Machine Learning Models in Predictive Maintenance for IT Infrastructure
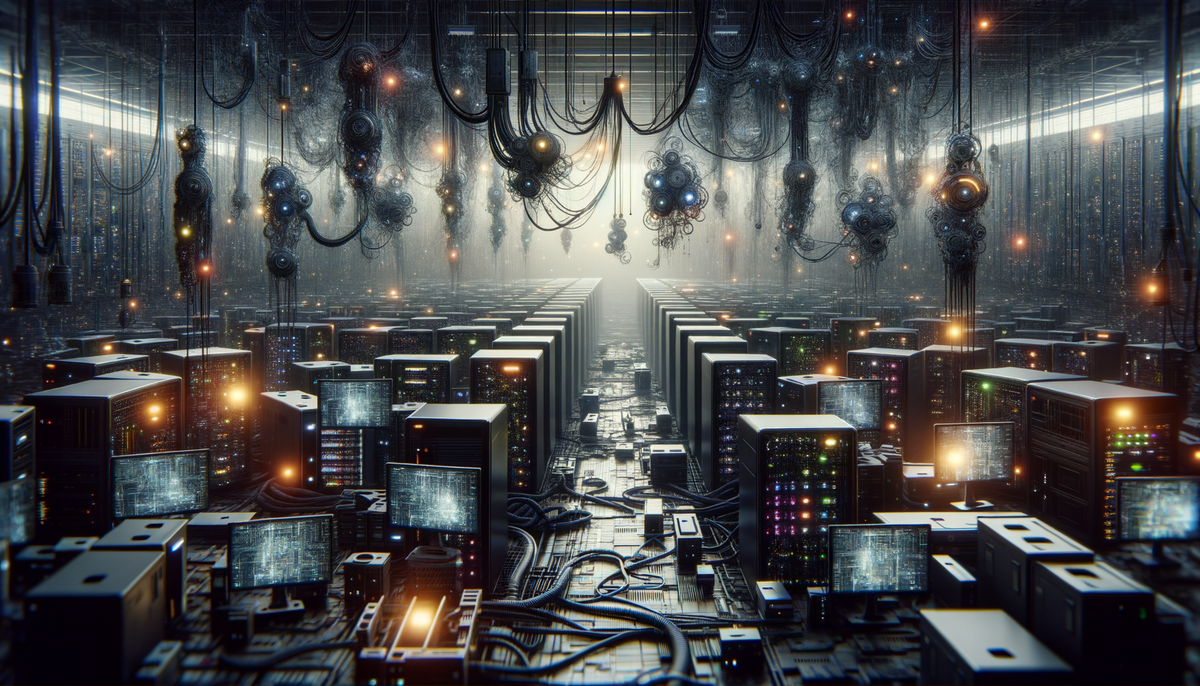
In today's fast-paced technological age, one of the biggest challenges organizations face is maintaining optimal performance of their Information Technology (IT) infrastructure. Traditional methods of maintenance are becoming increasingly outdated, cumbersome, and cost-inefficient, necessitating the shift toward smarter approaches. One such approach is Predictive Maintenance, and the use of Machine Learning (ML) models in predictive maintenance has revolutionized the management and upkeep of IT infrastructure.
The first question that arises is, what exactly is Predictive Maintenance? In simple terms, predictive maintenance refers to the use of data-driven, proactive maintenance strategies that predict when an IT infrastructure component might fail, facilitating timely intervention to prevent downtime. This contrasts sharply with traditional reactive maintenance strategies, which only address problems after they occur.
The Role of Machine Learning in Predictive Maintenance
Machine Learning, a subset of Artificial Intelligence (AI), provides systems the ability to automatically learn and improve from experience without being explicitly programmed. In the context of IT infrastructure, ML models can analyze vast amounts of data related to the operation of various infrastructure components, spot trends, and predict problems in advance, even before humans can detect them.
For instance, Supervised Learning models - one of the types of machine learning models - can be trained to recognize patterns that indicate potential issues. They use a labeled dataset, where each record includes input data and a desired outcome or prediction. With enough training data, these models can then predict the chances of infrastructure failure based on the patterns they discern from the data.
Unsupervised Learning algorithms, on the other hand, are useful in situations where sufficient labeled training data isn't available. These can identify anomalies or outliers in infrastructure operations which can signify potential issues.
Finally, Reinforcement Learning, where an agent makes decisions by interacting with its Environment to achieve a goal, has shown promise as an approach for managing IT systems. The agent tries various actions, gets rewarded or penalized depending upon the result, and learns what action to take under what circumstances.
Key Benefits of Using ML models in IT Infrastructure Maintenance
The benefits of implementing Machine Learning models in predictive maintenance for IT infrastructure maintenance are many:
- Reduce Equipment Downtime: By predicting issues before they occur, organizations can perform maintenance activities proactively preventing unexpected downtime.
- Save Costs: Preventing problems before they happen saves the organization from potential financial losses associated with unplanned downtime and expensive emergency repairs.
- Maximize Resources: Resources can be optimally allocated and utilized because maintenance can be performed when IT is actually needed, rather than on a routine basis regardless of the actual state of the equipment.
- Improve productivity: Predictive maintenance reduces the time spent on unnecessary maintenance, freeing up resources to focus on more strategic tasks.
Implementing Machine Learning models for predictive maintenance in IT infrastructure has a huge potential. By analyzing vast amounts of data and identifying patterns of failures, machine learning can predict issues before they occur. As more organizations realize the power of predictive maintenance, IT’s clear that machine learning will play an increasingly vital role in the future of IT infrastructure maintenance.