Synthetic Data Generation for AI Training
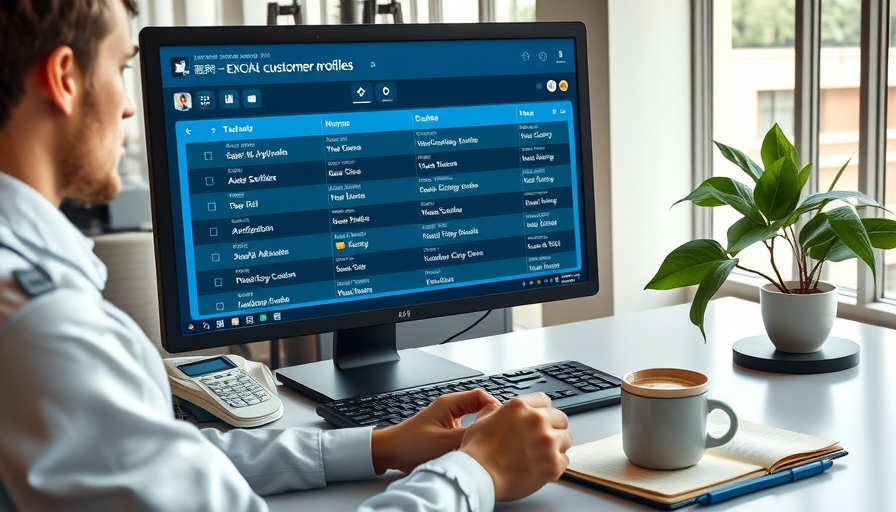
In the rapidly evolving field of artificial intelligence (AI), one of the most significant challenges is obtaining high-quality data to train models effectively. Synthetic data generation has emerged as a powerful solution to address this issue, enabling researchers and developers to create large datasets that are both diverse and representative. This thorough guide will delve into the intricacies of synthetic data generation, its benefits, applications, techniques, challenges, and best practices.
What is Synthetic Data Generation?
Synthetic data generation involves creating artificial datasets that mimic real-world data but are generated algorithmically rather than collected from actual sources. This technique leverages various algorithms and machine learning models to produce data points that can be used for training AI systems without the need for extensive, time-consuming, and sometimes expensive data collection processes.
Key Concepts in Synthetic Data Generation
-
Data Augmentation vs. Synthetic Data Generation:
- Data Augmentation: This involves modifying existing data to create new examples. For instance, rotating or flipping images in a dataset. It is useful but limited by the original data's quality and diversity.
- Synthetic Data Generation: This creates entirely new data points from scratch, often using complex models like Generative Adversarial Networks (GANs) or Variational Autoencoders (VAEs).
-
Deterministic vs. Stochastic Methods:
- Deterministic Methods: These methods use fixed rules to generate data. For example, creating synthetic customer data by randomly selecting names from a list and assigning random but plausible ages and addresses.
- Stochastic Methods: These methods introduce randomness to generate more varied and realistic data. Techniques like GANs fall into this category.
Benefits of Synthetic Data Generation
1. Data Privacy
One of the primary advantages of synthetic data is its ability to preserve privacy. Since the data is artificially generated, it does not contain sensitive information from real individuals, thus mitigating privacy concerns. For example, a healthcare institution can create synthetic patient records that mimic real data but do not reveal any personal health information.
2. Scalability
Synthetic datasets can be scaled easily to meet the demands of AI training. This scalability ensures that models can be trained on large volumes of data without the limitations imposed by real-world data availability. For instance, an autonomous vehicle company can generate millions of synthetic driving scenarios to train its self-driving algorithms.
3. Diversity and Variety
Synthetic data can be designed to include a wide range of scenarios, edge cases, and variations that might not be present in actual datasets, leading to more robust AI models. For example, a fraud detection system can be trained on synthetic data that includes various types of fraudulent transactions that are rare in real-world data.
4. Cost-Effectiveness
Generating synthetic data is often more cost-effective than collecting and labeling real-world data. This is particularly beneficial for industries where data collection is expensive or time-consuming, such as healthcare or finance.
5. Flexibility
Synthetic data can be tailored to specific needs and scenarios, allowing for greater flexibility in AI training. For instance, a company developing a new product can generate synthetic user data to test different features and improve the product before it hits the market.
Applications of Synthetic Data Generation
1. Healthcare
In healthcare, synthetic data generation can create realistic patient records for training medical AI systems without compromising patient privacy. For example, synthetic Electronic Health Records (EHRs) can be used to train diagnostic algorithms, ensuring that sensitive patient information remains protected.
Example: A hospital wants to develop an AI system to predict patient deterioration. By generating synthetic EHR data, the hospital can train the AI model on a diverse set of patient scenarios without accessing real patient records.
2. Finance
Financial institutions use synthetic data to develop fraud detection algorithms and risk assessment models by simulating various financial scenarios. For instance, synthetic transaction data can be used to train machine learning models to detect unusual patterns indicative of fraudulent activity.
Example: A bank wants to improve its fraud detection system. By generating synthetic transaction data that includes both legitimate and fraudulent transactions, the bank can train its AI model to identify suspicious activities more accurately.
3. Autonomous Vehicles
For autonomous driving, synthetic datasets can be used to train self-driving cars in a variety of simulated environments, reducing the need for extensive real-world testing. This is particularly useful for rare or dangerous scenarios that are difficult to replicate in the real world.
Example: An automotive company wants to test its autonomous vehicle's ability to handle emergency situations. By generating synthetic data that simulates various emergency scenarios, the company can train its AI model to respond appropriately without putting real drivers at risk.
4. Retail
In retail, synthetic data can be used to simulate customer behavior and preferences, helping companies optimize their marketing strategies and improve customer experience. For instance, synthetic customer data can be used to train recommendation algorithms that suggest products tailored to individual preferences.
Example: An e-commerce platform wants to enhance its product recommendation system. By generating synthetic customer data that includes browsing history, purchase patterns, and demographic information, the platform can train its AI model to provide more accurate and personalized recommendations.
5. Manufacturing
In manufacturing, synthetic data can be used to simulate production processes and identify potential issues before they occur. This helps in improving efficiency and reducing downtime. For example, synthetic sensor data can be used to train predictive maintenance models that predict equipment failures.
Example: A manufacturing company wants to improve its predictive maintenance system. By generating synthetic sensor data that mimics various machine conditions, the company can train its AI model to detect early signs of equipment failure and schedule maintenance accordingly.
Techniques for Synthetic Data Generation
1. Generative Adversarial Networks (GANs)
GANs are a type of AI algorithm that can generate highly realistic synthetic data by training two neural networks against each other: a generator and a discriminator. The generator creates synthetic data, while the discriminator evaluates its authenticity. This adversarial process continues until the generator produces data that is indistinguishable from real data.
Example: A company wants to create synthetic images of faces for a facial recognition system. By training a GAN on a dataset of real faces, the company can generate synthetic face images that are highly realistic and diverse.
2. Variational Autoencoders (VAEs)
VAEs are another method used to generate new data points by learning the underlying distribution of real data and sampling from it. VAEs consist of an encoder that compresses input data into a latent space and a decoder that reconstructs the data from the latent space. By sampling from the latent space, VAEs can generate new data points that are similar to the original data.
Example: A research lab wants to create synthetic molecular structures for drug discovery. By training a VAE on a dataset of known molecules, the lab can generate synthetic molecule structures that have similar properties to existing drugs.
3. Rule-Based Methods
Rule-based methods involve defining a set of rules or templates that dictate how synthetic data should be generated, ensuring consistency and control over the output. These rules can be based on statistical distributions, logical constraints, or domain-specific knowledge.
Example: A marketing firm wants to create synthetic customer profiles for market research. By defining rules that specify the distribution of age, gender, income, and other demographic factors, the firm can generate a large dataset of synthetic customer profiles that reflect the target market's characteristics.
4. Agent-Based Modeling
Agent-based modeling involves simulating the behavior of individual agents (e.g., people, vehicles, or cells) within a system to generate synthetic data. Each agent follows a set of rules or behaviors, and their interactions produce complex patterns that can be used for training AI models.
Example: A traffic management agency wants to simulate urban traffic flow to optimize signal timing. By creating an agent-based model where each vehicle is an agent following traffic rules, the agency can generate synthetic traffic data that reflects real-world conditions.
5. Differential Privacy
Differential privacy is a technique used to add noise to data in a way that preserves privacy while maintaining the overall distribution of the data. This method ensures that individual data points cannot be reconstructed from the synthetic data, providing an additional layer of privacy protection.
Example: A government agency wants to release synthetic census data for public use. By applying differential privacy techniques, the agency can add noise to the data in a way that preserves the overall distribution while protecting the privacy of individual respondents.
Challenges in Synthetic Data Generation
While synthetic data generation offers numerous benefits, there are also challenges to consider:
1. Data Realism
Ensuring that synthetic data is realistic enough to effectively train AI models can be a complex task. If the synthetic data does not accurately represent real-world conditions, the resulting AI model may perform poorly when deployed in real scenarios.
Mitigation: Regularly validate synthetic datasets against real-world data to ensure their quality and relevance. Use domain experts to evaluate the realism of the generated data.
2. Computational Resources
Generating high-quality synthetic datasets often requires significant computational power and resources. This can be a barrier for organizations with limited access to advanced computing infrastructure.
Mitigation: Leverage cloud-based solutions and distributed computing frameworks to scale up synthetic data generation processes. Optimize algorithms to reduce computational requirements without sacrificing data quality.
3. Data Bias
Synthetic data generated from biased real-world data can perpetuate or even amplify existing biases. This is particularly problematic in applications where fairness and equity are crucial, such as hiring algorithms or criminal justice systems.
Mitigation: Carefully design synthetic data generation processes to minimize bias. Use diverse and representative datasets for training generative models and regularly audit the generated data for potential biases.
4. Ethical Considerations
The use of synthetic data raises ethical questions related to privacy, fairness, and transparency. Organizations must ensure that synthetic data is used responsibly and ethically, with a clear understanding of its limitations and potential impacts.
Mitigation: Develop ethical guidelines for synthetic data generation and usage. Engage stakeholders, including ethical reviewers and affected communities, in the design and implementation of synthetic data projects.
Best Practices for Synthetic Data Generation
To maximize the effectiveness of synthetic data generation, consider the following best practices:
1. Understand Your Use Case
Tailor your synthetic data generation strategy to the specific requirements of your AI application. Different use cases may have unique needs in terms of data types, quality, and diversity.
Example: A company developing a natural language processing (NLP) system for customer service should focus on generating synthetic text data that covers a wide range of customer inquiries and responses.
2. Validate Synthetic Data
Regularly validate synthetic datasets against real-world data to ensure their quality and relevance. This can be done through statistical analysis, domain expert review, or comparison with benchmark datasets.
Example: A healthcare institution generating synthetic patient records should compare the distribution of diagnosis codes in the synthetic data with those in real EHR data to ensure accuracy.
3. Leverage Expertise
Engage with experts in data science and machine learning to develop and refine your synthetic data generation techniques. Their knowledge can help overcome technical challenges and ensure the quality of the generated data.
Example: A financial institution developing a fraud detection system should collaborate with data scientists and domain experts to design effective synthetic data generation methods that capture the nuances of fraudulent behavior.
4. Ensure Diversity
Generate synthetic data that covers a wide range of scenarios, edge cases, and variations to ensure robustness in AI training. This includes considering different demographic groups, geographical regions, and use cases.
Example: A company developing an image recognition system should generate synthetic images that include diverse lighting conditions, angles, and object variations to improve the model's generalization capabilities.
5. Document Processes
Maintain detailed documentation of your synthetic data generation processes, including algorithms used, parameters set, and validation methods employed. This ensures transparency and reproducibility, making it easier to troubleshoot issues and adapt to changing requirements.
Example: A research lab generating synthetic molecular structures for drug discovery should document the training process of their generative model, including hyperparameters and validation results.
6. Iterate and Improve
Synthetic data generation is an iterative process. Continuously collect feedback from users and stakeholders, and use it to refine your methods and improve the quality of the generated data.
Example: A marketing firm generating synthetic customer profiles should gather feedback from market researchers on the usefulness and accuracy of the synthetic data, and use this feedback to enhance future iterations.
Case Studies
Case Study 1: Synthetic Data for Healthcare AI
A leading healthcare institution aimed to develop an AI system for predicting patient deterioration. However, accessing real patient records was constrained by stringent privacy regulations. The institution turned to synthetic data generation using GANs to create realistic but anonymized patient records.
Process:
- Data Collection: Real EHR data from past patients were used as the basis for training the GAN.
- Model Training: A GAN was trained to generate synthetic EHR data that mimicked the distribution and patterns of real data.
- Validation: The generated synthetic data was validated against a subset of anonymized real EHR data to ensure accuracy and realism.
- Deployment: The synthetic data was used to train an AI model for predicting patient deterioration, significantly improving the system's performance without compromising patient privacy.
Outcome:
The AI system trained on synthetic data achieved high accuracy in predicting patient deterioration, enabling early intervention and improved patient outcomes. The use of synthetic data ensured compliance with privacy regulations while providing a scalable solution for training AI models.
Case Study 2: Synthetic Data for Autonomous Vehicles
An automotive company wanted to enhance the safety and reliability of its self-driving vehicles by generating synthetic driving scenarios. Real-world testing was limited due to safety concerns and logistical constraints, making synthetic data an attractive alternative.
Process:
- Data Collection: Real-world driving data were collected using on-board sensors and cameras.
- Scenario Design: Various driving scenarios, including emergency situations like sudden lane changes or pedestrian crossings, were designed.
- Simulation: An agent-based modeling approach was used to simulate the behavior of vehicles and pedestrians in these scenarios.
- Data Generation: Synthetic sensor data, including LiDAR, camera images, and GPS coordinates, were generated for each scenario.
- Validation: The synthetic data were validated against real-world driving data to ensure realism and accuracy.
- Deployment: The synthetic data were used to train the AI models powering the autonomous vehicles, significantly improving their ability to handle complex and dangerous situations.
Outcome:
The self-driving vehicles trained on synthetic data demonstrated improved performance in real-world conditions, handling emergency scenarios more effectively. This resulted in enhanced safety features and a reduction in accidents involving autonomous vehicles.
Synthetic data generation is a game-changer for AI training, providing a scalable, privacy-preserving, and diverse solution for developing robust AI models. By understanding the benefits, applications, techniques, challenges, and best practices associated with synthetic data, organizations can harness its potential to drive innovation and success in their AI endeavors.
As AI continues to evolve, synthetic data generation will play an increasingly important role in addressing data scarcity, privacy concerns, and the need for diverse and representative datasets. By embracing this technology, companies and researchers can push the boundaries of what is possible with AI, leading to more accurate, fair, and reliable systems.