Unlocking Business Value with Explainable AI (XAI)
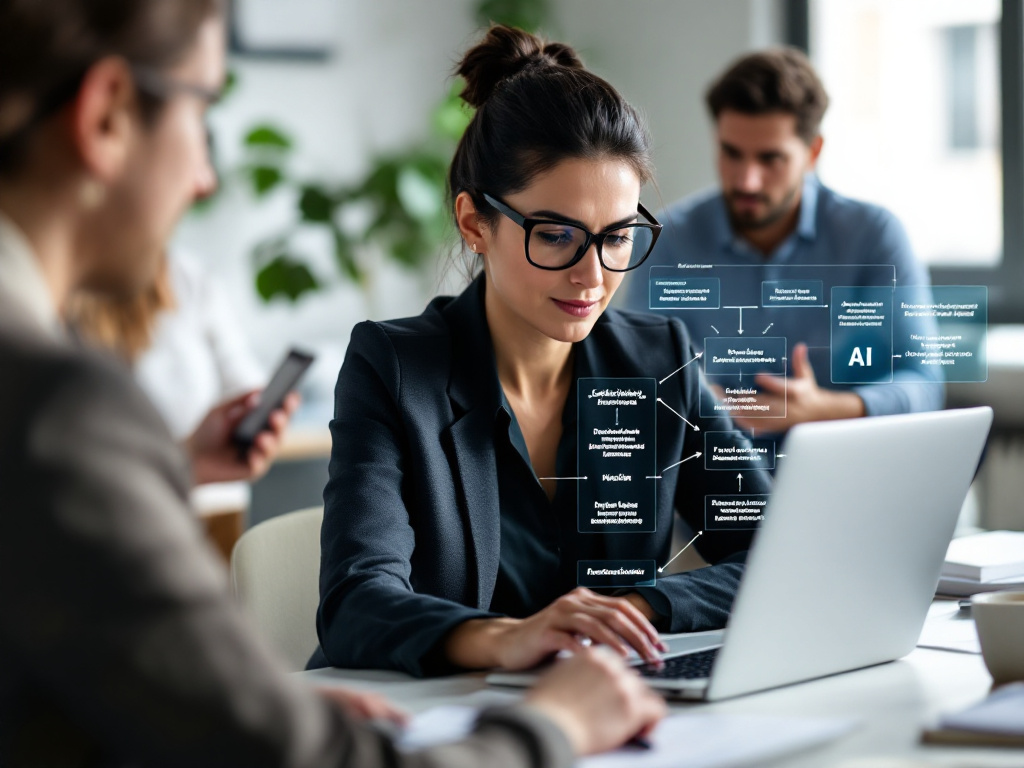
In today's fast-paced business environment, artificial intelligence (AI) has become a cornerstone for driving innovation and efficiency. However, as AI models become increasingly complex, the need for transparency and interpretability has grown. This is where Explainable AI (XAI) comes into play. XAI is a set of processes and methods that enable human users to comprehend and trust the results and output created by machine learning algorithms
. By making AI more understandable, businesses can unlock significant value and gain a competitive edge.
Understanding Explainable AI (XAI)
Explainable AI (XAI) refers to AI systems designed to provide clear explanations of their decision-making processes. Unlike traditional black box AI models, which offer little insight into how they arrive at conclusions, XAI aims to make the inner workings of AI transparent and understandable
. This transparency is crucial for building trust, ensuring compliance, and driving business value.
To illustrate, consider a healthcare scenario where an AI model is used to diagnose diseases. A traditional AI model might accurately predict a patient's condition but provide no insight into how it reached that conclusion. In contrast, an XAI model would not only make the prediction but also explain which factors (e.g., symptoms, medical history, test results) contributed most to the diagnosis. This transparency allows doctors to understand and trust the AI's recommendations, leading to better patient outcomes.
The Importance of Explainability in Business
-
Building Trust and Adoption
Trust is a fundamental aspect of any business relationship, and this extends to the use of AI. When stakeholders, including customers, employees, and regulators, understand how AI systems make decisions, they are more likely to trust and adopt these technologies
. For instance, a bank using XAI to explain loan approval decisions can build greater trust with its customers, as they can see the factors that influenced the decision
.Imagine a customer applying for a loan. If the bank's AI system denies the application, providing an explanation such as Your loan was denied due to a high debt-to-income ratio and a recent late payment on your credit report makes the decision transparent. This transparency builds trust and helps the customer understand what they need to improve for future applications.
In another example, consider an e-commerce platform that uses AI to recommend products to customers. If the recommendations are based on an XAI model, the platform can explain why certain products are suggested. For instance, This product is recommended because you recently purchased similar items and it has high ratings from customers like you. This explanation builds trust with the customer, making them more likely to act on the recommendation.
-
Enhancing Decision-Making
XAI provides valuable insights into the reasoning behind AI-driven recommendations. This can help businesses make more informed decisions and uncover new opportunities. For example, an auto insurer using XAI tools like SHAP values can understand the interactions between vehicle and driver attributes that contribute to risk, allowing them to adjust their models and improve performance
.In a marketing context, XAI can help businesses understand why certain campaigns are successful. For instance, if an AI model recommends targeting a specific demographic with a particular ad, XAI can explain that this demographic has shown high engagement with similar content in the past. This insight allows marketers to refine their strategies and allocate resources more effectively.
Similarly, in the finance sector, XAI can enhance decision-making by providing clear explanations for investment recommendations. For example, an AI model might recommend investing in a particular stock. XAI can explain that this recommendation is based on the stock's strong financial performance, positive market trends, and high analyst ratings. This transparency helps investors make more informed decisions and builds trust in the AI system.
-
Mitigating Risks
Explainability helps organizations mitigate regulatory and other risks. AI systems that run afoul of ethical norms can face intense scrutiny from the public, media, and regulators. By ensuring that AI models are transparent and explainable, businesses can avoid potential legal issues and comply with regulations such as the EU’s AI Act
.For example, a company using AI for hiring decisions must ensure that the model does not discriminate based on protected characteristics like race or gender. XAI can help by explaining how the model makes decisions, allowing the company to identify and address any biases. This proactive approach helps mitigate the risk of legal challenges and reputational damage.
In the healthcare industry, XAI can mitigate risks by providing clear explanations for AI-driven diagnoses. For instance, if an AI model recommends a specific treatment, XAI can explain the reasoning behind this recommendation. This transparency helps doctors verify the AI's findings and ensures that the treatment is appropriate for the patient, reducing the risk of medical errors.
-
Improving Model Performance
With XAI, businesses can troubleshoot and improve model performance by understanding the behaviors of AI models. Continuous model evaluation empowers businesses to compare model predictions, quantify model risk, and optimize performance. This leads to more accurate and reliable AI systems
.In a manufacturing setting, XAI can help engineers understand why a predictive maintenance model flags certain equipment for repair. By explaining which sensors or data points contributed to the prediction, XAI enables engineers to verify the model's accuracy and make necessary adjustments, improving overall equipment reliability.
Similarly, in the retail sector, XAI can improve model performance by providing clear explanations for inventory management recommendations. For example, if an AI model recommends restocking a particular product, XAI can explain that this recommendation is based on high demand and low current stock levels. This transparency helps retailers make more informed decisions and optimize their inventory management processes.
-
Fostering Innovation
XAI encourages a culture of innovation by making AI more accessible to non-technical stakeholders. When everyone in the organization can understand and contribute to AI initiatives, it fosters a collaborative environment that drives innovation and growth
.For instance, a retail company might use AI to optimize inventory management. With XAI, employees across departments—from procurement to sales—can understand how the AI model makes recommendations. This shared understanding encourages collaboration and leads to innovative solutions, such as new inventory strategies or improved customer experiences.
In the technology sector, XAI can foster innovation by providing clear explanations for AI-driven product recommendations. For example, if an AI model recommends adding a new feature to a software product, XAI can explain that this recommendation is based on user feedback and market trends. This transparency helps product managers make more informed decisions and drives innovation in product development.
Applications of XAI in Business
-
Customer Segmentation
XAI helps marketers comprehend the characteristics and factors that contribute to customer segmentation. By explaining how certain customer groups are identified and classified, XAI empowers marketers to tailor their efforts more effectively
.For example, an e-commerce platform might use AI to segment customers based on purchasing behavior. XAI can explain that customers who frequently buy eco-friendly products are more likely to respond to promotions for sustainable brands. This insight allows marketers to create targeted campaigns that resonate with this segment, increasing engagement and sales.
Similarly, in the telecommunications industry, XAI can help with customer segmentation by providing clear explanations for churn prediction models. For instance, if an AI model predicts that a particular customer segment is at high risk of churn, XAI can explain the factors contributing to this prediction, such as poor customer service experiences or competitive offers from other providers. This transparency helps marketers develop targeted retention strategies to reduce churn.
-
Predictive Maintenance
In manufacturing, XAI can clarify why equipment is predicted to fail by providing transparent, traceable explanations of the data and reasoning behind each prediction. This allows businesses to take proactive measures and prevent downtime
.Imagine a factory using AI to monitor machinery. If the AI predicts that a machine is likely to fail, XAI can explain that the prediction is based on unusual vibration patterns and elevated temperature readings. This information enables maintenance teams to address the issue before it causes a breakdown, saving time and money.
In the aviation industry, XAI can be used for predictive maintenance of aircraft engines. For example, if an AI model predicts that an engine component is likely to fail, XAI can explain the factors contributing to this prediction, such as abnormal sensor readings or maintenance history. This transparency helps airlines schedule maintenance proactively, reducing the risk of in-flight failures and improving safety.
-
Fair Lending Practices
Banks can use XAI to provide clear explanations for loan denials, ensuring compliance with regulations and building trust with their customer base
.For instance, a bank might use AI to assess loan applications. If a customer is denied a loan, XAI can explain that the decision was influenced by factors such as a low credit score and insufficient collateral. This transparency helps the bank comply with fair lending practices and builds trust with customers, who understand the reasons behind the decision.
Similarly, in the mortgage industry, XAI can provide clear explanations for mortgage approval decisions. For example, if an AI model approves a mortgage application, XAI can explain the factors contributing to this decision, such as the applicant's strong credit history and stable income. This transparency helps mortgage lenders comply with regulations and builds trust with applicants.
-
Healthcare Diagnostics
In healthcare, XAI can provide transparency in the diagnostic process by offering post-hoc explanations of model outputs. This allows medical professionals to gain a more holistic view of a patient’s case and make better-informed decisions
.Consider a hospital using AI to diagnose diseases from medical images. If the AI detects a potential tumor, XAI can explain which features of the image (e.g., shape, texture, size) contributed to the diagnosis. This information helps doctors verify the AI's findings and ensures that the treatment is appropriate for the patient, reducing the risk of medical errors.
In radiology, XAI can provide clear explanations for AI-driven diagnoses of conditions such as pneumonia or fractures. For example, if an AI model detects pneumonia in a chest X-ray, XAI can explain the features of the image that contributed to this diagnosis, such as the presence of infiltrates or consolidation. This transparency helps radiologists verify the AI's findings and ensures accurate diagnoses.
-
Fraud Detection
XAI can enhance fraud detection systems by providing clear explanations for flagged transactions. This transparency helps fraud analysts understand the reasoning behind the AI's decisions and take appropriate action.
For example, in the financial industry, XAI can explain why a particular transaction was flagged as fraudulent. Factors such as unusual transaction amounts, locations, or patterns can be highlighted by XAI, helping analysts investigate and confirm fraudulent activity. This transparency improves the accuracy of fraud detection systems and reduces false positives.
Similarly, in the insurance industry, XAI can provide clear explanations for fraud detection in claims processing. For instance, if an AI model flags a claim as potentially fraudulent, XAI can explain the factors contributing to this decision, such as inconsistencies in the claim details or the claimant's history. This transparency helps insurance investigators verify the AI's findings and take appropriate action.
Implementing XAI in Your Organization
To unlock the full potential of XAI, organizations should follow these steps:
-
Establish an AI Governance Committee
Form a cross-functional team that includes business leaders, technical experts, and legal and risk professionals. This committee will set standards and guidance for AI development teams and ensure that explainability is a key principle within your responsible AI guidelines
.The committee should define the scope of work, including the business objectives, intended users, technology, and legal requirements for each AI use case. This holistic approach ensures that explainability is integrated into all AI initiatives from the outset.
For example, the committee might develop a framework for evaluating the explainability of AI models, including criteria such as transparency, interpretability, and fairness. This framework can be used to assess AI models throughout their development and deployment, ensuring that they meet the organization's standards for explainability.
-
Invest in the Right Talent and Technology
Hire professionals who understand both the technical and ethical aspects of AI. Invest in explainability tools and technologies that meet the needs of your development teams and stakeholders
.For example, tools like LIME (Local Interpretable Model-Agnostic Explanations) and SHAP (SHapley Additive exPlanations) can help explain AI model predictions. Investing in these tools and training your team to use them effectively will enhance your organization's XAI capabilities.
Additionally, consider investing in platforms that support XAI, such as IBM's Watson OpenScale or Google's Explainable AI tool. These platforms provide comprehensive tools and frameworks for implementing XAI in your organization.
-
Conduct Ongoing Training and Research
Ensure that employees across the organization understand and can apply the latest developments in XAI. Stay updated on legal and regulatory requirements, consumer expectations, and industry norms
.Regular training sessions and workshops can help keep your team informed about new XAI techniques and best practices. Additionally, fostering a culture of continuous learning will encourage employees to explore and implement innovative XAI solutions.
For example, your organization might host workshops on XAI techniques, inviting experts in the field to share their knowledge and insights. These workshops can help your team stay up-to-date with the latest developments in XAI and apply them to your organization's AI initiatives.
-
Continuous Model Evaluation
Regularly evaluate AI models to understand their behaviors and track insights on deployment status, fairness, quality, and drift. This will help optimize model performance and ensure that AI systems remain transparent and trustworthy
.Implementing a robust monitoring framework will enable your organization to identify and address issues such as model bias or performance degradation. This proactive approach ensures that your AI models continue to deliver value over time.
For example, your organization might develop a dashboard for monitoring AI models, displaying key metrics such as accuracy, fairness, and explainability. This dashboard can be used to track the performance of AI models over time and identify any issues that need to be addressed.
Explainable AI (XAI) is a game-changer for businesses looking to harness the power of AI while maintaining transparency and trust. By making AI models more understandable, XAI helps organizations build trust, enhance decision-making, mitigate risks, improve model performance, and foster innovation. As AI continues to evolve, embracing XAI will be crucial for unlocking its full business value and staying ahead of the competition.