Revolutionizing Disaster Recovery: AI Integration for Enhanced Resilience and Efficiency
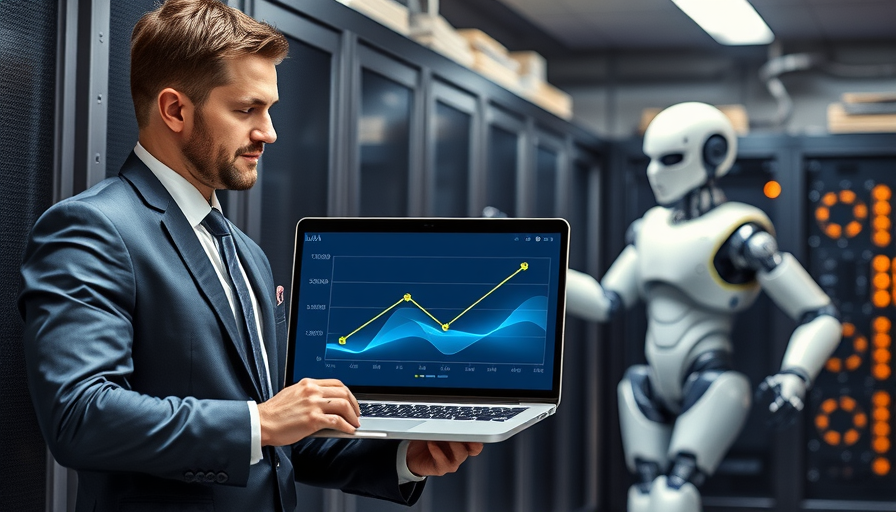
In today's rapidly evolving digital landscape, ensuring business continuity and minimizing downtime has become more critical than ever. Disaster recovery, a crucial component of business continuity planning, is undergoing a significant transformation with the integration of artificial intelligence (AI). This blog post explores how AI is revolutionizing disaster recovery, enhancing resilience, and improving efficiency for organizations worldwide.
The Evolution of Disaster Recovery
Traditional disaster recovery methods have relied heavily on manual processes, physical infrastructure, and predefined recovery plans. While these methods have served their purpose, they often fall short in addressing the complexities and dynamics of modern IT environments. The introduction of AI in disaster recovery is changing the game by offering more intelligent, adaptive, and proactive solutions.
Traditional Disaster Recovery Methods
-
Manual Processes: Traditional disaster recovery often involves manual interventions, such as backing up data to physical storage devices and manually restoring systems. These processes are time-consuming and prone to human error.
-
Physical Infrastructure: Traditional methods rely on physical infrastructure, such as data centers and backup servers. These infrastructure components require significant investment and maintenance, and they can be vulnerable to physical disasters like floods or fires.
-
Predefined Recovery Plans: Traditional disaster recovery plans are often static and predefined. They are based on anticipated scenarios and may not adapt well to unforeseen events or changing conditions.
Limitations of Traditional Methods
- Time-Consuming: Manual processes can take hours or even days to complete, leading to extended downtime and potential data loss.
- Error-Prone: Human intervention increases the risk of errors, which can further complicate the recovery process.
- Costly: Maintaining physical infrastructure and backup systems can be expensive, requiring ongoing investment in hardware and maintenance.
- Inflexible: Predefined recovery plans may not adapt well to new threats or changing conditions, limiting their effectiveness in dynamic environments.
Key Benefits of AI Integration in Disaster Recovery
1. Predictive Analytics
AI-powered predictive analytics enable organizations to foresee potential disruptions before they occur. By analyzing historical data, real-time metrics, and external factors, AI can identify patterns and trends that indicate impending failures or vulnerabilities. This proactive approach allows businesses to take preventive measures, reducing the likelihood of disasters and minimizing their impact.
How Predictive Analytics Works
- Data Collection: AI systems collect data from various sources, including system logs, performance metrics, and external data feeds.
- Pattern Recognition: Machine learning algorithms analyze the collected data to identify patterns and trends that indicate potential disruptions.
- Risk Assessment: AI assesses the risk associated with identified patterns and prioritizes preventive measures based on the likelihood and potential impact of disruptions.
- Preventive Actions: Based on the risk assessment, AI can trigger automated preventive actions, such as system updates, configuration changes, or resource reallocation.
Example: Predicting Hardware Failures
Imagine a data center with thousands of servers. Traditional monitoring systems might alert administrators to a hardware failure only after it occurs. However, an AI-powered predictive analytics system can analyze historical data and real-time metrics to identify patterns that indicate impending hardware failures. For example, if a server's temperature and fan speed data show unusual patterns, the AI system can predict a potential failure and trigger preventive maintenance before the failure occurs.
2. Automated Response
AI can automate the disaster recovery process, significantly reducing the time and effort required to restore operations. Automated response systems can quickly assess the situation, prioritize recovery tasks, and execute predefined recovery plans without human intervention. This ensures a faster and more efficient recovery process, minimizing downtime and reducing the risk of data loss.
How Automated Response Works
- Incident Detection: AI systems continuously monitor IT environments for signs of disruptions or failures.
- Impact Assessment: Upon detecting an incident, AI assesses its impact on operations and prioritizes recovery tasks based on criticality.
- Recovery Plan Execution: AI executes predefined recovery plans, including data restoration, system reconfiguration, and service redeployment.
- Validation: AI validates the recovery process to ensure that systems are restored to their normal operating state.
Example: Automated Response to a Cyber Attack
Consider a scenario where a cyber attack targets an organization's e-commerce platform. Traditional disaster recovery methods might require manual intervention to identify the attack, isolate affected systems, and restore services. However, an AI-powered automated response system can detect the attack in real-time, isolate affected systems, and initiate recovery processes automatically. For example, the AI system can trigger data backups, reconfigure network settings, and redeploy services to unaffected servers, minimizing downtime and reducing the impact of the attack.
3. Enhanced Resilience
AI enhances the resilience of IT systems by continuously monitoring and optimizing their performance. Machine learning algorithms can adapt to changing conditions, dynamically allocating resources and adjusting configurations to maintain optimal performance. This adaptability ensures that systems remain resilient even in the face of unexpected disruptions.
How AI Enhances Resilience
- Continuous Monitoring: AI systems continuously monitor system performance, identifying potential issues and optimizing configurations in real-time.
- Dynamic Resource Allocation: AI can dynamically allocate resources, such as CPU, memory, and storage, based on current demand and performance metrics.
- Adaptive Configuration: AI can adjust system configurations, such as network settings and application parameters, to optimize performance and resilience.
- Self-Healing: AI can initiate self-healing processes, such as automated repairs and system reconfigurations, to maintain optimal performance and resilience.
Example: Maintaining System Performance During Peak Loads
Imagine an e-commerce platform experiencing a sudden surge in traffic during a holiday sale. Traditional systems might struggle to handle the increased load, leading to performance degradation or even system failures. However, an AI-powered system can continuously monitor performance metrics and dynamically allocate resources to handle the increased load. For example, the AI system can scale up server capacity, optimize network settings, and adjust application parameters to maintain optimal performance and ensure a seamless user experience.
4. Improved Efficiency
AI integration in disaster recovery improves overall efficiency by streamlining processes, reducing manual effort, and eliminating human error. AI-driven systems can handle complex tasks more accurately and quickly than human counterparts, freeing up IT staff to focus on strategic initiatives and other critical tasks.
How AI Improves Efficiency
- Process Automation: AI can automate repetitive and time-consuming tasks, such as data backups, system updates, and performance monitoring.
- Error Reduction: AI-driven systems can perform tasks with high accuracy, reducing the risk of human error and ensuring consistent results.
- Resource Optimization: AI can optimize resource allocation and utilization, ensuring that systems operate efficiently and cost-effectively.
- Real-Time Decision Making: AI can provide real-time insights and recommendations, enabling IT staff to make informed decisions quickly and efficiently.
Example: Streamlining Data Backup Processes
Consider a scenario where an organization needs to back up large volumes of data regularly. Traditional methods might require manual intervention to schedule backups, monitor progress, and validate data integrity. However, an AI-powered system can automate the entire backup process, including scheduling, monitoring, and validation. For example, the AI system can analyze data usage patterns to optimize backup schedules, monitor backup progress in real-time, and validate data integrity automatically, ensuring efficient and reliable data backups.
5. Cost Savings
By automating disaster recovery processes and reducing downtime, AI can lead to significant cost savings for organizations. Faster recovery times mean less revenue loss, and automated systems require fewer resources, lowering operational costs. Additionally, AI can help optimize resource allocation, further reducing expenses.
How AI Reduces Costs
- Reduced Downtime: AI-driven systems can minimize downtime by automating recovery processes and reducing the time required to restore operations.
- Lower Operational Costs: AI can reduce the need for manual intervention, lowering operational costs and freeing up IT staff for other tasks.
- Optimized Resource Allocation: AI can optimize resource allocation and utilization, ensuring that systems operate cost-effectively.
- Proactive Maintenance: AI can predict and prevent potential disruptions, reducing the need for costly repairs and replacements.
Example: Optimizing Data Center Operations
Imagine a data center with thousands of servers and storage devices. Traditional methods might require significant investment in hardware and maintenance to ensure reliable operations. However, an AI-powered system can optimize data center operations by predicting hardware failures, automating maintenance tasks, and dynamically allocating resources. For example, the AI system can predict impending hardware failures and trigger preventive maintenance, reducing the need for costly repairs and replacements. Additionally, the AI system can dynamically allocate resources based on current demand, ensuring efficient and cost-effective operations.
Real-World Applications
Case Study 1: Financial Services
In the financial services industry, AI-driven disaster recovery systems have proven invaluable in maintaining business continuity. Banks and financial institutions rely on AI to monitor transactions, detect anomalies, and trigger automated recovery processes in real-time. This ensures that critical financial services remain available even during disruptions.
Example: Detecting and Mitigating Fraudulent Transactions
Consider a scenario where a financial institution experiences a sudden surge in fraudulent transactions. Traditional methods might require manual intervention to identify and mitigate fraudulent activities. However, an AI-powered system can detect fraudulent transactions in real-time, isolate affected accounts, and initiate recovery processes automatically. For example, the AI system can analyze transaction patterns to identify anomalies, isolate affected accounts, and trigger automated recovery processes, such as account suspension and transaction reversal, ensuring minimal disruption to financial services.
Case Study 2: Healthcare
Healthcare organizations have also benefited from AI integration in disaster recovery. AI-powered systems can monitor patient data, hospital operations, and IT infrastructure to predict and mitigate potential disruptions. This ensures that patient care remains uninterrupted, even in the face of natural disasters or cyber-attacks.
Example: Maintaining Patient Care During Natural Disasters
Imagine a healthcare organization facing a natural disaster, such as a hurricane or earthquake. Traditional methods might struggle to maintain patient care during such disruptions. However, an AI-powered system can predict and mitigate potential disruptions by monitoring patient data, hospital operations, and IT infrastructure. For example, the AI system can analyze weather forecasts and hospital operations data to predict potential disruptions and initiate preventive measures, such as evacuating patients, securing medical equipment, and ensuring IT infrastructure resilience. This ensures that patient care remains uninterrupted, even during natural disasters.
Case Study 3: Retail
In the retail sector, AI-driven disaster recovery solutions help maintain operational continuity during peak shopping seasons. By predicting and addressing potential disruptions, AI ensures that e-commerce platforms and point-of-sale systems remain functional, preventing revenue loss and maintaining customer satisfaction.
Example: Ensuring Seamless Operations During Peak Shopping Seasons
Consider a scenario where a retail organization experiences a sudden surge in online traffic during a peak shopping season. Traditional methods might struggle to handle the increased load, leading to performance degradation or even system failures. However, an AI-powered system can predict and address potential disruptions by monitoring online traffic, system performance, and IT infrastructure. For example, the AI system can analyze traffic patterns and system performance data to predict potential disruptions and initiate preventive measures, such as scaling up server capacity, optimizing network settings, and ensuring IT infrastructure resilience. This ensures that e-commerce platforms and point-of-sale systems remain functional, preventing revenue loss and maintaining customer satisfaction.
The integration of AI in disaster recovery is transforming the way organizations approach business continuity. By leveraging predictive analytics, automated response systems, and adaptive technologies, AI enhances resilience, improves efficiency, and delivers significant cost savings. As AI continues to evolve, its role in disaster recovery will become even more critical, enabling organizations to thrive in an increasingly unpredictable world.