Federated Learning with Decentralized AI
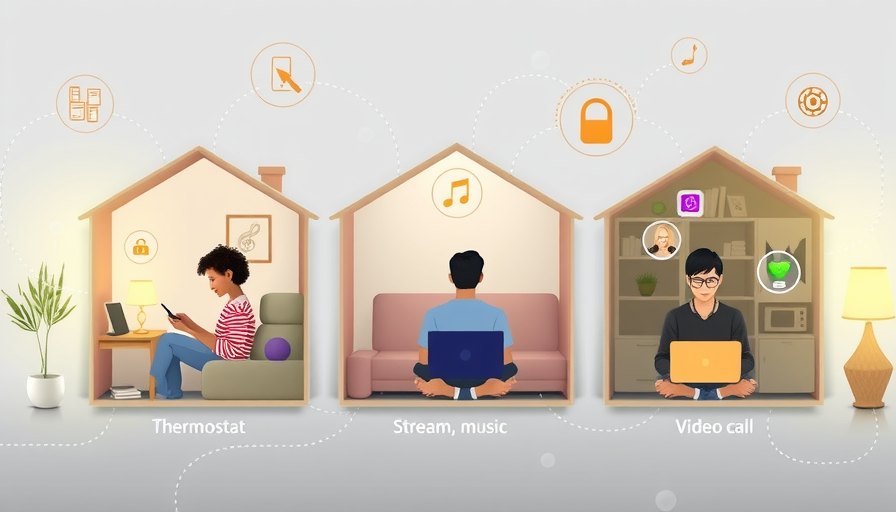
In today's digital age, data privacy and security have become paramount concerns. Traditional machine learning models often rely on centralized datasets, which can pose significant risks to user privacy. This is where federated learning comes into play, particularly when combined with decentralized AI. By leveraging these technologies, organizations can train machine learning models without compromising sensitive data.
What is Federated Learning?
Federated learning is a collaborative approach to training machine learning models that keeps user data decentralized. Instead of transferring raw data to a central server, models are trained on local devices and only the model updates (not the raw data) are shared with a centralized server or among other devices in a peer-to-peer network. This method ensures that sensitive information remains private.
Key Benefits of Federated Learning
- Enhanced Data Privacy: Since user data never leaves the device, federated learning provides a robust solution for maintaining data privacy.
- Reduced Data Transmission Costs: By minimizing the amount of data transferred over networks, federated learning can significantly reduce transmission costs.
- Improved Scalability: Federated learning allows models to be trained on a vast number of devices simultaneously, making it highly scalable.
How Federated Learning Works
Federated learning typically involves the following steps:
- Initialization: A central server initializes a global model and distributes it to all participating devices.
- Local Training: Each device trains the model on its local data. This step involves forward and backward propagation to update the model parameters.
- Model Update: After training, each device sends the model updates (usually the gradients or weight differences) back to the central server.
- Aggregation: The central server aggregates these updates using a technique like Federated Averaging to create an improved global model.
- Distribution: The updated global model is sent back to all devices for another round of training.
Example: Federated Learning in Healthcare
Consider a scenario where multiple hospitals want to collaborate on developing a predictive model for disease diagnosis but cannot share patient data due to privacy regulations. Each hospital can train the model locally using its own patient data and only share the model updates with a central server. The central server aggregates these updates to create an improved global model, which is then distributed back to all hospitals. This way, hospitals can benefit from collaborative learning without compromising patient privacy.
The Role of Decentralized AI
Decentralized AI complements federated learning by distributing the computational power and decision-making across multiple nodes rather than relying on a single central server. This decentralization enhances robustness and resilience, making the system less vulnerable to failures or attacks.
Key Benefits of Decentralized AI
- Improved Robustness: By distributing computations, decentralized AI reduces the risk of single points of failure.
- Enhanced Security: Decentralized systems are more resistant to certain types of cyberattacks, as there is no central point of control.
- Scalability: Decentralized AI can scale efficiently by adding more nodes to the network.
How Federated Learning Works with Decentralized AI
- Local Model Training: Each device trains its own model using local data.
- Model Updates Sharing: Only the updates (not the raw data) are shared with a central aggregator or with other devices in a peer-to-peer network.
- Aggregation and Distribution: The central aggregator or decentralized nodes combine these updates to create an improved global model, which is then distributed back to all devices.
Example: Decentralized AI in Autonomous Vehicles
In the context of autonomous vehicles, each car can act as a node in a decentralized network. Each car trains its own model based on its driving experiences and shares only the model updates with other cars or a central aggregator. This way, the fleet of cars can collectively improve their driving algorithms without relying on a central server, enhancing both privacy and security.
Real-World Applications
Federated learning with decentralized AI has numerous applications across various industries:
Healthcare
- Medical Research: Hospitals can collaborate on medical research without sharing sensitive patient data.
- Predictive Analytics: Develop models for predicting disease outbreaks or patient outcomes based on aggregated data from multiple sources.
Finance
- Fraud Detection: Banks can develop fraud detection models using customer transaction data without compromising privacy.
- Credit Scoring: Create more accurate credit scoring models by leveraging data from multiple financial institutions while maintaining data privacy.
Autonomous Vehicles
- Safety Improvements: Cars can learn from each other's driving experiences to improve safety and efficiency.
- Traffic Management: Develop models for predicting traffic patterns and optimizing routes based on aggregated data from multiple vehicles.
Smart Cities
- Energy Management: Optimize energy consumption in smart cities by aggregating data from various IoT devices without compromising user privacy.
- Waste Management: Improve waste management systems by analyzing data from smart bins across the city while maintaining data privacy.
Challenges and Solutions
Despite its advantages, federated learning with decentralized AI faces several challenges:
Communication Efficiency
Ensuring efficient communication between devices and servers is crucial for minimizing latency and energy consumption. Techniques such as differential privacy can be used to add noise to model updates, making it difficult for adversaries to infer sensitive information from the shared updates.
Model Security
Protecting model updates from adversarial attacks is essential to maintain the integrity of the training process. Secure multi-party computation (SMPC) techniques can be employed to ensure that model updates are aggregated securely without revealing individual updates.
Heterogeneity
Devices in a federated learning system may have different hardware capabilities and data distributions, leading to challenges in model convergence. Techniques such as personalized federated learning can address this by allowing each device to maintain its own personalized model while benefiting from the global model.
Example: Addressing Communication Efficiency
Consider a scenario where a network of smart sensors is used for environmental monitoring. Each sensor collects data locally and trains a model to detect anomalies. To ensure efficient communication, differential privacy techniques can be applied to add noise to the model updates before they are shared with a central aggregator. This way, the system can maintain data privacy while minimizing the amount of data transmitted over the network.
Advanced Concepts in Federated Learning
Federated Transfer Learning
Federated transfer learning extends federated learning by leveraging pre-trained models on related tasks. In this approach, devices first train a model on a related task and then fine-tune it on their local data. This can be particularly useful when devices have limited data for training.
Example: Federated Transfer Learning in Retail
In the retail industry, stores can use federated transfer learning to develop recommendation systems. Each store can leverage a pre-trained model from another store with similar customer behavior and fine-tune it using its own local data. This way, stores can benefit from collaborative learning without compromising customer privacy.
Federated Reinforcement Learning
Federated reinforcement learning combines federated learning with reinforcement learning to enable decentralized decision-making in dynamic environments. In this approach, each device learns a policy based on its local interactions and shares only the policy updates with other devices or a central aggregator.
Example: Federated Reinforcement Learning in Robotics
In robotics, federated reinforcement learning can be used to train robots to perform complex tasks in collaborative environments. Each robot can learn a policy based on its own experiences and share only the policy updates with other robots. This way, the fleet of robots can collectively improve their performance without relying on a central server.
Federated learning with decentralized AI represents a significant leap forward in maintaining data privacy while advancing machine learning capabilities. As more organizations adopt this approach, we can expect to see innovative solutions that prioritize user privacy without sacrificing the benefits of AI-driven insights. By addressing challenges such as communication efficiency, model security, and heterogeneity, federated learning with decentralized AI can pave the way for a more secure and privacy-preserving future.
Stay tuned for more updates on how federated learning and decentralized AI are shaping the future of technology!