicting demand, optimizing inventory levels, and identifying potential disruptions.
- predictive maintenance: Anticipating equipment failures before they occur using sensor data and Machine Learning algorithms.
- quality control: Detecting defects or anomalies in products during the manufacturing process to ensure high-quality standards.
Example: A manufacturing company uses AI-powered BI Tools to optimize its Supply Chain operations. By analyzing historical sales data, market trends, and supplier performance, the AI model can predict demand for raw materials and adjust procurement plans accordingly. This reduces lead times, minimizes stockouts, and improves overall Supply Chain efficiency.
Implementing AI in Business Intelligence
To successfully integrate AI into your BI strategy, consider the following steps:
1. Identify Key Use Cases
Determine where AI can provide the most significant impact within your organization. This could involve improving specific processes, Enhancing decision-making, or driving innovation. Conduct a thorough analysis of your current BI capabilities and identify gaps that AI can address.
Example: A telecommunications company identifies customer churn as a critical area for improvement. By implementing AI-driven predictive analytics, the company can analyze customer behavior data to identify at-risk customers and implement targeted retention strategies.
2. Invest in technology
Choose AI Tools and platforms that align with your business goals and existing infrastructure. Consider factors such as scalability, integration capabilities, and ease of use when selecting technologies.
Example: A financial services firm invests in an AI-powered data preparation tool to streamline its ETL processes. The tool automates data cleaning, integration, and transformation tasks, reducing manual effort and improving data quality.
3. data Quality Management
Ensure your data is clean, well-organized, and reliable for effective AI analysis. Implement data governance practices, such as data validation, standardization, and documentation, to maintain high data quality standards.
Example: A healthcare organization establishes a data governance framework to ensure the accuracy and reliability of its patient data. This includes regular data audits, validation checks, and documentation of data sources and transformation processes.
4. Skilled Workforce
Train your team to leverage AI Tools effectively. Provide opportunities for continuous learning and development, such as workshops, online courses, or certifications, to build AI expertise within your organization.
Example: A retail company offers training programs on AI and Machine Learning Techniques to its data analysts and business intelligence professionals. This enables them to develop and deploy AI-driven BI solutions that drive business value.
The Future of Business Intelligence
As AI continues to evolve, its role in Enhancing BI will only grow stronger. Organizations that embrace this technology will be better equipped to navigate the complexities of the modern business landscape and gain a competitive advantage. Some emerging trends in AI-enhanced BI include:
- Explainable AI (XAI): Making AI models more transparent and interpretable, allowing users to understand how predictions are made.
- AutoML: Automating the Machine Learning process to make IT more accessible to non-experts.
- Edge computing: Processing data closer to its source, reducing latency and enabling real-time analytics in distributed environments.
Integrating artificial intelligence into business intelligence strategies can unlock new levels of insight and efficiency. By leveraging AI-driven predictive analytics, NLP, automated data preparation, real-time analytics, and enhanced visualization, businesses can make more informed decisions faster than ever before. As AI technology continues to advance, its impact on BI will only deepen, driving innovation and creating new opportunities for organizations to thrive in an increasingly data-driven world.
By following best practices for implementation and staying abreast of emerging trends, businesses can harness the full potential of AI-enhanced BI to achieve their strategic goals and gain a competitive edge. Embracing this transformative technology is not just an option but a necessity for organizations seeking to stay ahead in the rapidly evolving business landscape.