Modern Data Warehousing Techniques: Transforming Business Insights
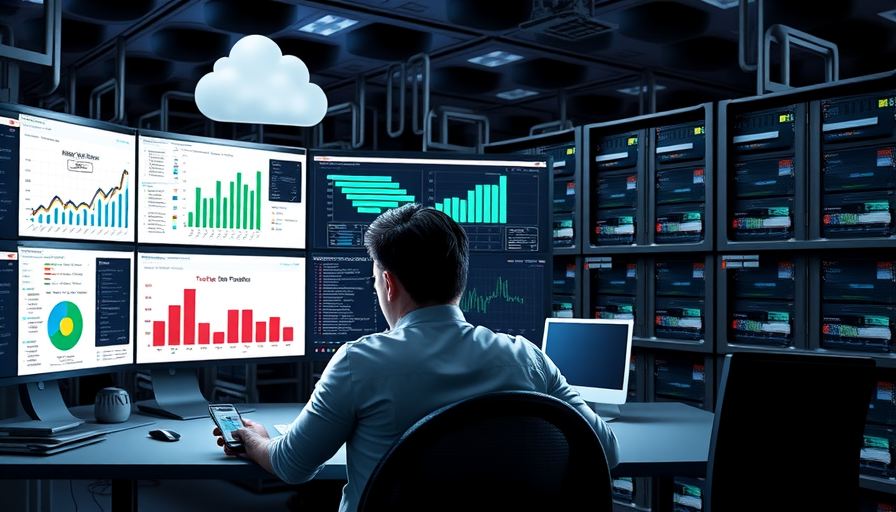
In today's data-driven world, modern data warehousing techniques are essential for organizations looking to harness the power of their data. These advanced methods enable businesses to store, manage, and analyze vast amounts of information efficiently, providing valuable insights that drive strategic decisions. This comprehensive guide delves into the latest trends, best practices, and detailed examples in data warehousing, ensuring your organization stays ahead of the curve.
The Evolution of Data Warehousing
Data warehousing has undergone significant evolution since its inception in the 1980s. Initially designed to store structured data from various sources, traditional data warehouses focused on providing a centralized repository for business intelligence and analytics. These early systems were typically built using relational databases and required substantial investment in hardware and infrastructure.
Transition to Modern Data Warehousing
The advent of big data, cloud computing, and advanced analytics technologies has revolutionized data warehousing. Modern data warehouses now encompass unstructured data as well, providing a holistic view of an organization's operations. These systems prioritize scalability, real-time processing, and seamless integration with diverse data sources.
Key Drivers of Evolution
- Data Volume and Variety: The explosion of big data has led to the need for warehouses that can handle structured, semi-structured, and unstructured data.
- Cloud Computing: Cloud-based solutions offer scalability, cost savings, and ease of access, making them an attractive option for modern data warehousing.
- Advanced Analytics: Technologies like machine learning and artificial intelligence require robust data infrastructure to support complex analytical workloads.
- Real-Time Processing: The demand for real-time insights has driven the development of systems that can process and analyze data as it is generated.
Key Features of Modern Data Warehouses
Scalability
Scalability is a critical feature of modern data warehouses, allowing organizations to expand their storage and processing capabilities as needed. This flexibility ensures that businesses can handle growing datasets without compromising performance or efficiency.
Example: Cloud-Based Scalability
Cloud-based data warehouses like Amazon Redshift automatically scale up or down based on demand. For instance, an e-commerce company experiencing a surge in traffic during the holiday season can seamlessly scale its data warehouse to accommodate increased data ingestion and processing requirements.
Real-Time Data Processing
Real-time data processing is another hallmark of modern data warehouses. By leveraging technologies like in-memory computing and streaming analytics, businesses can analyze data as it is generated, enabling quicker decision-making and improved operational agility.
Example: Financial Services
In the financial services industry, real-time fraud detection is crucial. Modern data warehousing techniques allow banks to process transaction data in real-time, identifying and mitigating fraudulent activities instantly. This capability enhances security and builds customer trust.
Integration with Big Data
Modern data warehousing seamlessly integrates with big data platforms, providing a unified environment for storing and analyzing both structured and unstructured data. This integration allows organizations to gain deeper insights from diverse datasets, fostering innovation and competitive advantage.
Example: Healthcare
In the healthcare sector, integrating electronic health records (EHRs) with genomic data can provide comprehensive patient profiles. Modern data warehouses enable healthcare providers to analyze this combined dataset, leading to personalized treatment plans and improved patient outcomes.
Top Modern Data Warehousing Techniques
Cloud-Based Solutions
Cloud-based data warehouses offer numerous benefits, including cost savings, scalability, and ease of access. Platforms like Amazon Redshift, Google BigQuery, and Azure Synapse Analytics are leading the way in providing robust, scalable solutions for modern data warehousing needs.
Example: Retail Industry
A retail company can use Google BigQuery to analyze customer purchase patterns, inventory levels, and supply chain data. The cloud-based solution allows the retailer to scale its data processing capabilities during peak seasons without investing in additional hardware.
Data Lake Architecture
Data lake architecture is a popular technique that allows organizations to store vast amounts of raw data in its native format until it is needed. This approach provides flexibility in how data is processed and analyzed, making it an ideal solution for big data environments.
Example: Telecommunications
Telecom companies can use data lakes to store call detail records (CDRs), network logs, and customer interaction data. This raw data can be analyzed to identify trends, optimize network performance, and enhance customer service.
Data Virtualization
Data virtualization enables businesses to access and integrate data from multiple sources without the need for physical data movement. This technique simplifies data management, reduces costs, and accelerates time-to-insight by providing a unified view of all relevant data.
Example: Manufacturing
In the manufacturing sector, data virtualization can integrate data from various machinery sensors, production lines, and enterprise resource planning (ERP) systems. This unified view allows manufacturers to monitor operations in real-time, identify bottlenecks, and optimize production processes.
Best Practices for Implementing Modern Data Warehousing
Focus on Data Governance
Implementing strong data governance practices is crucial for ensuring data quality, security, and compliance. By establishing clear policies and procedures, organizations can maintain the integrity of their data warehouses and build trust in their analytics capabilities.
Example: Financial Regulations
Financial institutions must comply with regulations like GDPR and CCPA. Implementing robust data governance ensures that customer data is handled securely and complies with regulatory requirements, avoiding hefty fines and reputational damage.
Leverage Automation Tools
Automation tools play a pivotal role in modern data warehousing by streamlining data ingestion, transformation, and analysis processes. These tools reduce manual effort, minimize errors, and accelerate the delivery of actionable insights.
Example: ETL Processes
Automated ETL (Extract, Transform, Load) tools like Apache NiFi can automate the process of extracting data from various sources, transforming it into a usable format, and loading it into the data warehouse. This automation ensures that data is consistently updated and ready for analysis.
Prioritize Data Security
Data security is paramount in today's digital landscape. Modern data warehouses must incorporate robust security measures to protect sensitive information from breaches and unauthorized access. Encryption, access controls, and regular audits are essential components of a secure data warehouse.
Example: Healthcare Data
Healthcare providers handle sensitive patient information that must be protected under HIPAA regulations. Implementing strong encryption, access controls, and regular security audits ensures that patient data is secure, maintaining trust and compliance.
Ensure Data Quality
Data quality is the foundation of effective data warehousing. Ensuring that data is accurate, complete, and consistent is essential for generating reliable insights and making informed decisions.
Example: Customer Data
Inaccurate or incomplete customer data can lead to poor marketing strategies and lost revenue. Implementing data quality measures like data cleansing and validation ensures that customer data is reliable, leading to effective targeting and improved customer satisfaction.
Foster a Data-Driven Culture
A data-driven culture encourages employees at all levels to use data in their decision-making processes. This culture fosters innovation, improves operational efficiency, and drives business growth.
Example: Sales Performance
In a sales organization, fostering a data-driven culture can lead to better performance tracking and incentive alignment. Sales teams can use data analytics to identify high-performing strategies, optimize resource allocation, and improve overall sales effectiveness.
Modern data warehousing techniques offer unprecedented opportunities for organizations to leverage their data effectively. By embracing scalability, real-time processing, big data integration, cloud solutions, data lakes, and data virtualization, businesses can transform their operations and gain valuable insights that drive success.
Stay ahead of the competition by adopting these modern data warehousing practices and ensuring your organization is well-prepared to navigate the complexities of today's data-driven world. Implementing strong data governance, leveraging automation tools, prioritizing data security, ensuring data quality, and fostering a data-driven culture are essential steps in building a robust and effective data warehouse.