What Will be the Next Big Innovations in AI Hardware
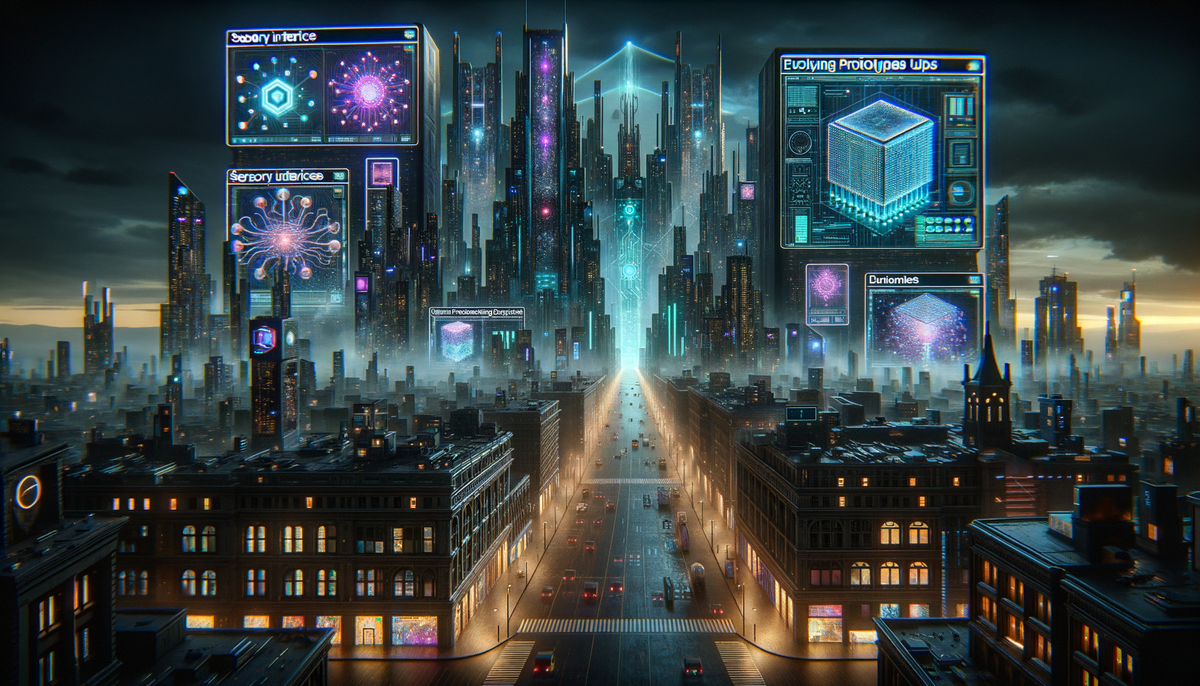
Artificial Intelligence (AI) has revolutionized various facets of technology and industry. As the demand for AI applications continues to surge, the need for more advanced AI hardware becomes increasingly critical. The race to develop innovative AI hardware is intensifying, and several key innovations are on the horizon that could shape the future landscape of AI. This blog post explores the next big innovations in AI hardware and their potential impact on the industry.
Quantum computing
Quantum computing is often hailed as the next frontier in AI hardware. quantum computers utilize the principles of quantum mechanics to process information at unprecedented speeds. Unlike classical computers, which rely on bits as the smallest unit of data, quantum computers use quantum bits, or qubits. This allows them to perform complex calculations much faster. Quantum AI hardware can significantly accelerate machine learning processes, enabling the solving of problems that were previously intractable.
Neuromorphic Engineering
Neuromorphic engineering is an exciting area that focuses on creating hardware inspired by the human brain's architecture. Neuromorphic chips mimic the Neural Networks of the brain, offering efficient processing power and energy consumption. These chips can lead to the development of more sophisticated AI systems capable of real-time learning and adaptation. Companies like Intel and IBM are leading the charge in developing neuromorphic hardware, which holds promise for Enhancing the efficiency and capabilities of AI systems.
Graph Processing Units (GPUs)
GPUs have been instrumental in the advancement of AI, especially in Deep Learning applications. However, the next generation of GPUs, with enhanced memory and parallel processing capabilities, promises even greater performance. Innovations like NVIDIA's Tensor Cores, which are specifically optimized for AI workloads, are pushing the boundaries of what GPUs can achieve. Future GPUs will likely offer even more specialized features for AI, making them essential for handling the ever-increasing demands of machine learning algorithms.
Application-Specific Integrated Circuits (ASICs)
Application-Specific Integrated Circuits (ASICs) are custom-designed chips optimized for specific tasks. In the context of AI, ASICs can be tailored to accelerate particular machine learning algorithms, offering superior performance compared to general-purpose hardware. Google's Tensor Processing Unit (TPU) is a prime example of an ASIC designed for AI workloads. The development of more specialized ASICs will pave the way for high-efficiency AI hardware, enabling faster and more accurate AI processing.
Field-Programmable Gate Arrays (FPGAs)
Field-Programmable Gate Arrays (FPGAs) offer a flexible and reconfigurable hardware option for AI applications. Unlike ASICs, which are fixed and cannot be altered post-manufacturing, FPGAs can be reprogrammed to suit different tasks. This adaptability makes them an excellent choice for AI research and development. FPGAs are also known for their high computational efficiency, making them suitable for various AI applications, from data centers to edge devices.
Photonic computing
Photonic computing is an emerging technology that uses light rather than electricity to perform computations. Photons, or light particles, can travel much faster than electrons, allowing photonic computers to process information at exceedingly high speeds. This technology has the potential to revolutionize AI hardware by enabling ultra-fast data processing and reducing energy consumption. While still in the experimental stage, photonic computing is gaining traction and could become a cornerstone of future AI systems.
Edge AI Hardware
Edge AI refers to processing data locally on edge devices rather than relying on centralized cloud servers. As the number of connected devices grows, the demand for efficient edge AI hardware increases. Innovations in edge AI hardware focus on developing low-power, high-performance chips capable of handling AI tasks directly on devices like smartphones, drones, and IoT sensors. Companies like ARM and NVIDIA are at the forefront of creating edge AI solutions that offer real-time processing with minimal latency.
Conclusion
The future of AI hardware is incredibly promising, with numerous innovations set to transform how AI systems are built and deployed. Quantum computing, neuromorphic Engineering, advanced GPUs, specialized ASICs, adaptable FPGAs, photonic computing, and edge AI hardware each offer unique advantages that will drive the next wave of AI advancements. As these technologies continue to evolve, they will unlock new possibilities and push the boundaries of what Artificial Intelligence can achieve.