AI in Fraud Prevention: Transforming Financial Security
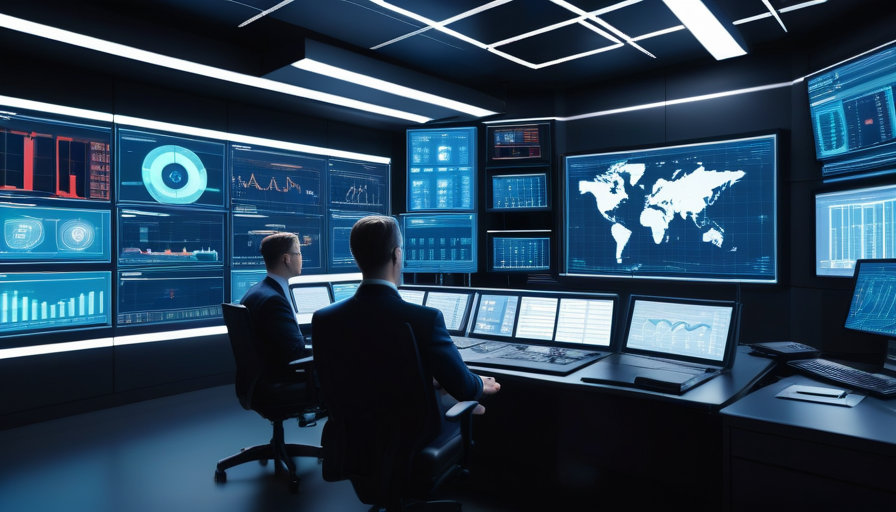
In recent years, Artificial Intelligence (AI) has become an integral part of financial services, revolutionizing how fraud is detected, prevented, and mitigated. The rise of AI-driven technologies has not only enhanced the accuracy and efficiency of Fraud Detection systems but also enabled organizations to anticipate fraudulent activities before they occur. This blog post explores the role of AI in fraud prevention within financial services, highlighting its benefits, key applications, and future potential.
Understanding fraud prevention in financial services
Fraud is a persistent challenge for financial institutions worldwide, as IT undermines trust, erodes revenue, and can lead to severe legal consequences. Traditional Fraud Detection methods rely on manual checks, rule-based systems, and statistical models. While these approaches have worked to some extent, they are often insufficient to combat the growing sophistication of fraudulent activities.
AI offers a more advanced solution by leveraging machine learning algorithms, Natural Language Processing, and pattern recognition Techniques. These technologies enable financial institutions to analyze vast amounts of data in real-time, identify suspicious patterns, and respond swiftly to potential threats. With AI-powered Fraud Detection systems, organizations can not only prevent fraud but also address IT proactively before IT escalates.
AI for Fraud Detection: A Closer Look
AI has become the backbone of modern Fraud Detection systems, particularly at the transactional level. Traditional Fraud Detection methods often rely on predefined rules or thresholds set by experts. However, these approaches are limited in their ability to adapt to evolving threats and can miss emerging patterns that require immediate attention.
AI-driven Fraud Detection systems, on the other hand, use machine learning algorithms to analyze historical data and identify patterns indicative of fraudulent activity. For instance, Supervised Learning models can be trained on datasets containing both fraudulent and legitimate transactions to classify new transactions accurately. Techniques such as decision trees, support vector machines (SVMs), and Neural Networks are commonly employed for this purpose.
One of the key advantages of AI in Fraud Detection is its ability to handle large volumes of data with Precision and speed. Traditional manual methods become inefficient when dealing with millions of transactions daily, whereas AI systems can process such data in real-time, ensuring prompt resolution of suspicious activities.
Moreover, AI systems are capable of detecting anomalies that might go unnoticed by human analysts. For example, Clustering Algorithms can group similar transaction patterns together, making IT easier to spot outliers that could indicate fraud. Advanced Techniques like Deep Learning and Natural Language Processing (NLP) enable systems to analyze unstructured data, such as conversation transcripts or emails, providing a more comprehensive view of potential threats.
AI in Behavioral analysis for fraud prevention
AI has also been instrumental in Enhancing behavioral analysis for fraud prevention in financial services. By analyzing customer behavior patterns, AI-powered systems can identify red flags that may indicate fraudulent intent. For instance, unusual login attempts, frequent account sign-ins from multiple devices, or rapid changes in spending habits could be signs of compromised credentials or identity theft.
AI-driven behavioral analysis often employs Deep Learning models to understand user behavior at a granular level. These models can learn the normal patterns of customer interactions and flag deviations as potential threats. For example, automated chatbots used for customer service can monitor conversation history and detect inconsistencies in customer inquiries, which could be a sign of fraudulent activity.
Moreover, AI systems can analyze transaction histories to identify recurring patterns that are suspicious. For instance, multiple large deposits or withdrawals from an account with minimal transactions could raise flags for further investigation. Advanced Algorithms can also predict the likelihood of fraud based on historical data, enabling organizations to allocate resources more effectively in prevention efforts.
AI for compliance and Regulatory Adherence
compliance is a critical aspect of fraud prevention in financial services, as adherence to regulatory requirements ensures transparency and accountability. AI plays a pivotal role in automating compliance checks, monitoring transactions for adherence to anti-money laundering (AML) and know-your-customer (KYC) Regulations.
AI-powered systems can flag suspicious activities that may violate regulatory constraints, such as multiple large transactions from a single source or unusual account creation patterns. These systems also help organizations maintain records of all transactions in compliance with AML/KYC requirements, making IT easier to justify actions during audits.
Furthermore, AI-driven chatbots and automated customer service platforms can assist in addressing potential compliance risks proactively. For example, chatbots can educate customers about anti-fraud measures or answer common questions related to account usage, reducing the likelihood of misuse.
AI for predictive analytics: risk assessment and fraud prevention
AI has also been embraced by financial institutions for predictive analytics, enabling them to assess credit risk, detect high-risk customers, and prevent fraudulent activities proactively. machine learning algorithms can analyze vast datasets, including customer demographics, transaction history, and economic indicators, to predict the likelihood of default or fraud.
For instance, AI-driven credit scoring models use historical data to evaluate the creditworthiness of individuals and organizations. These models incorporate machine learning Techniques such as random forests and gradient boosting to ensure high accuracy and reduce biases in decision-making. predictive analytics can also help detect early signs of potential fraud by analyzing trends in customer behavior or market conditions.
AI-powered risk assessment Tools go beyond credit scoring, offering insights into operational risks associated with each transaction or account. These systems use Natural Language Processing (NLP) to analyze unstructured data, such as news articles and regulatory updates, providing a holistic view of potential risks and enabling proactive measures to mitigate them.
AI for Automated Dispute resolution
In the event of disputes between customers or institutions, AI can play a crucial role in resolving conflicts efficiently and fairly. AI-driven chatbots and automated resolution systems can handle routine inquiries, complaints, and disputes, reducing the workload on human staff and ensuring timely resolutions.
Moreover, AI-powered chatbots can be trained to recognize common dispute patterns and provide tailored solutions based on historical data. For example, a customer service bot equipped with NLP capabilities can analyze a customer's complaint and suggest potential resolutions or recommend further actions based on similar cases resolved in the past.
AI can also assist in verifying customer identities during disputes, ensuring that only legitimate users are engaged. By integrating Fraud Detection mechanisms into automated Dispute resolution systems, organizations can minimize the risk ofFraudulent claims while maintaining trust with their customers.
The Future of AI in Fraud Prevention
As AI technology continues to evolve, its role in fraud prevention is poised for further growth. Upcoming advancements in Deep Learning, quantum computing, and edge computing will enable even more sophisticated Fraud Detection systems, capable of processing data at unprecedented speeds and scales. These innovations promise to enhance the accuracy and efficiency of fraud prevention mechanisms, ensuring that financial institutions remain one step ahead of potential threats.
Moreover, AI's ability to integrate with Blockchain technology is expected to revolutionize the future of fraud prevention. By combining the immutable nature of Blockchain with AI-driven analytics, organizations can create robust systems for verifying transactions and maintaining transparency in financial processes. This integration will likely enhance trust and security in the financial ecosystem, fostering a more resilient and secure digital Environment.
challenges and Considerations
Despite its immense potential, the adoption of AI in fraud prevention comes with challenges that must be carefully addressed. One major concern is data privacy and security, as organizations collect vast amounts of customer data for training and operational purposes. Ensuring compliance with Regulations such as GDPR and CCPA while maintaining high levels of data security will be a significant challenge.
Another critical issue is the potential for AI-driven systems to introduce biases or errors in their decision-making processes. Organizations must adopt rigorous quality control measures, including testing and validation protocols, to ensure that AI systems are accurate, fair, and transparent.
Additionally, ethical considerations must be addressed to prevent the misuse of AI-powered Fraud Detection mechanisms. For example, there is a risk that organizations could use these systems to target vulnerable customers or manipulate their decision-making processes for personal gain. Organizations must establish clear guidelines and oversight mechanisms to ensure responsible usage of AI in fraud prevention.