Explainable AI (XAI): What Businesses Need to Know
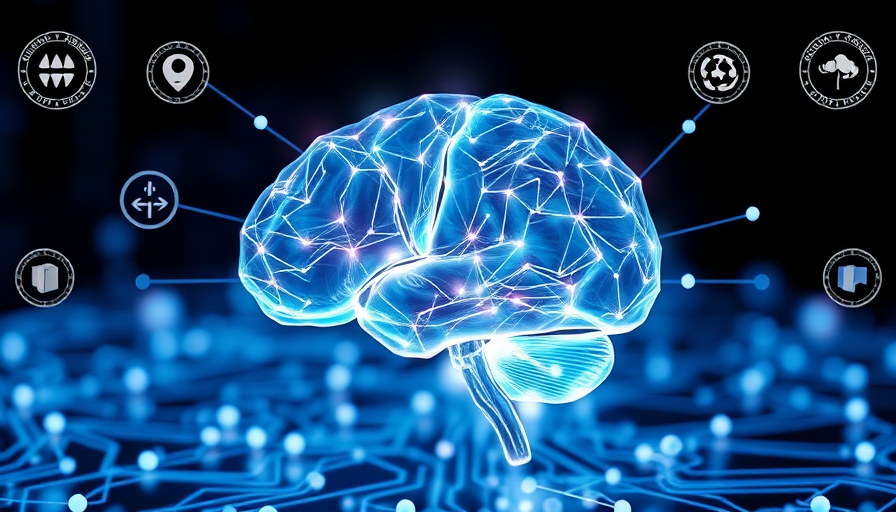
In today's rapidly evolving digital landscape, artificial intelligence (AI) has emerged as a transformative force for businesses across industries. From Enhancing customer experiences to optimizing supply chains and driving strategic decision-making, AI is revolutionizing the way organizations operate. However, as AI systems become more complex and integrated into critical business processes, the need for explainable AI (XAI) has grown significantly. But what exactly is XAI, and why should your business prioritize IT? This comprehensive guide will delve into the intricacies of explainable AI, its importance, benefits, implementation strategies, and future trends.
What is Explainable AI (XAI)?
Explainable AI refers to artificial intelligence systems designed to provide clear, understandable explanations for their decisions and actions. Unlike traditional black-box models—where the internal workings are opaque and difficult to interpret—XAI aims to demystify AI processes, making them transparent and comprehensible to human users. This transparency is achieved through various Techniques that shed light on how AI systems process data and arrive at conclusions.
Key Concepts in Explainable AI
-
Interpretability: This refers to the ability of an AI model to be understood by humans. Interpretability can be global (understanding the overall behavior of the model) or local (understanding specific predictions).
-
Transparency: Transparency involves making the internal mechanisms of an AI system visible and understandable. IT includes both the data used and the processes applied to that data.
-
Explainability: Explainability is the capacity of an AI system to provide explanations for its decisions in a way that humans can understand. This often involves generating human-readable descriptions or visualizations of the decision-making process.
-
Accountability: Accountability ensures that AI systems can be held responsible for their actions and decisions. This is crucial for building trust and ensuring ethical use of AI.
Types of Explainable AI Techniques
Several Techniques can be employed to make AI models more explainable:
-
Model-Agnostic Methods: These methods can be applied to any type of model without needing access to its internal workings. Examples include LIME (Local Interpretable Model-agnostic Explanations) and SHAP (SHapley Additive exPlanations).
-
Model-Specific Methods: These Techniques are tailored to specific types of models, such as decision trees or Neural Networks. For example, feature importance in tree-based models or saliency maps in Neural Networks.
-
Intrinsic Explainability: Some models are inherently more explainable than others. For instance, linear regression models are easier to interpret because they involve simple mathematical equations.
Why Businesses Need Explainable AI
Incorporating explainable AI into your business strategy can offer a myriad of benefits that go beyond mere compliance with Regulations. Here's an in-depth look at why XAI is essential for modern businesses:
1. Enhanced Transparency
One of the primary advantages of XAI is enhanced transparency. In complex systems, understanding how AI arrives at its conclusions is crucial for ensuring fairness and mitigating biases. This level of clarity is particularly important in industries like finance, healthcare, and legal services, where decisions can significantly impact individuals' lives.
Example: In the financial sector, an explainable credit scoring model can provide clear reasons why a loan application was approved or denied. This transparency helps borrowers understand the decision-making process and take corrective actions if necessary.
2. Regulatory compliance
Many regulatory bodies now require that AI systems be explainable to ensure fairness and accountability. For instance, the General data Protection regulation (GDPR) in Europe mandates that individuals have the right to know why certain decisions were made about them. Explainable AI helps businesses meet these compliance requirements without compromising performance.
Example: Under GDPR, companies must be able to explain how an individual's personal data was used to make a decision, such as whether they qualify for a mortgage or insurance policy. XAI Tools can generate these explanations automatically.
3. Improved Decision-Making
Transparent AI systems allow businesses to identify and correct biases, leading to more accurate and reliable decision-making processes. This can result in better outcomes for customers and stakeholders, fostering trust and loyalty.
Example: In healthcare, an explainable diagnostic tool can help doctors understand why a particular diagnosis was suggested by the AI system. This transparency enables doctors to confirm or challenge the AI's recommendations based on their expertise.
4. Customer Trust
Customers are becoming increasingly aware of the role AI plays in their interactions with businesses. By using explainable AI, companies can build customer trust by demonstrating that their systems are fair, transparent, and accountable.
Example: An e-commerce platform using explainable recommendation algorithms can provide customers with clear explanations for product suggestions, Enhancing trust and satisfaction.
5. risk management
In industries where risk management is critical, such as finance and insurance, XAI helps in identifying potential risks and understanding their sources. This insight allows businesses to mitigate risks effectively and make more informed decisions.
Example: An insurer using explainable AI can better understand the factors contributing to a high-risk profile for a customer, enabling them to offer tailored solutions or adjust policies accordingly.
Implementing Explainable AI
To implement XAI effectively, businesses need to consider several key factors and follow a structured approach. Here’s a comprehensive guide:
1. Assessing Business Needs
Before diving into implementation, IT's essential to assess your business needs and identify areas where explainability can add the most value.
- Stakeholder analysis: Identify stakeholders who will benefit from XAI and understand their expectations.
- Regulatory Requirements: Review relevant Regulations and standards that require or encourage the use of explainable AI.
- risk assessment: Evaluate the risks associated with using black-box models in your industry and how XAI can mitigate them.
2. Choosing the Right Tools
Selecting the right Tools and frameworks is crucial for successful explainable AI implementation. Here are some popular options:
- LIME (Local Interpretable Model-agnostic Explanations): LIME approximates a complex model locally with an interpretable model to explain individual predictions.
- SHAP (SHapley Additive exPlanations): SHAP values provide a unified measure of feature importance based on game theory.
- Anchor Points: This technique identifies necessary conditions for a prediction, making IT easier to understand the decision-making process.
- Counterfactual Explanations: These explanations show what changes in input features would result in a different outcome, helping users understand the boundaries of the model's decisions.
3. data Preparation
High-quality data is the foundation of any successful AI implementation, including XAI. Ensure your data is:
- Clean and Preprocessed: Remove duplicates, handle missing values, and normalize data as needed.
- Relevant: Use features that are directly related to the problem you're trying to solve.
- Diverse: Include a variety of examples to capture different scenarios and edge cases.
4. Model training
When training your AI models, consider Techniques that promote explainability:
- Simpler Models: Prefer simpler models like decision trees or linear regression when possible, as they are inherently more interpretable.
- Regularization: Use regularization Techniques to prevent overfitting and improve model transparency.
- Feature Engineering: Carefully select and engineer features that contribute positively to the model's explainability.
5. Evaluation and Validation
Evaluate your XAI models using appropriate metrics and validation Techniques:
- accuracy vs. Explainability Trade-Off: Balance model accuracy with explainability, as highly accurate but opaque models may not be suitable for all applications.
- User Testing: Conduct user testing to ensure that explanations are understandable and actionable.
- Bias Detection: Use Tools to detect and mitigate biases in your AI models.
6. continuous monitoring and Evaluation
Regularly monitor and evaluate your explainable AI systems to ensure they remain transparent, fair, and effective over time:
- Performance Tracking: Track key performance indicators (KPIs) related to model accuracy and explainability.
- Feedback Loop: Establish a feedback loop with users and stakeholders to continuously improve the system.
- Regulatory Updates: Stay updated on regulatory changes and adjust your XAI implementations accordingly.
7. training and Skills Development
Ensure that your team has the necessary skills to work with XAI Tools:
- training Programs: Offer training programs or workshops focused on explainable AI Techniques and Tools.
- Expertise Hiring: Consider hiring experts in AI explainability if in-house expertise is lacking.
Use Cases of Explainable AI
To better understand the practical applications of XAI, let's explore some real-world use cases:
1. healthcare
In healthcare, explainable AI can help doctors make more informed decisions by providing clear explanations for diagnostic suggestions. For example, an explainable AI system might highlight specific features in a medical image that indicate a particular condition, helping radiologists confirm or refute the diagnosis.
Example: An XAI tool used in pathology can identify and explain which cells or structures in a biopsy sample are indicative of cancer, enabling pathologists to validate the findings.
2. Finance
In finance, explainable AI can enhance transparency in credit scoring, Fraud Detection, and risk management. For instance, an explainable credit scoring model can provide clear reasons why a loan application was approved or denied, helping borrowers understand the decision-making process.
Example: A bank using XAI for Fraud Detection can generate explanations for flagged transactions, enabling investigators to quickly verify or dismiss potential fraud cases.
3. customer service
In customer service, explainable AI can improve chatbots and virtual assistants by making their decisions more transparent. For example, an explainable recommendation system in an e-commerce platform can provide customers with clear reasons for product suggestions, Enhancing trust and satisfaction.
Example: A retail company using XAI in its recommendation engine can explain why a particular item was suggested to a customer, based on their browsing history and purchase patterns.
4. Legal Services
In legal services, explainable AI can assist in document review and case prediction by providing clear explanations for its decisions. For instance, an explainable AI system might highlight specific clauses in a contract that indicate potential risks or issues, helping lawyers make more informed decisions.
Example: A law firm using XAI for case prediction can generate explanations for predicted outcomes, enabling attorneys to better prepare their cases and advise clients.
Future of Explainable AI
As the demand for transparency and accountability continues to grow, explainable AI is poised to become a standard practice in many industries. Here are some future trends to watch:
1. Increased Adoption
More businesses will adopt XAI as regulatory pressures and customer expectations for transparency increase. This trend will be driven by the need to build trust, ensure compliance, and drive Innovation.
2. Advancements in Explainability Techniques
Researchers and developers will continue to innovate, creating more sophisticated and user-friendly explainability Techniques. These advancements will make IT easier for businesses to implement XAI and benefit from its transparency.
3. integration with Other technologies
XAI will increasingly be integrated with other emerging technologies like Blockchain and the Internet of Things (IoT) to create even more transparent and secure systems. For example, explainable AI combined with Blockchain can provide an immutable record of decisions and their explanations.
4. Ethical AI
The focus on ethical AI will grow, with businesses prioritizing fairness, accountability, and transparency in their AI implementations. Explainable AI will play a crucial role in achieving these Ethical Standards by making AI systems more understandable and trustworthy.
Explainable AI (XAI) is not just a technological trend but a necessity for modern businesses seeking to navigate the complexities of AI ethics, regulatory compliance, and customer trust. By understanding what XAI is and why IT matters, companies can leverage this technology to enhance transparency, ensure compliance, improve decision-making, and build customer trust.
Implementing XAI requires a structured approach that includes assessing business needs, choosing the right Tools, preparing high-quality data, training models with explainability in mind, evaluating and validating results, continuously monitoring performance, and developing the necessary skills within your team. Real-world use cases in healthcare, finance, customer service, and legal services demonstrate the practical benefits of XAI across industries.
As we look to the future, the adoption of XAI will continue to grow, driven by advancements in explainability Techniques, integration with other technologies, and a greater emphasis on ethical AI. Businesses that embrace XAI early will be better positioned to navigate regulatory challenges, build customer trust, and drive Innovation.
In summary, explainable AI is a critical component of responsible and effective AI implementation. By prioritizing transparency, fairness, and accountability, businesses can harness the power of AI while building trust with customers, stakeholders, and regulators.