Machine Learning for Data Center Cooling Optimization
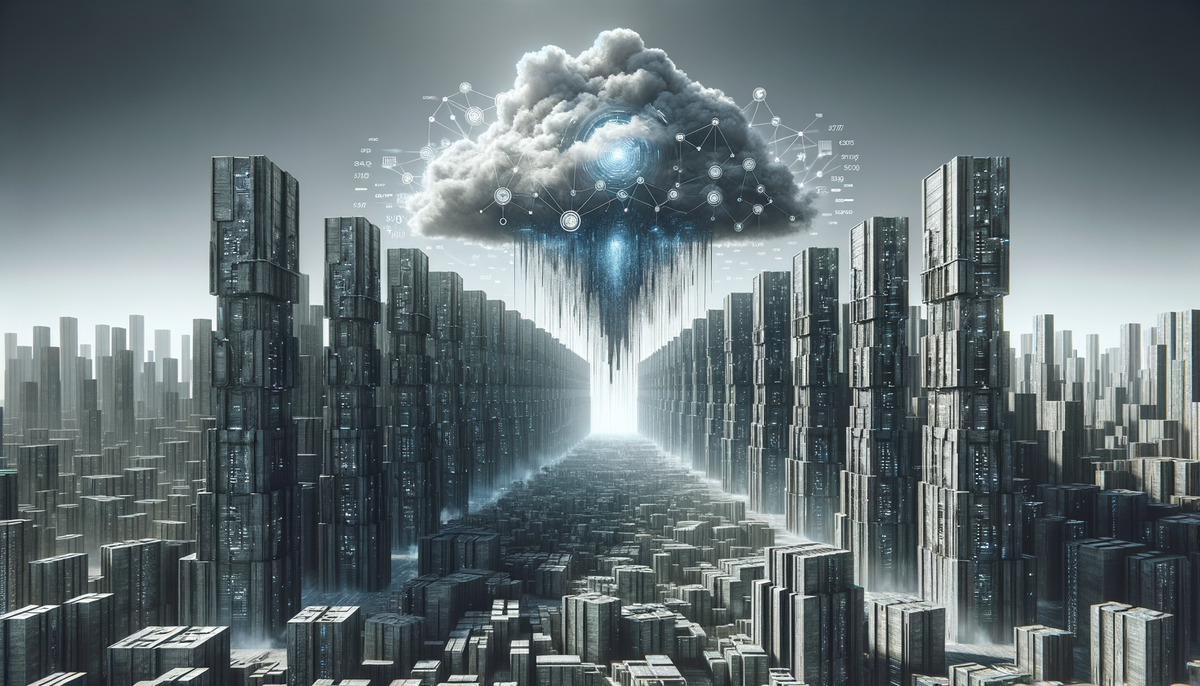
In today's digital era, data centers act as the backbone of the internet, Cloud Computing, and countless digital applications. As the global demand for data processing and storage expands exponentially, so too does the energy consumption of data centers. A significant component of this energy consumption comes from cooling systems, which are vital for maintaining optimal operating temperatures and ensuring hardware longevity. Enter machine learning, a transformative technology poised to revolutionize data center cooling optimization, boosting efficiency and sustainability.
Understanding the Importance of data Center Cooling
Effective cooling is indispensable for data centers. Servers, storage units, and networking equipment generate tremendous amounts of heat during operation. Without adequate cooling measures, these devices can overheat, leading to performance degradation, equipment failure, or even catastrophic data loss.
The traditional approaches to data center cooling often involve fixed protocols that operate continuously at maximum power to ensure safety. While these methods are reliable, they are typically inefficient and costly. They fail to adapt to dynamic changes in workload, environmental conditions, and technological advancements. This is where machine learning algorithms offer a solution.
How Machine Learning Transforms Cooling Systems
Machine learning, a subset of artificial intelligence, involves the use of algorithms that enable systems to learn from historical data, identify patterns, and make informed decisions with minimal human intervention. Here are some ways machine learning enhances data center cooling optimization:
1. Predictive Analytics
machine learning models can predict potential temperature spikes or cooling requirements by analyzing past and real-time data from sensors throughout the data center. Predictive analytics helps in preemptively adjusting the cooling systems, preventing overheating and reducing energy expenditure.
2. Dynamic Adjustment
Traditional cooling systems might operate uniformly across the data center, which can lead to unnecessary cooling and energy use. machine learning models can dynamically adjust cooling levels in different zones based on the real-time workload and temperature data. This zoning approach targets specific areas that need cooling, optimizing energy use.
3. Fault Detection and Prevention
machine learning algorithms can identify unusual patterns that signify equipment faults or inefficiencies, enabling the early detection of potential issues before they culminate into serious problems. By rapidly diagnosing these anomalies, data centers can minimize downtime and maintenance costs.
4. energy consumption Reduction
Energy efficiency is not only environmentally responsible but also economically beneficial. By optimizing cooling processes, machine learning can significantly reduce the power consumption of data centers. This reduction decreases both Operational Costs and the carbon footprint associated with ever-growing digital infrastructure.
Implementing Machine Learning in Cooling Systems
Now that we understand the potential benefits of machine learning in optimizing data center cooling, the question becomes: how can these solutions be effectively implemented?
1. data Collection
The foundation of any machine learning model is data. For cooling optimization, vast amounts of data from various sensors are collected, including temperature, humidity, power usage, and airflow rates. Ensuring data quality and relevance is crucial to creating reliable models.
2. Selecting the Right Algorithms
Several machine learning algorithms can be applied to cooling systems, from simple linear regression models to more complex neural networks. The choice of the algorithm largely depends on the quality and quantity of available data, as well as the specific objectives of the optimization.
3. Continual Learning
data centers are dynamic environments with constantly changing workloads and conditions. As such, machine learning models require continuous updates and learning to maintain their effectiveness. Implementing a feedback loop enables these systems to adapt to changes in real-time.
4. integration with Existing Systems
For seamless implementation, machine learning solutions must integrate with existing cooling and monitoring systems within data centers. This may involve updating or replacing current infrastructure, which should be approached strategically to minimize disruptions during the transition.
case studies: Success Stories in Cooling optimization
Several industry leaders have harnessed the power of machine learning to optimize their data center cooling processes effectively. Let's explore a few notable examples:
Google has been at the forefront of integrating machine learning into its data centers. By leveraging DeepMind's AI, Google managed to reduce the energy used for cooling by an impressive 40%. The system uses neural networks trained on data from thousands of sensors to predict and control cooling systems autonomously, reducing Operational Costs and the Environmental impact.
Microsoft
Microsoft's data centers have also benefited from machine learning optimization. They implemented AI systems that monitor and analyze cooling efficiencies across their facilities, helping to decrease their PUE (Power Usage Effectiveness) and enhance sustainability. This move not only optimizes operation expenditure but also aligns with Microsoft's commitment to carbon neutrality.
The Future of data Center Cooling with Machine Learning
As machine learning technologies advance, their application in data center cooling optimization is likely to become even more sophisticated and impactful. future trends may include:
-
Enhanced Real-time Performance: As data processing capabilities improve, real-time predictive analytics and adjustments will become increasingly precise and instantaneous.
-
Edge computing integration: With the rise of edge computing, machine learning models could operate closer to data sources, reducing latency, and providing faster insights.
-
Increased automation: The path towards fully autonomous data centers is becoming clearer with machine learning advancements. Greater automation will allow data centers to self-regulate airflows, temperatures, and responses to equipment failures without human intervention.
-
Sustainability and compliance: As global environmental concerns rise, regulatory standards for data center efficiency and emissions may become stricter. Machine learning optimization could be essential for compliance and sustainability goals.
Conclusion
Machine learning for data center cooling optimization represents a significant leap towards more energy-efficient, cost-effective, and sustainable digital infrastructure management. As technology continues to evolve, the possibilities for Enhancing data center operations are vast. Embracing these advanced Techniques will be crucial for industry leaders aiming to minimize their environmental footprint while maintaining high-performance standards. The integration of machine learning into cooling systems not only conserves resources but also future-proofs data centers against emerging challenges in an increasingly digital world.