How Does Infrastructure Impact AI Performance?
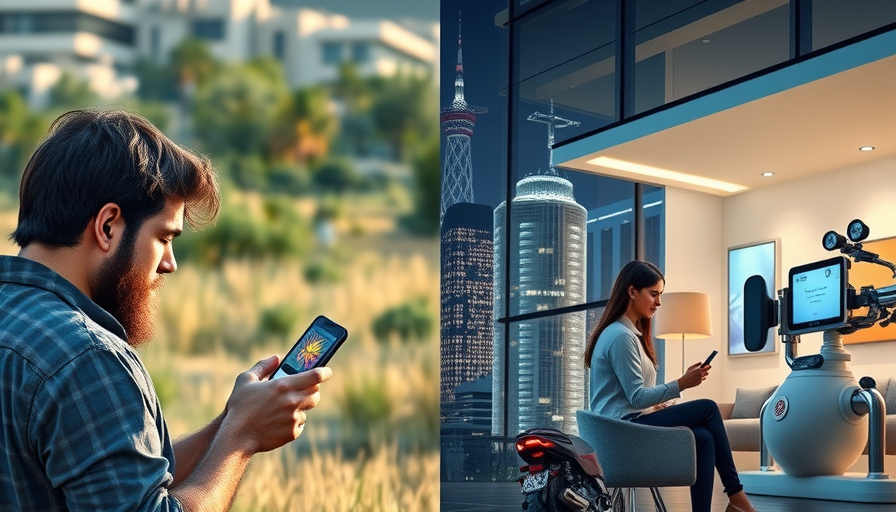
Artificial Intelligence (AI) has revolutionized various industries by enabling machines to perform tasks that typically require human intelligence. From healthcare and finance to retail and manufacturing, AI applications are transforming business operations and customer experiences. However, the performance of AI systems is heavily influenced by the underlying infrastructure. Understanding how infrastructure impacts AI performance is crucial for businesses looking to leverage AI effectively. This comprehensive guide delves into the key aspects of AI infrastructure and provides detailed examples to illustrate their importance.
The Role of Infrastructure in AI Performance
Hardware Specifications
The hardware specifications of your infrastructure play a significant role in determining AI performance. High-performance processors, such as GPUs (Graphics Processing Units) and TPUs (Tensor Processing Units), are essential for handling the computational demands of AI algorithms. These components accelerate data processing, enabling faster training and inference times.
Central Processing Units (CPUs)
While CPUs are versatile and can handle a wide range of tasks, they are not optimized for the parallel processing required by many AI algorithms. CPUs excel in sequential processing, making them suitable for tasks that involve single-threaded execution. However, for AI workloads that require massive parallelism, such as training deep learning models, CPUs may struggle to keep up.
Example: Training a convolutional neural network (CNN) on a large dataset might take days on a CPU due to its sequential processing limitations. In contrast, a GPU can perform the same task in hours by leveraging thousands of cores that work in parallel.
Graphics Processing Units (GPUs)
GPUs are designed for parallel processing, making them ideal for AI tasks that involve matrix and vector operations. NVIDIA's CUDA platform, for instance, allows developers to write parallel code that runs efficiently on GPUs. This makes GPUs well-suited for training deep learning models and performing real-time inference.
Example: NVIDIA's A100 GPU is commonly used in data centers for AI training and inference. Its Tensor Cores can perform mixed-precision training, significantly speeding up the process compared to traditional CPUs.
Tensor Processing Units (TPUs)
Developed by Google, TPUs are custom-built accelerators designed specifically for machine learning workloads. TPUs excel at performing the matrix multiplications that are fundamental to neural network operations. They offer high throughput and energy efficiency, making them a popular choice for large-scale AI deployments.
Example: Google's Cloud TPU v4 pods can provide up to 1 exaflop of AI performance, enabling researchers to train massive models like Transformers on extensive datasets in a fraction of the time it would take on traditional hardware.
Storage Solutions
Efficient storage solutions are vital for managing large datasets required by AI models. AI infrastructure needs to support high-speed access to data, which is why SSDs (Solid State Drives) and NVMe (Non-Volatile Memory Express) storage options are preferred over traditional HDDs (Hard Disk Drives). These storage technologies provide faster read/write speeds, ensuring that AI applications can process data quickly.
Solid State Drives (SSDs)
SSDs use flash memory to store data, providing faster access times and higher throughput compared to traditional HDDs. This makes SSDs ideal for AI workloads that require frequent reads and writes of large datasets.
Example: A data scientist working on natural language processing (NLP) might need to frequently access a large corpus of text data. Using an SSD instead of an HDD can significantly reduce the time it takes to load this data into memory, speeding up the training process.
Non-Volatile Memory Express (NVMe)
NVMe is a protocol designed to take full advantage of the capabilities of modern SSDs. It provides lower latency and higher throughput compared to traditional storage interfaces like SATA. NVMe drives are particularly well-suited for AI workloads that require high-speed data access.
Example: In a recommendation system, real-time personalization requires fast access to user behavior data. NVMe drives can provide the low-latency storage needed to retrieve and process this data in real-time, ensuring a seamless user experience.
Networking
Network latency and bandwidth are critical factors in AI performance. High-speed networking infrastructure ensures seamless communication between different components of the AI system, including servers, GPUs, and storage devices. Low-latency networks minimize delays, improving the overall responsiveness of AI applications.
Local Area Networks (LANs)
In a data center or on-premises setup, a high-performance LAN is essential for ensuring fast communication between AI nodes. Technologies like InfiniBand and Ethernet with Remote Direct Memory Access (RDMA) can provide low-latency, high-throughput networking.
Example: In a distributed training setup, multiple GPUs might be used to train a large model in parallel. A high-speed LAN ensures that these GPUs can communicate efficiently, reducing the time it takes to synchronize gradients and update model parameters.
Wide Area Networks (WANs)
For AI applications that span multiple geographic locations, a reliable WAN is crucial. Technologies like Software-Defined WAN (SD-WAN) can optimize network performance by dynamically routing traffic over the best available paths.
Example: A global e-commerce company might use AI for real-time fraud detection across its international operations. An optimized WAN ensures that data from different regions can be processed and analyzed in real-time, improving the accuracy and responsiveness of the fraud detection system.
Scalability and Flexibility
Scalable AI infrastructure allows businesses to handle increasing volumes of data and more complex AI models without performance degradation. Cloud-based solutions offer scalability by providing on-demand resources, making it easier for organizations to scale their AI operations as needed.
Horizontal vs. Vertical Scaling
Horizontal scaling involves adding more nodes to a system to distribute the workload, while vertical scaling involves upgrading existing hardware to handle more load. Both approaches have their advantages and can be combined to achieve optimal scalability.
Example: A startup developing an AI-powered chatbot might start with a single server for development and testing. As user demand grows, they can horizontally scale by adding more servers to handle increased traffic. Simultaneously, they might vertically scale by upgrading the CPUs and GPUs on existing servers to improve performance.
Cloud vs. On-Premises Infrastructure
Both cloud and on-premises infrastructures have their advantages when it comes to AI performance. Cloud platforms like AWS, Google Cloud, and Azure provide scalable computing power, storage, and networking capabilities. However, on-premises solutions offer more control over data security and compliance, which can be crucial for industries with strict regulatory requirements.
Cloud Infrastructure
Cloud providers offer a range of services tailored for AI workloads, including managed GPU instances, high-performance storage, and optimized networking. These services allow businesses to focus on developing AI applications without worrying about the underlying infrastructure.
Example: AWS provides EC2 instances with NVIDIA GPUs that are optimized for deep learning tasks. These instances come pre-installed with popular machine learning frameworks like TensorFlow and PyTorch, making it easy for developers to get started with AI projects.
On-Premises Infrastructure
On-premises infrastructure offers greater control over data security and compliance, which is essential for industries like healthcare and finance. Organizations can also customize their on-premises setup to meet specific performance requirements.
Example: A financial institution might use an on-premises AI system for real-time fraud detection. By keeping the data on-site, they can ensure that sensitive information is protected and compliant with regulatory standards. They can also optimize the hardware configuration to achieve the lowest possible latency for real-time processing.
Monitoring and Optimization
Regular monitoring of AI infrastructure is essential for maintaining optimal performance. Tools that provide real-time insights into system performance help identify bottlenecks and areas for improvement. AI infrastructure optimization involves tuning hardware and software configurations to maximize efficiency and reduce latency.
Performance Monitoring Tools
Performance monitoring tools like Prometheus, Grafana, and Datadog can provide real-time metrics on CPU usage, memory consumption, network traffic, and storage I/O. These tools help identify performance bottlenecks and optimize resource allocation.
Example: A data science team might use Grafana to monitor the performance of their AI training jobs. By visualizing key metrics like GPU utilization and training time, they can identify inefficiencies and make adjustments to improve overall performance.
Infrastructure Optimization Techniques
Infrastructure optimization involves tuning hardware and software configurations to maximize efficiency. This can include techniques like load balancing, resource allocation, and network optimization.
Example: In a multi-tenant AI platform, load balancing can distribute incoming requests across multiple servers to ensure even utilization and prevent bottlenecks. Resource allocation strategies like Kubernetes' autoscaling can dynamically adjust the number of pods based on demand, ensuring optimal performance.
Security Considerations
Securing your AI infrastructure is paramount to protecting sensitive data and ensuring the reliability of AI applications. Robust security measures, including encryption, access controls, and regular updates, are necessary to safeguard against cyber threats.
Data Encryption
Encrypting data at rest and in transit is essential for protecting sensitive information. Technologies like TLS (Transport Layer Security) and AES (Advanced Encryption Standard) can ensure that data is secure both during storage and transmission.
Example: A healthcare provider using AI for diagnostic imaging might encrypt patient data to comply with HIPAA regulations. By using TLS for data in transit and AES for data at rest, they can ensure that sensitive medical information is protected from unauthorized access.
Access Controls
Implementing strict access controls is crucial for preventing unauthorized access to AI systems. Role-based access control (RBAC) and multi-factor authentication (MFA) can help ensure that only authorized users have access to critical resources.
Example: In a financial institution, RBAC can be used to restrict access to the AI fraud detection system based on job roles. Only data scientists and security analysts with appropriate permissions can access and modify the system, reducing the risk of insider threats.
Regular Updates and Patching
Regularly updating and patching AI infrastructure is essential for addressing vulnerabilities and ensuring system reliability. Automated patch management tools can help streamline this process, ensuring that all components are up-to-date.
Example: A cloud-based AI service might use automated patch management to keep its virtual machines and containers updated with the latest security patches. This ensures that the service remains secure against known vulnerabilities and maintains high availability.
Understanding how infrastructure impacts AI performance is key to building efficient and effective AI systems. By investing in high-performance hardware, scalable storage solutions, robust networking infrastructure, and reliable cloud or on-premises setups, businesses can optimize their AI operations for better results. Regular monitoring and security measures further enhance the reliability and performance of AI applications.
In summary, the following key factors significantly influence AI performance:
- Hardware Specifications: High-performance processors like GPUs and TPUs are essential for handling the computational demands of AI algorithms.
- Storage Solutions: Fast storage technologies like SSDs and NVMe ensure quick data access, which is crucial for AI workloads.
- Networking: Low-latency, high-throughput networks enable seamless communication between AI components, improving overall responsiveness.
- Scalability: Cloud-based solutions offer scalable resources, making it easier to handle increasing volumes of data and more complex models.
- Monitoring and Optimization: Regular monitoring helps identify bottlenecks, while optimization techniques maximize efficiency and reduce latency.
- Security Measures: Robust security measures protect sensitive data and ensure the reliability of AI applications.
By carefully considering these factors, businesses can build a robust AI infrastructure that supports high-performance AI applications and drives innovation across industries.