10 Use Cases for AI Innovations and Applications in Biotechnology
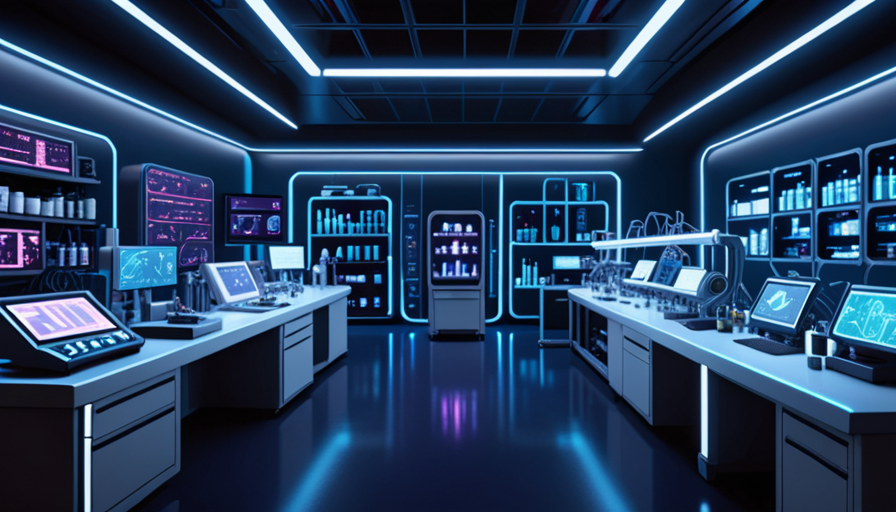
Artificial Intelligence (AI) has revolutionized numerous industries, with Biotechnology being one of the most transformative fields benefiting from these advancements. The integration of AI into biotech is not just Enhancing existing processes but also opening up new avenues for Innovation and discovery. This blog post explores ten significant use cases where AI is making an impact in Biotechnology, driving innovations and applications that promise to reshape our understanding of life sciences.
1. drug discovery and Development
AI has significantly accelerated the drug discovery process, which traditionally takes years and requires substantial financial investment. machine learning algorithms can analyze vast datasets to identify potential drug candidates much faster than traditional methods. Companies like Atomwise use AI to predict how different molecules will bind to target proteins, drastically reducing the time needed to find promising compounds.
How AI Accelerates drug discovery
- Molecular simulation: AI models simulate molecular interactions at an atomic level, predicting how a compound will behave in the body. This helps in identifying potential drug candidates that are likely to be effective and safe.
- data integration: AI can integrate data from various sources, including genomic information, proteomic data, and clinical trial results, to provide a comprehensive view of potential drug targets.
- predictive analytics: machine learning algorithms analyze historical data on drug failures and successes to predict the likelihood of a new compound being effective.
Case Study: Insilico Medicine
Insilico Medicine uses AI to discover novel drugs for aging-related diseases. Their platform, Pharma.AI, integrates Deep Learning with Reinforcement Learning to identify potential drug candidates and optimize their properties. This approach has led to the discovery of several promising compounds that are currently in preclinical development.
Benefits of AI in drug discovery
- Reduced Costs: By identifying promising compounds earlier in the process, AI can significantly reduce the financial burden associated with drug development.
- Faster Time-to-Market: AI-driven insights accelerate the drug discovery pipeline, allowing new therapies to reach patients more quickly.
- Improved Success Rates: predictive analytics and molecular Simulations enhance the likelihood of identifying effective drugs, reducing the risk of late-stage failures.
2. Genomic analysis
genomics involves studying organisms' complete set of DNA, including all of its genes. Analyzing genomic data can be overwhelming due to its complexity and volume. AI algorithms excel at identifying patterns in large datasets, making them invaluable Tools in genomics. Tools like DeepVariant from Google use Deep Learning to improve the accuracy of genetic variant calling, which is crucial for understanding genetic diseases.
applications of AI in genomics
- Genetic Variant Identification: AI models can identify rare and common genetic variants that may be associated with specific diseases or traits.
- Functional Annotation: machine learning algorithms help annotate genomic regions to understand their functional significance, aiding in the identification of potential Therapeutic Targets.
- personalized medicine: AI analyzes individual genomic data to tailor treatments specifically suited to a patient's genetic makeup, ensuring better outcomes and fewer side effects.
Case Study: 23andMe
23andMe uses AI to analyze genetic data from millions of customers, providing insights into ancestry, health risks, and trait predictions. Their platform leverages machine learning to identify genetic variants associated with various conditions, enabling personalized health recommendations.
Benefits of AI in genomics
- Enhanced accuracy: AI models improve the Precision of genetic variant calling, reducing errors and increasing reliability.
- Scalability: machine learning algorithms can handle large-scale genomic data efficiently, making IT possible to analyze vast datasets quickly.
- personalized insights: AI-driven genomics enables personalized health recommendations, empowering individuals to take proactive steps towards better health.
3. Precision Medicine
Precision medicine aims to tailor treatments to individual patients based on their genetic makeup, lifestyle, and environmental factors. AI plays a crucial role in this field by analyzing complex datasets to identify patterns that can inform personalized treatment plans.
How AI Enhances Precision Medicine
- data integration: AI integrates data from various sources, including electronic health records (EHRs), genomic information, and wearable devices, to provide a holistic view of a patient's health.
- predictive analytics: machine learning algorithms analyze historical data to predict the likelihood of treatment success or failure for individual patients.
- Treatment optimization: AI models optimize treatment plans by considering multiple factors, such as genetic variants, drug interactions, and lifestyle choices.
Case Study: Tempus
Tempus uses AI to integrate clinical and molecular data from cancer patients, providing insights that inform personalized treatment plans. Their platform analyzes genomic sequencing data, EHRs, and other relevant information to identify the most effective therapies for individual patients.
Benefits of AI in Precision Medicine
- Improved Outcomes: Personalized treatment plans based on AI-driven insights lead to better health outcomes and reduced side effects.
- Efficient resource allocation: AI helps allocate resources more effectively by identifying patients who are likely to benefit from specific treatments, reducing waste and improving efficiency.
- Enhanced Patient Experience: Precision medicine empowers patients with personalized health recommendations, Enhancing their overall experience and engagement in healthcare.
4. drug repurposing
drug repurposing involves finding new uses for existing drugs, which can be more cost-effective than developing new medications from scratch. AI accelerates this process by analyzing large datasets to identify potential new indications for approved drugs.
How AI Facilitates drug repurposing
- data Mining: AI models analyze vast amounts of data from clinical trials, scientific literature, and other sources to uncover hidden relationships between diseases and drugs.
- Network analysis: machine learning algorithms create networks that map interactions between drugs, targets, and diseases, identifying potential repurposing opportunities.
- predictive modeling: AI predicts the likelihood of a drug being effective for a new indication based on its molecular properties and historical data.
Case Study: BenevolentAI
BenevolentAI uses machine learning to identify new indications for existing drugs. Their platform analyzed data from scientific literature, clinical trials, and other sources to discover that baricitinib, an approved rheumatoid arthritis drug, could be effective against COVID-19. This finding was later validated in clinical studies.
Benefits of AI in drug repurposing
- cost savings: Repurposing existing drugs is generally less expensive than developing new ones from scratch.
- Faster Time-to-Market: AI-driven insights accelerate the repurposing process, allowing new therapies to reach patients more quickly.
- Enhanced Efficacy: Identifying new indications for approved drugs can lead to more effective treatments for a broader range of conditions.
5. Environmental Biotechnology
AI plays a crucial role in environmental Biotechnology by optimizing processes for waste treatment, pollution control, and resource recovery. machine learning models analyze data from wastewater treatment plants to improve efficiency and reduce energy consumption.
applications of AI in Environmental Biotechnology
- Wastewater Treatment: AI optimizes the operation of wastewater treatment facilities by analyzing real-time data on Water Quality, flow rates, and chemical concentrations.
- Pollution Control: machine learning algorithms monitor environmental changes and predict the impact of pollutants on ecosystems, aiding in the development of strategies for conservation and restoration.
- Resource Recovery: AI helps identify opportunities for recovering valuable resources from waste streams, such as nutrients, metals, and energy.
Case Study: BlueTech research
BlueTech research uses AI to optimize wastewater Treatment Processes. Their platform analyzes data from sensors and other sources to improve the efficiency of treatment facilities, reducing energy consumption and Operational Costs.
Benefits of AI in Environmental Biotechnology
- Improved efficiency: AI-driven insights enhance the performance of waste treatment and pollution control systems, leading to better outcomes and reduced Environmental impact.
- cost savings: Optimizing resource recovery and reducing energy consumption can result in significant cost savings for wastewater treatment facilities.
- Enhanced sustainability: AI helps develop strategies for conservation and restoration, promoting a more sustainable approach to environmental management.
6. Synthetic Biology
Synthetic biology involves designing and Engineering biological systems to perform specific functions. AI plays a crucial role in this field by enabling the design of complex biological circuits and optimizing genetic constructs.
How AI Enhances Synthetic Biology
- design automation: AI automates the design process for synthetic biological systems, generating optimized genetic constructs based on predefined criteria.
- predictive modeling: machine learning algorithms predict the behavior of synthetic biological systems, enabling researchers to test and refine their designs more efficiently.
- data integration: AI integrates data from various sources, such as genomic sequencing, proteomics, and metabolomics, to provide a comprehensive view of synthetic biological systems.
Case Study: Ginkgo Bioworks
Ginkgo Bioworks uses AI to design and engineer biological systems for various applications, including pharmaceuticals, agriculture, and industrial Biotechnology. Their platform analyzes data from genomic sequencing and other sources to optimize genetic constructs and improve the performance of synthetic biological systems.
Benefits of AI in Synthetic Biology
- Enhanced efficiency: Automating the design process and integrating data from multiple sources can significantly enhance the efficiency of synthetic biology research.
- Improved Outcomes: predictive modeling enables researchers to test and refine their designs more effectively, leading to better outcomes and reduced time-to-market.
- Innovation: AI-driven insights facilitate the development of novel biological systems with applications in various fields, from healthcare to agriculture.
7. drug discovery
drug discovery involves identifying new therapeutic compounds that can treat diseases. AI plays a crucial role in this field by analyzing vast amounts of data to identify potential drug candidates and optimize their properties.
How AI Enhances drug discovery
- data Mining: AI models analyze data from scientific literature, clinical trials, and other sources to uncover hidden relationships between molecular structures and biological activity.
- predictive modeling: machine learning algorithms predict the likelihood of a compound being effective against a specific disease based on its molecular properties and historical data.
- optimization: AI optimizes the properties of drug candidates by considering factors such as solubility, stability, and toxicity.
Case Study: Insilico Medicine
Insilico Medicine uses AI to identify new therapeutic compounds for various diseases. Their platform analyzes data from scientific literature, clinical trials, and other sources to uncover hidden relationships between molecular structures and biological activity, enabling the discovery of novel drug candidates.
Benefits of AI in drug discovery
- Enhanced efficiency: AI-driven insights accelerate the drug discovery process, reducing the time and cost associated with identifying new therapeutic compounds.
- Improved Outcomes: predictive modeling enables researchers to test and refine their designs more effectively, leading to better outcomes and reduced side effects.
- Innovation: AI facilitates the development of novel therapeutic compounds with applications in various fields, from oncology to infectious diseases.
8. Agricultural Biotechnology
Agricultural Biotechnology involves using biological systems to improve crop yields, enhance nutritional content, and develop disease-resistant varieties. AI plays a crucial role in this field by analyzing data from genomic sequencing, proteomics, and metabolomics to optimize agricultural practices.
How AI Enhances Agricultural Biotechnology
- data integration: AI integrates data from various sources, such as genomic sequencing, environmental sensors, and satellite imagery, to provide a comprehensive view of agricultural systems.
- predictive modeling: machine learning algorithms predict crop yields, disease outbreaks, and other factors that impact agricultural productivity.
- optimization: AI optimizes agricultural practices by considering factors such as soil health, water availability, and nutrient requirements.
Case Study: Indigo Agriculture
Indigo Agriculture uses AI to optimize agricultural practices for improved crop yields and sustainability. Their platform analyzes data from genomic sequencing, environmental sensors, and other sources to provide farmers with personalized recommendations for optimizing their crops.
Benefits of AI in Agricultural Biotechnology
- Enhanced efficiency: AI-driven insights enable more efficient use of resources, such as water, nutrients, and pesticides, leading to improved crop yields and reduced Environmental impact.
- Improved Outcomes: predictive modeling enables farmers to make informed decisions about agricultural practices, resulting in better outcomes and increased profitability.
- sustainability: AI helps develop strategies for Sustainable Agriculture, promoting a more environmentally friendly approach to food production.
9. Microbiome research
The microbiome refers to the community of microorganisms that live in and on the human body. AI plays a crucial role in microbiome research by analyzing complex datasets to identify patterns that can inform personalized health recommendations.
How AI Enhances Microbiome research
- data integration: AI integrates data from various sources, such as genomic sequencing, metabolomics, and clinical records, to provide a comprehensive view of the microbiome.
- predictive modeling: machine learning algorithms predict the impact of microbial communities on human health based on their composition and function.
- optimization: AI optimizes interventions aimed at modulating the microbiome for improved health outcomes.
Case Study: DayTwo
DayTwo uses AI to analyze data from genomic sequencing, metabolomics, and other sources to provide personalized nutrition recommendations based on an individual's microbiome. Their platform predicts how different foods will affect a person's blood sugar levels, enabling more effective management of conditions such as diabetes and obesity.
Benefits of AI in Microbiome research
- Enhanced efficiency: AI-driven insights accelerate the discovery process for understanding the role of the microbiome in human health.
- Improved Outcomes: predictive modeling enables researchers to test and refine their hypotheses more effectively, leading to better outcomes and personalized health recommendations.
- Innovation: AI facilitates the development of novel interventions aimed at modulating the microbiome for improved health.
10. personalized medicine
personalized medicine involves tailoring medical treatments to individual patients based on their genetic makeup, lifestyle, and environmental factors. AI plays a crucial role in this field by analyzing complex datasets to identify patterns that can inform personalized treatment plans.
How AI Enhances personalized medicine
- data integration: AI integrates data from various sources, such as genomic sequencing, proteomics, and clinical records, to provide a comprehensive view of an individual's health.
- predictive modeling: machine learning algorithms predict the likelihood of a patient responding to a specific treatment based on their genetic makeup and other factors.
- optimization: AI optimizes treatment plans by considering factors such as drug interactions, side effects, and patient preferences.
Case Study: Tempus
Tempus uses AI to analyze data from genomic sequencing, proteomics, and clinical records to provide personalized treatment recommendations for cancer patients. Their platform integrates data from various sources to predict the likelihood of a patient responding to specific treatments, enabling more effective management of cancer.
Benefits of AI in personalized medicine
- Enhanced efficiency: AI-driven insights accelerate the development of personalized treatment plans, reducing the time and cost associated with identifying effective therapies.
- Improved Outcomes: predictive modeling enables healthcare providers to make informed decisions about treatment options, resulting in better outcomes and increased patient satisfaction.
- Innovation: AI facilitates the development of novel therapeutic approaches tailored to individual patients, promoting a more personalized approach to healthcare.
AI has revolutionized various fields within Biotechnology by Enhancing efficiency, improving outcomes, and fostering Innovation. From drug discovery and agricultural Biotechnology to microbiome research and personalized medicine, AI-driven insights are transforming how we approach complex biological systems. As technology continues to advance, the potential for AI in Biotechnology will only grow, paving the way for groundbreaking discoveries and improved health outcomes.