Predicting Infrastructure Failures with AI
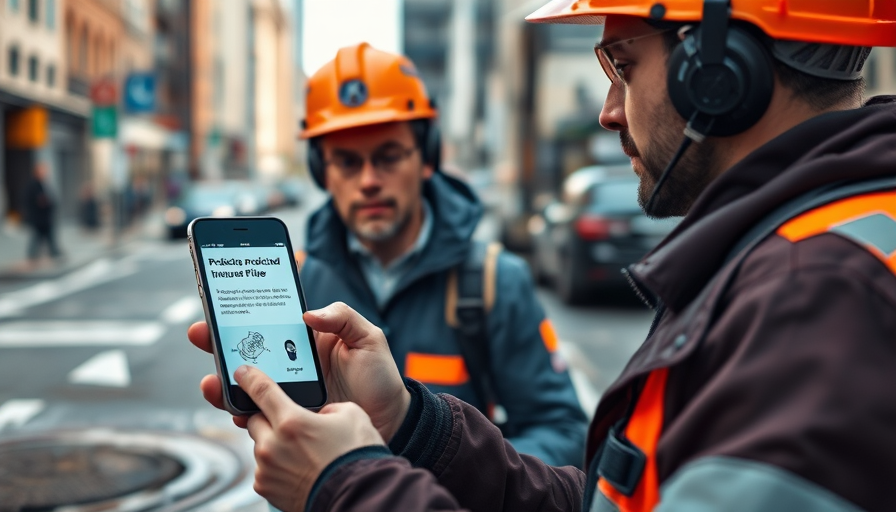
In today's interconnected world, infrastructure failures can have cascading effects, leading to significant economic losses, safety hazards, and disruptions in services. From collapsing bridges to widespread power outages and network disruptions, these incidents highlight the need for proactive maintenance strategies. Artificial Intelligence (AI) offers a transformative approach to predicting and preventing infrastructure failures. This comprehensive guide explores the role of AI in infrastructure monitoring, its benefits, successful case studies, future trends, and considerations for implementation.
The Role of AI in Predicting infrastructure Failures
AI has emerged as a game-changer in monitoring and maintaining the integrity of various infrastructure systems. By leveraging machine learning algorithms, predictive analytics, and real-time data processing, AI can identify patterns and anomalies that indicate impending failures. This Proactive approach enables timely interventions, reducing downtime and Enhancing safety.
How AI Works in infrastructure Monitoring
Predicting infrastructure failures with AI involves several key steps:
- data Collection: sensors and Internet of Things (IoT) devices gather real-time data from various infrastructure components.
- data Preprocessing: Raw data is cleaned, normalized, and transformed into a format suitable for analysis.
- data analysis: Machine Learning algorithms analyze the processed data to detect anomalies and predict potential issues.
- Predictive Maintenance: Based on predictions, maintenance teams can schedule repairs or upgrades before a failure occurs.
- continuous monitoring: AI systems continuously monitor infrastructure performance, updating predictions as new data becomes available.
data Collection
Effective infrastructure monitoring begins with robust data collection. sensors and IoT devices are deployed throughout the infrastructure to collect real-time data on various parameters such as temperature, vibration, strain, pressure, and more. For example:
- Bridges: Strain gauges and accelerometers monitor structural stress and vibrations.
- Power Grids: smart meters and phasor measurement units (PMUs) track electrical parameters like voltage, current, and frequency.
- Railways: Wheel sensors and track circuits detect train movements and potential track defects.
data Preprocessing
Raw data collected from sensors often contains noise, missing values, and inconsistencies. data preprocessing involves cleaning and transforming this raw data into a format suitable for analysis. Techniques include:
- Filtering: Removing noise and irrelevant data points.
- Normalization: Scaling data to a standard range (e.g., 0 to 1) to ensure consistent analysis.
- Imputation: Filling in missing values using statistical methods or machine learning algorithms.
data analysis
Machine Learning algorithms analyze the preprocessed data to detect anomalies and predict potential failures. Common Techniques include:
- Supervised Learning: Algorithms like support vector machines (SVM) and random forests are trained on labeled data to classify infrastructure conditions.
- Unsupervised Learning: Clustering Algorithms like K-means identify patterns and outliers in unlabeled data.
- reinforcement learning: Agents learn optimal maintenance strategies by interacting with the infrastructure Environment.
Predictive Maintenance
Predictive maintenance leverages AI predictions to schedule repairs or upgrades before a failure occurs. This Proactive approach minimizes downtime and reduces maintenance costs. For example:
- Aircraft Engines: sensors monitor engine performance, and AI algorithms predict when components are likely to fail, enabling timely maintenance.
- manufacturing Equipment: Machine Learning models analyze vibration data to forecast equipment failures, allowing for scheduled repairs during downtime.
continuous monitoring
AI systems continuously monitor infrastructure performance, updating predictions as new data becomes available. This real-time monitoring enables dynamic decision-making and adaptive maintenance strategies. For instance:
- smart buildings: AI-powered building management systems (BMS) monitor energy consumption, HVAC performance, and occupancy levels to optimize comfort and efficiency.
- Water Distribution Networks: Real-time sensors and AI algorithms detect leaks and pressure drops, enabling prompt repairs and minimizing water loss.
Benefits of Using AI for infrastructure Monitoring
AI-driven infrastructure monitoring offers numerous benefits, including cost savings, enhanced safety, and improved operational efficiency.
cost savings
predicting failures early allows organizations to avoid costly repairs and downtime. By scheduling maintenance proactively, companies can extend the lifespan of their assets and reduce overall maintenance costs. For example:
- Wind Turbines: AI algorithms analyze vibration data to predict gearbox failures, enabling timely maintenance and reducing repair costs.
- Road Maintenance: Sensor networks detect pavement deterioration, allowing for proactive repairs and minimizing long-term expenses.
safety Enhancements
AI systems can identify potential safety hazards, reducing the risk of accidents and ensuring the well-being of users. For instance:
- Tunnels: AI-powered monitoring systems detect structural deformations and water ingress, preventing collapses and Enhancing passenger safety.
- Oil and Gas Pipelines: Machine Learning models analyze pressure and flow data to predict leaks and corrosion, minimizing environmental risks.
efficiency Improvements
Predictive maintenance schedules ensure that infrastructure is maintained at optimal performance levels, Enhancing overall efficiency. For example:
- data Centers: AI algorithms optimize cooling systems by analyzing temperature and humidity data, reducing energy consumption and improving server uptime.
- Public Transportation: Predictive analytics forecast passenger demand, enabling optimized scheduling and Route Planning.
case studies: Successful Implementation
smart cities
smart cities leverage AI-driven infrastructure monitoring to enhance urban management and improve the Quality of life for residents. For example:
- Singapore: The city-state uses sensors and AI algorithms to monitor traffic flow, optimize signal timing, and reduce congestion.
- Barcelona: Barcelona's smart streetlights use AI to adjust brightness based on real-time occupancy data, saving energy and reducing light pollution.
bridge monitoring
In Singapore, sensors embedded in bridges continuously monitor structural integrity. Machine Learning algorithms analyze vibration and strain data to detect anomalies and predict potential failures. This Proactive approach ensures the safety of commuters and reduces maintenance costs.
traffic management
Barcelona's smart traffic management system uses AI to optimize signal timing based on real-time traffic data. By reducing congestion and minimizing travel times, the city enhances mobility and reduces carbon emissions.
Industrial applications
In industrial settings, AI-powered predictive maintenance systems have proven highly effective in Enhancing operational efficiency and reducing downtime. For example:
- manufacturing: Factories use machine learning algorithms to analyze data from machinery, predicting equipment failures and scheduling preventive maintenance.
- Mining: AI-driven monitoring systems detect potential safety hazards and optimize resource extraction processes.
Predictive Maintenance in manufacturing
In a manufacturing plant, sensors monitor vibration, temperature, and pressure in critical machinery. Machine Learning models analyze this data to predict component failures, enabling timely maintenance and minimizing production downtime.
safety Enhancements in Mining
AI-powered monitoring systems detect potential safety hazards like gas leaks and roof collapses in mining operations. By alerting miners to these risks, the system enhances worker safety and reduces accidents.
Transportation infrastructure
AI is revolutionizing Transportation infrastructure by Enhancing safety, efficiency, and reliability. For example:
- Railways: AI algorithms analyze track and wheel sensor data to predict potential defects and schedule maintenance proactively.
- Aviation: Predictive maintenance systems monitor aircraft engine performance, detecting anomalies and predicting failures before they occur.
Track Monitoring in Railways
In a railway network, sensors embedded in tracks detect vibrations and stress levels. Machine Learning models analyze this data to identify potential defects, enabling timely repairs and minimizing disruptions to service.
Engine Monitoring in Aviation
Airlines use AI-powered engine monitoring systems to analyze performance data in real-time. By detecting anomalies and predicting failures, the system enables proactive maintenance, Enhancing safety and reducing Operational Costs.
future trends in Predictive infrastructure Maintenance
The future of infrastructure monitoring with AI is promising, with several emerging trends set to enhance accuracy and efficiency:
deep learning
deep learning Techniques, such as Convolutional Neural Networks (CNNs) and recurrent neural networks (RNNs), are being increasingly used for infrastructure monitoring. These models can analyze complex data patterns and provide more accurate predictions.
- image recognition: CNNs can analyze visual data from drones or cameras to detect structural defects in bridges and buildings.
- Time Series analysis: RNNs can model temporal dependencies in sensor data, predicting failures based on historical trends.
Edge computing
Edge computing enables real-time data processing at the source, reducing latency and Enhancing the responsiveness of AI systems. This is particularly beneficial for infrastructure monitoring, where timely interventions are crucial.
- Smart sensors: Edge-enabled sensors process data locally, sending only relevant information to central systems.
- Real-Time decision making: Edge computing facilitates real-time decision-making, enabling immediate responses to detected anomalies.
5G technology
The rollout of 5G technology promises to enhance the capabilities of AI-driven infrastructure monitoring. With faster data transfer rates and lower latency, 5G enables more efficient data collection and analysis.
- Enhanced Connectivity: 5G networks support a higher density of IoT devices, enabling comprehensive infrastructure monitoring.
- Real-Time data Processing: Lower latency allows for real-time data processing, Enhancing the responsiveness of AI systems.
Blockchain technology
Blockchain technology can ensure data security and transparency in infrastructure management. By providing an immutable record of transactions and events, Blockchain enhances trust and accountability.
- Secure data Sharing: Blockchain enables secure sharing of sensor data between stakeholders, ensuring data integrity.
- Transparent Maintenance Records: Immutable records of maintenance activities enhance transparency and accountability.
challenges and Considerations
While AI offers numerous benefits for predicting infrastructure failures, there are challenges to consider:
data Quality
The effectiveness of AI systems depends on high-quality data. Ensuring accurate and reliable data collection is crucial. Factors affecting data quality include:
- Sensor Calibration: Regular calibration of sensors ensures accurate data collection.
- Environmental Interference: External factors like weather conditions can affect sensor readings, requiring robust filtering Techniques.
integration Complexity
Integrating AI solutions with existing infrastructure can be complex and requires careful planning. Considerations include:
- Compatibility: Ensuring that new AI systems are compatible with legacy infrastructure components.
- scalability: Designing scalable AI solutions that can handle increasing data volumes and complexities.
Ethical and privacy Concerns
AI-driven infrastructure monitoring raises ethical and privacy concerns, particularly regarding data collection and usage. Key considerations include:
- data privacy: Ensuring that personal data collected from sensors is anonymized and secure.
- Bias in Algorithms: Mitigating biases in machine learning algorithms to ensure fair and unbiased decision-making.
Predicting infrastructure failures with AI represents a significant leap forward in maintaining the integrity and safety of our built Environment. By leveraging Machine Learning, predictive analytics, and real-time data processing, organizations can proactively address potential issues, save costs, and enhance operational efficiency. As technology continues to evolve, the future of infrastructure monitoring looks increasingly promising.