Challenges in AI Deployment and How to Overcome Them
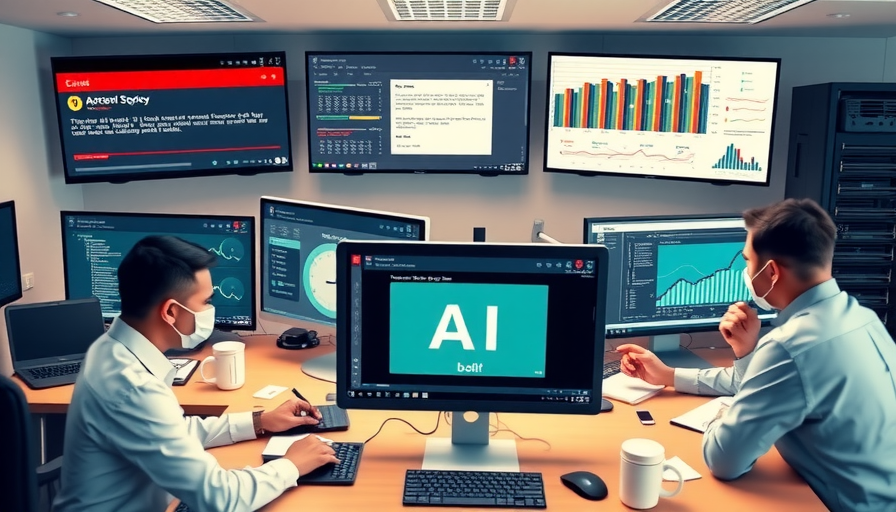
Artificial Intelligence (AI) has revolutionized various industries by offering unprecedented capabilities in data analysis, automation, and decision-making. However, deploying AI solutions is not without its challenges. The journey from developing an AI model to successfully deploying IT in a real-world Environment can be fraught with difficulties. In this comprehensive blog post, we will delve into the top obstacles faced during AI deployment and provide actionable strategies to overcome them.
Understanding AI Deployment challenges
AI deployment involves integrating AI models into production environments where they can interact with real-world data and systems. This process is complex and multifaceted, encompassing technical, organizational, and ethical dimensions. Some of the most common AI deployment challenges include:
- data Quality Issues: Poor quality data can significantly impact the performance and accuracy of AI models.
- Scalability Concerns: Ensuring that your AI solution can handle increasing amounts of data without compromising performance.
- integration Problems: Seamlessly integrating AI into existing systems and workflows.
- Regulatory compliance: Adhering to legal and regulatory standards, especially in sensitive industries like healthcare and finance.
- Ethical Considerations: Ensuring that AI solutions are fair, transparent, and unbiased.
- Resource Constraints: Managing computational resources and infrastructure effectively.
- Model Drift: Handling the deterioration of model performance over time due to changing data patterns.
Let's explore each of these challenges in detail and discuss strategies to overcome them.
1. Improving data Quality
The Importance of data Quality
data is the lifeblood of AI models. Poor quality data can lead to inaccurate predictions, biased outcomes, and ultimately, failed AI deployments. Ensuring high-quality data is crucial for building reliable and effective AI solutions.
Common data Quality Issues
- Missing Values: Incomplete datasets where certain values are missing.
- Inconsistent data: data that is not standardized, leading to discrepancies.
- Outliers: Extreme values that can skew model performance.
- Noise: Irrelevant or incorrect data points that affect model accuracy.
Strategies for Improving data Quality
-
data Cleaning: Implement robust data cleaning processes to handle missing values, inconsistencies, and outliers. Tools like Pandas in Python can be very helpful for this purpose.
import pandas as pd # Example of handling missing values df = pd.read_csv('[data](https://www.ai-infra-link.com/smart-grids-the-intersection-of-it-engineering-and-energy-management/).csv') df.fillna(method='ffill', inplace=True) # Forward fill to handle missing values
-
data Validation: Use data validation Techniques to ensure that the data meets predefined criteria. This can include checks for data types, ranges, and formats.
-
Automated data Quality Tools: Leverage Tools like Great Expectations or Talend to automate data quality checks and ensure consistency.
-
Regular Audits: Conduct regular data audits to identify and rectify data quality issues proactively.
Example: data Cleaning in a healthcare Setting
In a healthcare setting, patient records may contain missing or inconsistent data. For example, some records might have missing blood pressure readings, while others might have inconsistent date formats. By implementing data cleaning processes, you can fill in missing values using appropriate imputation Techniques and standardize date formats to ensure consistency.
2. Scaling AI solutions
The Need for Scalability
As AI solutions handle increasingly large datasets and user bases, Scalability becomes a critical concern. Ensuring that your AI solution can scale effectively is essential for maintaining performance and reliability.
Common Scalability challenges
- Resource Limitations: Insufficient computational resources to handle large-scale data processing.
- latency Issues: Increased latency as the system struggles to process data in real-time.
- Cost management: High costs associated with scaling infrastructure.
Strategies for Scaling AI solutions
-
Cloud-Based solutions: Use cloud platforms like AWS, Azure, or Google Cloud to leverage scalable infrastructure. These platforms offer flexible resource allocation and can handle large-scale data processing efficiently.
-
containerization and Orchestration: Adopt containerization with Docker and orchestration with Kubernetes to ensure that your AI solutions can scale seamlessly. containers allow you to package applications and their dependencies, making IT easier to deploy and scale them across different environments.
# Example [Kubernetes](https://www.ai-infra-link.com/the-ever-growing-impact-of-kubernetes-in-cloud-computing/) deployment configuration apiVersion: apps/v1 kind: Deployment metadata: name: [AI](https://www.ai-infra-link.com/ai-and-sustainability-how-tech-can-help-fight-climate-change/)-model-deployment spec: replicas: 3 selector: matchLabels: app: [AI](https://www.ai-infra-link.com/ai-and-sustainability-how-tech-can-help-fight-climate-change/)-model template: metadata: labels: app: [AI](https://www.ai-infra-link.com/ai-and-sustainability-how-tech-can-help-fight-climate-change/)-model spec: [containers](https://www.ai-infra-link.com/building-scalable-it-solutions-with-cloud-native-technologies/): - name: [AI](https://www.ai-infra-link.com/ai-and-sustainability-how-tech-can-help-fight-climate-change/)-model-container image: [AI](https://www.ai-infra-link.com/ai-and-sustainability-how-tech-can-help-fight-climate-change/)-model-image:latest ports: - containerPort: 80
-
Horizontal and Vertical Scaling: Implement horizontal scaling by adding more instances of your AI solution to distribute the load. Vertical scaling involves upgrading the hardware resources of existing instances.
-
load balancing: Use load balancers to distribute incoming traffic evenly across multiple instances of your AI solution, ensuring optimal performance and reliability.
Example: Scaling an e-commerce Recommendation System
In an e-commerce setting, a recommendation system might need to handle millions of user interactions daily. By using cloud-based solutions and containerization, you can ensure that the system can scale seamlessly to meet Increasing Demand. Load balancers can distribute traffic across multiple instances, ensuring fast response times and reliable performance.
3. Seamless integration
The Importance of integration
Seamless integration is crucial for the successful deployment of AI solutions. AI models need to work harmoniously with existing systems and workflows to provide value and drive Innovation.
Common integration challenges
- Legacy Systems: Integrating AI with legacy systems that were not designed with modern AI capabilities in mind.
- data Silos: Isolated data sources that make IT difficult to integrate AI solutions effectively.
- API Compatibility: Ensuring that APIs used for integration are compatible and secure.
Strategies for Seamless integration
-
APIs (Application Programming Interfaces): Use APIs to enable seamless communication between different software components. RESTful APIs and GraphQL are popular choices for integrating AI solutions with existing systems.
import requests # Example of using a RESTful API to integrate an [AI](https://www.ai-infra-link.com/ai-and-sustainability-how-tech-can-help-fight-climate-change/) model response = requests.post('http://api.example.com/predict', json=[data](https://www.ai-infra-link.com/smart-grids-the-intersection-of-it-engineering-and-energy-management/)) [prediction](https://www.ai-infra-link.com/harnessing-the-power-of-ai-10-ways-ai-can-improve-infrastructure-for-disaster-risk-reduction-2/) = response.json()
-
Middleware solutions: Implement middleware solutions like Apache Kafka or RabbitMQ to facilitate data exchange between different systems.
-
data Warehousing: Use data warehousing solutions like Amazon Redshift or Google BigQuery to centralize data and break down silos, making IT easier to integrate AI solutions.
-
microservices architecture: Adopt a microservices architecture to build modular and scalable AI solutions that can be easily integrated with existing systems.
Example: Integrating an AI-Powered Chatbot
In a customer service setting, integrating an AI-powered chatbot with existing CRM (Customer Relationship Management) systems is essential for providing seamless support. By using APIs, you can enable the chatbot to access customer data and provide personalized responses. Middleware solutions can facilitate real-time data exchange between the chatbot and CRM systems.
4. Ensuring Regulatory compliance
The Need for Regulatory compliance
Adhering to legal and regulatory standards is crucial, especially in sensitive industries like healthcare, finance, and telecommunications. Non-compliance can result in hefty fines, reputational damage, and legal action.
Common Regulatory challenges
- data privacy: Ensuring that AI solutions comply with data privacy Regulations like GDPR (General data Protection regulation) or CCPA (California Consumer privacy Act).
- Industry-Specific Regulations: Adhering to industry-specific Regulations like HIPAA (Health Insurance Portability and accountability Act) in healthcare.
- bias and fairness: Ensuring that AI solutions do not perpetuate bias or discrimination.
Strategies for Ensuring Regulatory compliance
-
Automated Compliance Tools: Use Automated Compliance Tools to monitor and ensure adherence to regulatory standards. Tools like OneTrust or BigID can help automate data privacy compliance.
-
Regular Audits: Conduct regular audits to identify and rectify compliance issues proactively. Engage with legal and compliance experts to stay informed about evolving Regulations.
-
data Anonymization: Implement data Anonymization Techniques to protect sensitive information and comply with data privacy Regulations.
from faker import Faker fake = Faker() # Example of [data](https://www.ai-infra-link.com/smart-grids-the-intersection-of-it-engineering-and-energy-management/) [Anonymization](https://www.ai-infra-link.com/ai-and-personal-data-protection-tools-and-techniques/) def anonymize_data([data](https://www.ai-infra-link.com/smart-grids-the-intersection-of-it-engineering-and-energy-management/)): for entry in [data](https://www.ai-infra-link.com/smart-grids-the-intersection-of-it-engineering-and-energy-management/): entry['name'] = fake.name() entry['email'] = fake.email() return [data](https://www.ai-infra-link.com/smart-grids-the-intersection-of-it-engineering-and-energy-management/)
-
bias audits: Conduct regular bias audits to ensure that AI solutions are fair and unbiased. Use Tools like IBM's AI fairness 360 to detect and mitigate bias in AI models.
Example: Ensuring compliance in a Financial Institution
In a financial institution, ensuring compliance with Regulations like GDPR or CCPA is crucial for protecting customer data. By using Automated Compliance Tools and conducting regular audits, you can ensure that your AI solutions adhere to regulatory standards. data Anonymization Techniques can be used to protect sensitive information, while bias audits can help ensure fairness in AI-driven decision-making processes.
5. Ethical AI Deployment
The Importance of Ethical AI
Ensuring that AI solutions are fair, transparent, and unbiased is essential for building trust and driving adoption. Ethical considerations are critical for the responsible deployment of AI.
Common Ethical challenges
- Bias: AI models can inadvertently perpetuate or amplify existing biases in data.
- transparency: Lack of transparency in AI decision-making processes can erode trust.
- accountability: Ensuring that there is accountability for AI-driven decisions and actions.
Strategies for Ethical AI Deployment
-
fairness, accountability, and transparency (FAccT): Promote ethical AI practices by incorporating FAccT principles into your AI development and deployment processes. Use explainable AI Techniques to make AI decision-making more transparent.
-
Bias Mitigation: Implement bias mitigation Techniques like re-sampling, re-weighting, or using fairness-aware algorithms to reduce bias in AI models.
from aif360.datasets import StandardDataset # Example of using AIF 360 to detect and mitigate bias dataset = StandardDataset(predicted_values=y_pred, label_names=['label'], favorable_label='favorable', unfavorable_label='unfavorable') bias_mitigator = BiasMitigator(dataset) bias_mitigator.fit()
-
ethical guidelines: Develop and adhere to ethical guidelines for AI development and deployment. Engage with stakeholders to ensure that ethical considerations are prioritized.
-
Regular Audits: Conduct regular audits to identify and rectify ethical issues proactively. Use Tools like IBM's AI Explainability 360 to explain AI decisions and build trust.
Example: Ethical Considerations in Hiring
In a hiring setting, ensuring that AI-driven recruitment processes are fair and unbiased is crucial for maintaining diversity and inclusion. By using bias mitigation Techniques and explainable AI, you can reduce bias in candidate screening and ensure transparency in decision-making processes. Regular audits can help identify and rectify ethical issues proactively.
6. Managing Resources Effectively
The Need for Resource Management
Managing computational resources and infrastructure effectively is essential for the successful deployment of AI solutions. Inefficient resource management can lead to increased costs, performance bottlenecks, and Scalability issues.
Common Resource Management challenges
- Computational Resources: Insufficient computational power to handle AI workloads.
- infrastructure Costs: High costs associated with maintaining and scaling infrastructure.
- energy consumption: Energy-intensive AI workloads can lead to increased carbon footprint.
Strategies for Effective Resource Management
-
Efficient Algorithms: Use efficient algorithms and optimization Techniques to reduce computational requirements. Techniques like model pruning, quantization, or knowledge distillation can help optimize AI models.
import [TensorFlow](https://www.ai-infra-link.com/the-evolution-of-ai-development-tools-and-frameworks/) as tf # Example of model quantization in [TensorFlow](https://www.ai-infra-link.com/the-evolution-of-ai-development-tools-and-frameworks/) converter = tf.lite.TFLiteConverter.from_saved_model('saved_model_dir') converter.optimizations = [tf.lite.Optimize.DEFAULT] tflite_model = converter.convert()
-
Hardware Acceleration: Leverage hardware acceleration Techniques like GPUs (Graphics Processing Units) or TPUs (Tensor Processing Units) to enhance computational performance.
-
Cloud-Based solutions: Use cloud platforms to leverage scalable and cost-effective infrastructure. cloud providers offer flexible resource allocation and can help optimize costs.
-
Monitoring and optimization: Implement monitoring Tools to track resource usage and identify optimization opportunities. Tools like Prometheus or Grafana can help monitor AI workloads and ensure optimal performance.
Example: Optimizing Resource Usage in a manufacturing Setting
In a manufacturing setting, optimizing resource usage is crucial for ensuring efficient operations. By using efficient algorithms and hardware acceleration, you can reduce computational requirements and enhance performance. Cloud-based solutions can provide scalable infrastructure, while monitoring Tools can help identify optimization opportunities and ensure optimal resource management.
7. Handling Model Drift
The Importance of Addressing Model Drift
Model drift refers to the deterioration of model performance over time due to changing data patterns. Addressing model drift is crucial for maintaining the accuracy and reliability of AI solutions.
Common Causes of Model Drift
- Concept Drift: Changes in the underlying relationships between input features and target variables.
- data Drift: Changes in the distribution of input data.
- Feedback Loops: AI-driven decisions that affect future data patterns, leading to drift.
Strategies for Handling Model Drift
-
continuous monitoring: Implement continuous monitoring protocols to detect model drift early. Use Tools like Evidently or Arize to monitor model performance and identify drift.
-
Automated Retraining: Set up automated retraining pipelines to update models regularly based on new data. Tools like MLflow or Kubeflow can help automate the retraining process.
import mlflow # Example of using MLflow for automated retraining with mlflow.start_run(): model = train_model(new_data) mlflow.log_param(param_name, param_value) mlflow.sklearn.log_model(model, model)
-
Drift Detection Tools: Use drift detection Tools to identify changes in data patterns and trigger retraining processes. Tools like AlgoSkeptic or DriftKit can help detect drift and ensure model reliability.
-
Regular Audits: Conduct regular audits to assess model performance and identify drift issues proactively. Engage with domain experts to stay informed about evolving data patterns.
Example: Handling Model Drift in a Financial Fraud Detection System
In a financial Fraud Detection system, handling model drift is crucial for maintaining accuracy and reliability. By implementing continuous monitoring and automated retraining protocols, you can detect drift early and update models regularly based on new data. Drift detection Tools can help identify changes in data patterns, while regular audits can ensure that the system remains accurate and reliable.
While AI deployment challenges are numerous and varied, with the right strategies and Tools, they can be effectively managed. By focusing on data quality, Scalability, integration, regulatory compliance, ethical considerations, resource management, and handling model drift, you can ensure a successful AI deployment that drives Innovation and efficiency.
In this comprehensive guide, we have explored the top obstacles faced during AI deployment and provided actionable strategies to overcome them. From improving data quality to ensuring ethical AI practices, each challenge requires a thoughtful approach and the use of appropriate Tools and Techniques.
As AI continues to evolve, staying informed about best practices and emerging technologies will be crucial for successful deployment. By prioritizing these key areas, you can build robust and reliable AI solutions that deliver value and drive Innovation in your industry.