AI in Manufacturing: From Design to Production Optimization
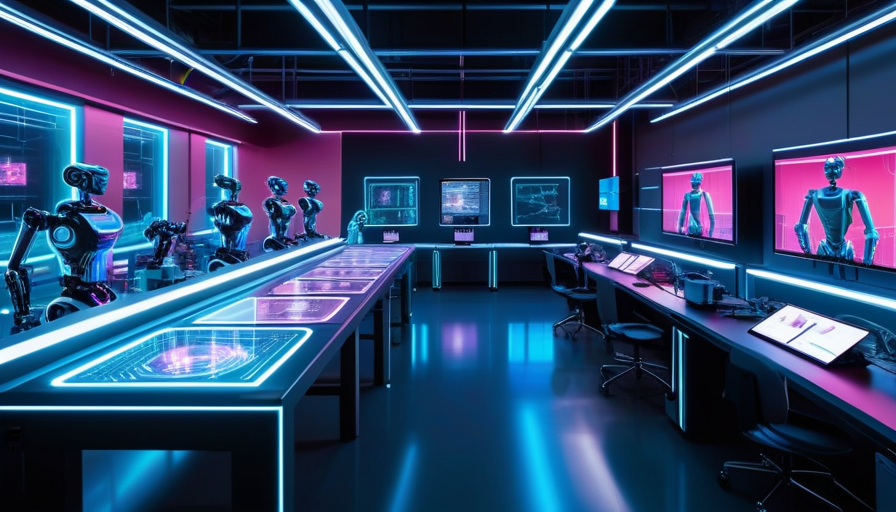
The integration of Artificial Intelligence (AI) into manufacturing is revolutionizing how products are designed, produced, and optimized. This transformative technology is Enhancing efficiency, reducing costs, and driving Innovation across the entire production lifecycle—from design through to optimization. In this comprehensive guide, we'll explore how AI impacts various stages of the manufacturing process, offering insights into its applications, benefits, and future potential.
The Evolution of manufacturing with AI
Historically, manufacturing has been a domain characterized by manual processes, extensive human intervention, and a significant focus on efficiency improvements through mechanization. However, as industries face increasing demands for customization, speed, and sustainability, traditional methods have proven inadequate in keeping up with these evolving needs. This is where AI enters the scene, offering intelligent solutions that promise to overhaul manufacturing paradigms.
The Shift Towards digital transformation
The Fourth Industrial Revolution (Industry 4.0) has brought about a seismic shift in manufacturing, driven by digital technologies such as AI, IoT, and big data analytics. These advancements have enabled manufacturers to transition from traditional, siloed operations to interconnected, data-driven ecosystems. At the heart of this transformation is AI, which provides the cognitive capabilities needed to analyze vast amounts of data, identify patterns, and make informed decisions in real-time.
The Role of AI in Modern manufacturing
AI's role in manufacturing extends across multiple dimensions, from Enhancing design processes to optimizing production workflows and Supply Chain management. By leveraging machine learning algorithms, Natural Language Processing (NLP), Computer Vision, and robotics, manufacturers can achieve unprecedented levels of Precision, efficiency, and adaptability.
Designing with AI
The design phase of manufacturing is witnessing significant advancements thanks to AI technologies like generative design and predictive analytics. These Tools enable designers to create complex models faster than ever before, optimizing for various parameters such as material usage, structural integrity, and aesthetic appeal.
Generative design
Generative design uses algorithms to generate numerous design alternatives based on specific constraints and desired outcomes. For instance, an automotive manufacturer might leverage generative design to develop a new vehicle chassis that meets stringent safety standards while minimizing weight and material costs. By analyzing thousands of potential designs in mere hours—a task that would take human engineers weeks or even months—generative design accelerates the Innovation process and opens up new possibilities for optimization.
Case Study: Airbus and Generative design
Airbus, a leading aerospace company, has successfully implemented generative design to optimize the structure of its aircraft components. By using AI algorithms to explore multiple design iterations, Airbus was able to reduce the weight of certain parts by up to 40%, resulting in significant fuel savings and reduced carbon emissions.
predictive analytics
predictive analytics involves using historical data and machine learning models to forecast future trends and outcomes. In the context of manufacturing design, predictive analytics can help identify potential issues early in the development process, allowing designers to make proactive adjustments and avoid costly redesigns later on.
applications in Product Development
- Failure prediction: Predictive Models can analyze design parameters and historical failure data to anticipate potential points of weakness or failure in a product. This enables engineers to reinforce critical areas and enhance overall durability.
- Performance optimization: By simulating various operating conditions, predictive analytics can help optimize product performance under different scenarios, ensuring that designs meet required specifications across a range of environments.
Computer-Aided design (CAD) integration
AI is also Enhancing traditional CAD Tools by incorporating machine learning capabilities. These advanced CAD systems can learn from user behavior and design patterns to provide intelligent suggestions, automate repetitive tasks, and improve overall design efficiency.
Benefits of AI-Enhanced CAD
- Automated Drafting: AI-powered CAD Tools can automatically generate drafts based on initial sketches or verbal descriptions, reducing the time required for manual drafting.
- design Recommendations: By analyzing successful designs from similar projects, AI can offer recommendations for improving current designs, such as suggesting alternative materials or structural modifications.
Optimizing Production with AI
Once a design is finalized, AI plays a crucial role in optimizing production processes to ensure efficiency, quality, and consistency. From automated assembly lines to predictive maintenance, AI-driven solutions are transforming the factory floor.
Automated quality control
AI-powered vision systems and sensors can perform real-time inspections of products as they move along the assembly line. These systems use Computer Vision algorithms to detect defects, measure dimensions, and ensure compliance with quality standards.
Advantages of Automated quality control
- Consistency: AI-driven quality control ensures consistent inspection standards, reducing human error and variability.
- Speed: Automated inspections can be performed at high speeds, keeping pace with fast-moving assembly lines without compromising accuracy.
- data Collection: AI systems can collect detailed data on product quality, enabling manufacturers to identify trends, track performance metrics, and make data-driven improvements.
predictive maintenance
predictive maintenance uses machine learning algorithms to analyze sensor data from machinery and equipment, predicting when failures are likely to occur. This Proactive approach allows manufacturers to schedule maintenance activities before breakdowns happen, minimizing downtime and reducing repair costs.
Implementing predictive maintenance
- Sensor integration: Equip machinery with sensors that collect data on temperature, vibration, pressure, and other critical parameters.
- data analysis: Use machine learning models to analyze sensor data in real-time, identifying anomalies and predicting potential failures.
- Maintenance Scheduling: Based on predictive insights, schedule maintenance activities during planned downtimes or when the impact on production is minimal.
Robotic Process automation (RPA)
Robotic process automation involves using software robots to automate repetitive tasks, freeing up human workers for more complex and strategic activities. In manufacturing, RPA can be applied to various processes, from inventory management to order processing and Supply Chain coordination.
applications of RPA in manufacturing
- inventory management: automate the tracking of raw materials and finished goods, ensuring accurate stock levels and reducing the risk of shortages or excess inventory.
- Order Processing: Streamline order entry, fulfillment, and shipping processes by automating data entry, validation, and communication with suppliers and customers.
Smart Manufacturing and IoT
The Internet of Things (IoT) enables the interconnection of physical devices, sensors, and machinery, creating a networked ecosystem where data can be collected, analyzed, and acted upon in real-time. When combined with AI, IoT drives smart manufacturing initiatives that enhance operational efficiency, flexibility, and responsiveness.
Key Components of Smart manufacturing
- Connected Devices: Equip machinery, equipment, and Tools with sensors and communication capabilities to collect and transmit data.
- data Analytics: Use AI algorithms to analyze real-time data from connected devices, identifying patterns, optimizing processes, and making informed decisions.
- Automated Control Systems: Implement automated control systems that adjust production parameters based on real-time data insights, ensuring optimal performance and efficiency.
Enhancing Supply Chain Management with AI
AI's impact extends beyond the factory floor to encompass the entire Supply Chain, from procurement and logistics to distribution and customer service. By leveraging AI-driven solutions, manufacturers can achieve greater visibility, agility, and resilience in their supply chains.
demand forecasting
Accurate demand forecasting is crucial for optimizing inventory levels, reducing stockouts, and minimizing excess inventory. AI-powered demand forecasting models use historical sales data, market trends, and external factors to predict future demand with high accuracy.
Benefits of AI-Driven demand forecasting
- Improved inventory management: Accurately forecasted demand enables manufacturers to maintain optimal inventory levels, reducing holding costs and improving cash flow.
- Enhanced customer satisfaction: By ensuring product availability and minimizing stockouts, manufacturers can meet customer expectations and enhance satisfaction.
logistics optimization
AI can optimize logistics operations by analyzing Transportation routes, carrier performance, and delivery schedules. This enables manufacturers to reduce shipping costs, improve on-time delivery, and enhance overall Supply Chain efficiency.
applications of AI in Logistics
- Route Planning: Use AI algorithms to determine the most efficient routes for transporting goods, considering factors such as distance, traffic, weather, and fuel consumption.
- Carrier Selection: Analyze carrier performance data to select the most reliable and cost-effective Transportation providers for each shipment.
Supplier Relationship Management
AI-driven solutions can enhance supplier relationship management by providing insights into supplier performance, risk assessment, and Collaboration opportunities. This enables manufacturers to build stronger, more resilient supply chains that are better equipped to handle disruptions and changes in demand.
Leveraging AI for supplier management
- performance monitoring: Use AI algorithms to analyze supplier performance data, identifying trends, strengths, and areas for improvement.
- risk assessment: Assess the risk associated with each supplier based on factors such as financial stability, compliance, and operational capabilities. This enables manufacturers to diversify their supply base and mitigate risks.
Improving customer service with AI
AI is transforming customer service in manufacturing by enabling personalized interactions, faster response times, and proactive issue resolution. From chatbots to virtual assistants, AI-driven solutions are Enhancing the customer experience at every touchpoint.
chatbots and virtual assistants
chatbots and virtual assistants use Natural Language Processing (NLP) to understand and respond to customer inquiries in real-time. These AI-powered Tools can handle a wide range of customer service tasks, from answering FAQs to providing product recommendations and troubleshooting technical issues.
Benefits of AI-Powered customer service
- 24/7 Availability: chatbots and virtual assistants can provide round-the-clock support, ensuring that customers receive assistance whenever they need IT.
- Consistency: AI-driven customer service Tools deliver consistent responses, reducing variability and improving the overall quality of interactions.
personalized recommendations
AI algorithms can analyze customer data to provide personalized product recommendations, Enhancing the shopping experience and driving sales. By understanding individual preferences and behaviors, manufacturers can offer tailored suggestions that meet each customer's unique needs.
Implementing personalized recommendations
- data Collection: Collect customer data from various sources, including purchase history, browsing behavior, and demographic information.
- AI analysis: Use machine learning models to analyze customer data and identify patterns, preferences, and trends.
- Recommendation Engine: Develop a recommendation engine that uses AI insights to provide personalized product suggestions.
Predictive customer support
Predictive customer support involves using AI algorithms to anticipate customer needs and proactively address potential issues before they arise. This Proactive approach enhances the customer experience by minimizing disruptions and ensuring timely resolution of problems.
applications of Predictive customer support
- Issue Detection: Use machine learning models to analyze customer data, identifying patterns that indicate potential issues or dissatisfaction.
- Proactive Resolution: Based on predictive insights, take proactive measures to address potential problems before they impact the customer experience.
Ethical Considerations and challenges in AI for manufacturing
While AI offers numerous benefits for manufacturing, IT also presents ethical considerations and challenges that must be addressed to ensure responsible implementation. These include data privacy, job displacement, and algorithmic bias.
data privacy and security
AI systems rely on large volumes of data to function effectively, raising concerns about data privacy and security. Manufacturers must implement robust measures to protect sensitive information and comply with regulatory requirements.
best practices for data Protection
- data encryption: Use encryption Techniques to secure data at rest and in transit, preventing unauthorized access.
- access control: Implement strict access controls to ensure that only authorized personnel can access sensitive data.
- compliance: Adhere to relevant data protection Regulations, such as GDPR or CCPA, to safeguard customer and employee information.
job displacement
The automation of manufacturing processes through AI raises concerns about job displacement. Manufacturers must address these challenges by investing in reskilling programs, fostering a culture of continuous learning, and promoting ethical AI practices that prioritize human well-being.
Strategies for Addressing job displacement
- Reskilling Programs: Provide training and development opportunities to help employees acquire new skills and adapt to changing job requirements.
- Ethical AI Practices: Implement AI solutions that augment rather than replace human capabilities, ensuring a balanced approach to automation.
algorithmic bias
AI algorithms can inadvertently perpetuate biases present in the data they are trained on, leading to unfair outcomes. Manufacturers must be vigilant about identifying and mitigating algorithmic bias to ensure equitable treatment of all stakeholders.
Mitigating algorithmic bias
- Diverse data Sets: Use diverse and representative data sets to train AI models, reducing the risk of bias.
- Bias Detection Tools: Employ Tools and Techniques for detecting and addressing biases in AI algorithms.
AI is revolutionizing manufacturing by Enhancing efficiency, improving product quality, and driving Innovation. From predictive maintenance to personalized customer service, AI offers a wide range of applications that can transform every aspect of the manufacturing process. However, manufacturers must also address ethical considerations and challenges to ensure responsible implementation. By embracing AI with a focus on ethics, data protection, and human well-being, manufacturers can unlock new opportunities for growth and success in an increasingly competitive landscape.