Navigating AI Deployment: Overcoming Infrastructure Challenges
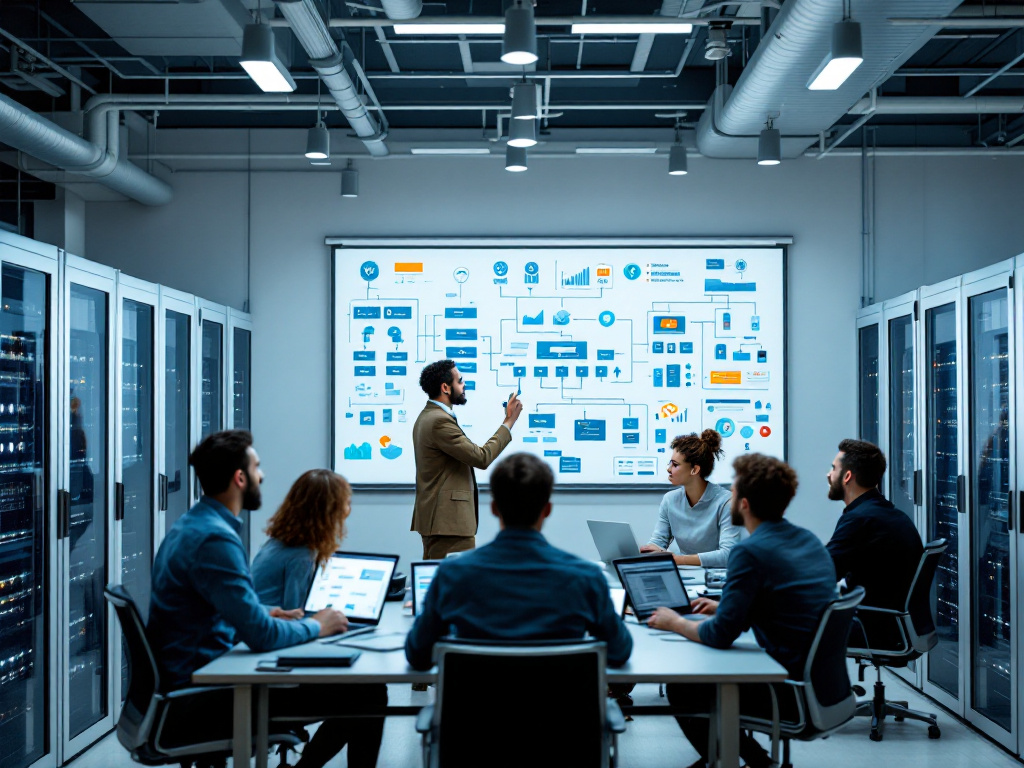
Deploying artificial intelligence (AI) can revolutionize businesses, but the journey is fraught with infrastructure challenges. From integrating specialized workloads to managing vast data volumes, these hurdles can slow down or even derail AI initiatives. This article explores the top infrastructure challenges in AI deployment and provides strategies to overcome them.
1. Integrating Specialized AI Workloads
One of the primary challenges in AI deployment is integrating specialized AI workloads into existing enterprise infrastructure. AI workloads are unique and require optimized processing, storage, and network capabilities. Deploying AI workflows on traditional enterprise storage can lead to performance issues, such as slow application speeds and intermittent failures
.
Solution:
To address this, organizations should design their AI infrastructure on a reference architecture that accounts for the specific needs of AI workloads. This includes investing in high-performance computing resources, scalable storage solutions, and robust network infrastructure
.
For example, consider a healthcare organization implementing an AI system for medical image analysis. The AI workload requires high-speed processing of large image files and real-time analysis. Traditional storage solutions may not handle the data throughput efficiently, leading to delays and inaccuracies. By investing in specialized infrastructure, such as GPU-accelerated servers and high-bandwidth network solutions, the organization can ensure that the AI system performs optimally.
Detailed Example:
Imagine a hospital that wants to use AI to analyze MRI scans for early detection of cancer. The AI model needs to process high-resolution images quickly and accurately. Traditional storage systems might struggle with the high data throughput required, leading to slow processing times and potential misdiagnoses. To overcome this, the hospital invests in a specialized AI infrastructure that includes:
- GPU-accelerated servers: These servers are designed to handle the intensive computational requirements of AI models, ensuring that images are processed in real-time.
- High-bandwidth network solutions: These solutions ensure that data is transferred quickly between different parts of the infrastructure, reducing latency and improving overall performance.
- Scalable storage solutions: These solutions can handle the large volumes of data generated by medical imaging, ensuring that the AI model has access to all the data it needs to make accurate predictions.
By implementing this specialized infrastructure, the hospital can ensure that its AI system for medical image analysis performs optimally, leading to faster and more accurate diagnoses.
2. Managing Data Volume and Quality
AI systems rely on vast amounts of data for training and inference. Managing this data volume while ensuring its quality is a significant challenge. Poor data quality can lead to inaccurate models and compromised insights
.
Solution:
Adopt a data-first strategy that prioritizes data quality and quantity from the outset. Invest in data cleaning and validation tools to ensure the accuracy and completeness of your data. Additionally, consider using data management solutions that can handle large-scale datasets efficiently
.
For instance, a retail company using AI for customer behavior analysis needs to process transaction data, customer profiles, and social media interactions. Ensuring the data is clean, accurate, and well-structured is crucial for training effective AI models. By implementing data validation tools and establishing robust data collection processes, the company can maintain high data quality and improve the accuracy of its AI insights.
Detailed Example:
Consider an e-commerce retailer that wants to use AI to personalize product recommendations for its customers. The AI model needs to analyze vast amounts of data, including customer purchase history, browsing behavior, and social media interactions. However, the data may be incomplete or inaccurate, leading to poor recommendations. To overcome this, the retailer implements a data-first strategy that includes:
- Data cleaning tools: These tools automatically identify and correct errors in the data, such as duplicate entries or missing values.
- Data validation processes: These processes ensure that the data is accurate and complete before it is used to train the AI model.
- Data management solutions: These solutions can handle large-scale datasets efficiently, ensuring that the AI model has access to all the data it needs to make accurate recommendations.
By implementing this data-first strategy, the retailer can ensure that its AI system for personalized product recommendations performs optimally, leading to increased customer satisfaction and sales.
3. Scalability Issues
As AI deployments scale, infrastructure challenges become more pronounced. Bottlenecks can appear, leading to slowed systems and underperforming applications. Scalability issues often arise from inadequate capacity planning and a lack of end-to-end system design
.
Solution:
Focus on scalability from the beginning of your AI project. Use an AI reference architecture to plan for current and future needs. Ensure that your infrastructure can handle increased workloads and integrate seamlessly with existing systems
.
For example, an e-commerce platform experiencing rapid growth may face scalability challenges with its AI-driven recommendation engine. As the user base expands, the system must handle more data and process recommendations in real-time. By designing the infrastructure with scalability in mind, such as using cloud-based solutions and distributed computing, the platform can ensure that the recommendation engine performs efficiently even under high demand.
Detailed Example:
Imagine an online marketplace that wants to use AI to provide personalized product recommendations to its customers. As the marketplace grows, the AI system needs to handle an increasing volume of data and process recommendations in real-time. However, the existing infrastructure may not be able to scale to meet these demands, leading to slow processing times and inaccurate recommendations. To overcome this, the marketplace implements a scalable infrastructure that includes:
- Cloud-based solutions: These solutions allow the marketplace to scale its infrastructure quickly and easily, ensuring that it can handle increased workloads without compromising performance.
- Distributed computing: This approach allows the marketplace to distribute the processing of recommendations across multiple servers, reducing the load on any single server and improving overall performance.
- End-to-end system design: This approach ensures that the infrastructure is designed to handle increased workloads from the outset, reducing the risk of bottlenecks and improving overall scalability.
By implementing this scalable infrastructure, the marketplace can ensure that its AI system for personalized product recommendations performs optimally, leading to increased customer satisfaction and sales.
4. Shadow AI and Governance
Shadow AI refers to AI projects that operate outside the control of the IT department, leading to security and governance issues. This can result in redundant investments and increased operational costs
.
Solution:
Establish a centralized AI infrastructure or a center of excellence to prevent shadow AI. This approach ensures standardization, economies of scale, and better governance. It also promotes collaboration and a shared understanding across teams
.
For instance, a financial institution may have multiple departments developing AI models for fraud detection, customer service, and risk assessment. Without centralized governance, these departments may use different tools and standards, leading to inefficiencies and security risks. By establishing a centralized AI infrastructure, the institution can ensure that all AI projects adhere to the same standards, reducing redundancies and improving overall governance.
Detailed Example:
Consider a bank that wants to use AI to detect fraudulent transactions. However, different departments within the bank are developing their own AI models using different tools and standards. This lack of centralized governance leads to inefficiencies, security risks, and increased operational costs. To overcome this, the bank establishes a centralized AI infrastructure that includes:
- Standardized tools and processes: These ensure that all AI projects within the bank use the same tools and follow the same processes, reducing redundancies and improving overall efficiency.
- Centralized governance: This approach ensures that all AI projects are overseen by a central authority, reducing security risks and improving overall governance.
- Collaboration and shared understanding: This approach promotes collaboration and a shared understanding across teams, ensuring that all stakeholders are aligned and working towards the same goals.
By implementing this centralized AI infrastructure, the bank can ensure that its AI system for fraud detection performs optimally, leading to increased security and operational efficiency.
5. Infrastructure Optimization
AI workloads have specific requirements that traditional enterprise infrastructure may not meet. This includes the need for high-bandwidth data throughput, low-latency processing, and massive parallelism
.
Solution:
Invest in specialized infrastructure optimized for AI workloads. This includes GPU servers, tensor processing units (TPUs), and high-performance networking solutions. Ensure that your infrastructure can support the unique demands of AI applications
.
For example, a manufacturing company implementing AI for predictive maintenance needs to process sensor data from machinery in real-time. Traditional infrastructure may not handle the high-bandwidth data throughput and low-latency processing required for accurate predictions. By investing in specialized infrastructure, such as GPU servers and high-performance networking, the company can ensure that the AI system provides timely and accurate maintenance recommendations.
Detailed Example:
Imagine a manufacturing company that wants to use AI to predict when its machinery will need maintenance. The AI model needs to process sensor data from the machinery in real-time to make accurate predictions. However, the existing infrastructure may not be able to handle the high-bandwidth data throughput and low-latency processing required, leading to inaccurate predictions and potential downtime. To overcome this, the manufacturing company implements an optimized infrastructure that includes:
- GPU servers: These servers are designed to handle the intensive computational requirements of AI models, ensuring that sensor data is processed in real-time.
- High-performance networking solutions: These solutions ensure that data is transferred quickly between different parts of the infrastructure, reducing latency and improving overall performance.
- Tensor processing units (TPUs): These specialized processors are designed to accelerate machine learning workloads, ensuring that the AI model can make accurate predictions quickly and efficiently.
By implementing this optimized infrastructure, the manufacturing company can ensure that its AI system for predictive maintenance performs optimally, leading to reduced downtime and increased operational efficiency.
6. Talent Shortage
Building and maintaining AI infrastructure requires specialized skills, including data science, machine learning engineering, and AI architecture. The demand for these skills often outstrips the supply, leading to a talent shortage
.
Solution:
Invest in training and development programs for your existing employees. Partner with universities and external service providers to access specialized expertise. Leveraging AI infrastructure solutions like edge computing and hybrid cloud can also help streamline workflows and reduce the need for manual intervention
.
For instance, a tech startup developing AI-driven personalized marketing solutions may struggle to find qualified data scientists and machine learning engineers. By investing in training programs for existing employees and partnering with universities, the startup can build a skilled workforce. Additionally, leveraging edge computing and hybrid cloud solutions can help automate workflows and reduce the reliance on manual intervention, allowing the team to focus on high-value tasks.
Detailed Example:
Consider a tech startup that wants to develop AI-driven personalized marketing solutions. However, the startup is struggling to find qualified data scientists and machine learning engineers to build and maintain the AI infrastructure. To overcome this, the startup implements a talent development strategy that includes:
- Training and development programs: These programs provide existing employees with the skills they need to build and maintain AI infrastructure, reducing the reliance on external hiring.
- Partnerships with universities: These partnerships allow the startup to access specialized expertise and recruit new talent, ensuring that it has the skills it needs to develop AI-driven solutions.
- Edge computing and hybrid cloud solutions: These solutions help automate workflows and reduce the need for manual intervention, allowing the team to focus on high-value tasks and improving overall efficiency.
By implementing this talent development strategy, the tech startup can ensure that it has the skills it needs to develop AI-driven personalized marketing solutions, leading to increased innovation and competitiveness.
7. Ethical Considerations
Ethical considerations are crucial in AI deployment. Ensuring that AI models are fair, transparent, and accountable is essential to building trust and avoiding biases
.
Solution:
Design and build your AI infrastructure with ethical principles in mind. Invest in processes and tools that promote fairness, transparency, and accountability. Regularly review and update your ethical guidelines to align with evolving standards
.
For example, a healthcare provider using AI for patient diagnosis must ensure that the AI models are unbiased and transparent. By implementing ethical guidelines and using tools that promote fairness and accountability, the provider can build trust with patients and stakeholders. Regularly reviewing and updating these guidelines ensures that the AI infrastructure aligns with the latest ethical standards and best practices.
Detailed Example:
Imagine a healthcare provider that wants to use AI to assist with patient diagnoses. However, the AI model may inadvertently perpetuate biases or make decisions that impact patients' lives without their knowledge or consent. To overcome this, the healthcare provider implements an ethical AI infrastructure that includes:
- Ethical guidelines: These guidelines ensure that the AI model is designed and implemented in a way that aligns with ethical principles such as fairness, transparency, and accountability.
- Tools for fairness and accountability: These tools help identify and mitigate biases in the AI model, ensuring that it makes fair and unbiased decisions.
- Regular reviews and updates: These ensure that the ethical guidelines and tools are up-to-date and align with the latest ethical standards and best practices.
By implementing this ethical AI infrastructure, the healthcare provider can ensure that its AI system for patient diagnosis is fair, transparent, and accountable, leading to increased trust and patient satisfaction.
8. Data Infrastructure Challenges
Integrating AI with existing data infrastructure can be challenging. Legacy systems may not be equipped to handle the data requirements of AI workloads, leading to integration issues and inefficiencies
.
Solution:
Modernize your data infrastructure to support AI workloads. Invest in data management solutions that can integrate with legacy systems and provide the necessary data throughput and processing power. Ensure that your data infrastructure is scalable and flexible to accommodate future needs
.
For instance, a logistics company implementing AI for route optimization may face challenges integrating the AI system with its existing data infrastructure. Legacy systems may not handle the real-time data processing and analysis required for optimal route planning. By modernizing the data infrastructure and investing in scalable data management solutions, the company can ensure that the AI system integrates seamlessly and provides accurate route recommendations.
Detailed Example:
Consider a logistics company that wants to use AI to optimize its delivery routes. However, the existing data infrastructure may not be able to handle the real-time data processing and analysis required for optimal route planning, leading to inefficiencies and increased costs. To overcome this, the logistics company implements a modernized data infrastructure that includes:
- Scalable data management solutions: These solutions can handle the large volumes of data generated by the logistics company's operations, ensuring that the AI model has access to all the data it needs to make accurate recommendations.
- Real-time data processing and analysis: These capabilities ensure that the AI model can process and analyze data in real-time, leading to more accurate and timely route recommendations.
- Integration with legacy systems: This ensures that the AI system can integrate seamlessly with the logistics company's existing data infrastructure, reducing the risk of integration issues and improving overall efficiency.
By implementing this modernized data infrastructure, the logistics company can ensure that its AI system for route optimization performs optimally, leading to increased efficiency and reduced costs.
9. Cost Management
Deploying AI infrastructure can be expensive, especially when investing in high-performance computing resources and scalable storage solutions. Managing costs while ensuring optimal performance is a significant challenge
.
Solution:
Optimize your AI infrastructure for cost-efficiency. Use cloud-based solutions that offer scalability and flexibility. Invest in infrastructure that can handle increased workloads without compromising performance. Regularly review and optimize your infrastructure to ensure cost-effectiveness
.
For example, a startup developing AI-driven customer service chatbots may face high infrastructure costs. By leveraging cloud-based solutions, the startup can scale its infrastructure as needed and avoid upfront capital expenditures. Regularly reviewing and optimizing the infrastructure ensures that the startup can manage costs effectively while maintaining high performance.
Detailed Example:
Imagine a startup that wants to develop AI-driven customer service chatbots. However, the high cost of deploying AI infrastructure may be a barrier to entry. To overcome this, the startup implements a cost-efficient AI infrastructure that includes:
- Cloud-based solutions: These solutions allow the startup to scale its infrastructure quickly and easily, ensuring that it can handle increased workloads without compromising performance.
- Flexible pricing models: These models ensure that the startup only pays for the resources it uses, reducing upfront capital expenditures and improving overall cost-efficiency.
- Regular reviews and optimizations: These ensure that the infrastructure is optimized for cost-efficiency, reducing waste and improving overall performance.
By implementing this cost-efficient AI infrastructure, the startup can ensure that its AI-driven customer service chatbots perform optimally, leading to increased customer satisfaction and competitiveness.
10. Collaboration and Communication
Effective AI deployment requires collaboration between IT, business, and AI teams. Miscommunication and siloed efforts can lead to delays and inefficiencies in AI projects
.
Solution:
Promote collaboration and communication across teams. Establish cross-functional project teams that include members from IT, business, and AI departments. Ensure that all stakeholders are aligned on the project goals and expectations
.
For instance, a retail company implementing AI for inventory management may face challenges due to siloed efforts between the IT, business, and AI teams. By establishing cross-functional project teams and promoting open communication, the company can ensure that all stakeholders are aligned and working towards the same goals. This collaboration helps identify and address issues early, leading to more efficient AI deployment.
Detailed Example:
Consider a retail company that wants to use AI to optimize its inventory management. However, siloed efforts between the IT, business, and AI teams may lead to miscommunication and inefficiencies, resulting in delays and increased costs. To overcome this, the retail company implements a collaborative AI deployment strategy that includes:
- Cross-functional project teams: These teams include members from IT, business, and AI departments, ensuring that all stakeholders are aligned and working towards the same goals.
- Open communication: This ensures that all stakeholders are kept informed and can provide input throughout the AI deployment process, reducing the risk of miscommunication and improving overall efficiency.
- Shared goals and expectations: These ensure that all stakeholders are aligned on the project goals and expectations, reducing the risk of delays and improving overall success.
By implementing this collaborative AI deployment strategy, the retail company can ensure that its AI system for inventory management performs optimally, leading to increased efficiency and reduced costs.
Navigating AI deployment requires overcoming significant infrastructure challenges. By addressing issues related to workload integration, data management, scalability, governance, and cost management, organizations can build robust AI infrastructure that drives innovation and delivers value. Investing in specialized infrastructure, promoting collaboration, and prioritizing ethical considerations are key to successful AI deployment.
Also read: