Ethical AI Engineering Practices: Ensuring Fairness, Transparency, and Accountability
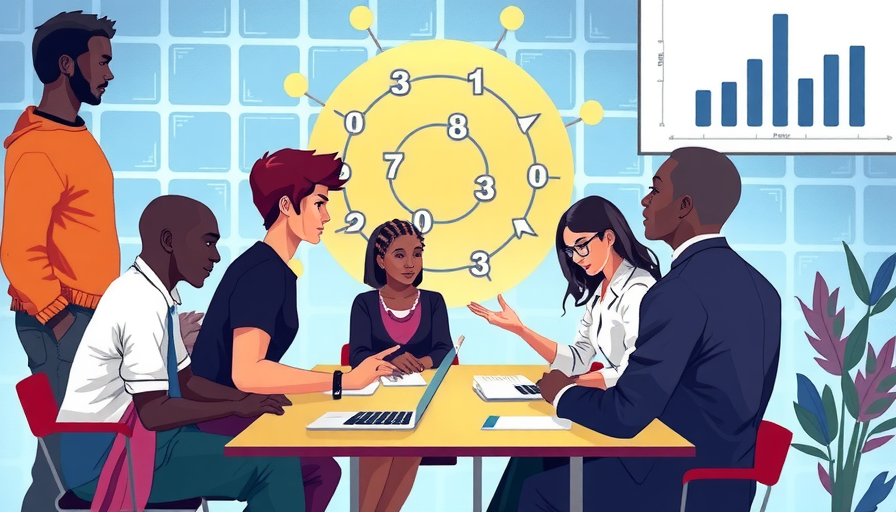
Artificial Intelligence (AI) is transforming industries and societies at an unprecedented pace. From healthcare and finance to Transportation and entertainment, AI systems are being integrated into every aspect of our lives. However, with the increasing adoption of AI comes a growing need for ethical considerations to ensure that these technologies are developed and deployed responsibly. Ethical AI Engineering practices focus on creating fair, transparent, and accountable AI systems that benefit society without causing harm.
The Importance of Ethical AI Engineering
Ethical AI Engineering is crucial for several reasons:
-
Preventing Harm: AI systems can inadvertently cause harm if not designed with ethical considerations in mind. For example, biased algorithms can perpetuate or amplify existing inequalities. Ethical AI Engineering helps prevent such outcomes by promoting fairness and inclusivity.
-
Building Trust: Transparent and accountable AI systems build trust with users and stakeholders. When people understand how AI decisions are made and who is responsible for them, they are more likely to accept and adopt these technologies.
-
compliance with Regulations: Many regions have implemented Regulations governing AI and data use, such as the General data Protection regulation (GDPR) in Europe and the California Consumer privacy Act (CCPA) in the United States. Ethical AI Engineering helps organizations comply with these Regulations and avoid legal penalties.
-
Promoting Innovation: By focusing on ethical considerations, AI developers can create more innovative and impactful solutions that address real-world problems. Ethical AI Engineering encourages a holistic approach to problem-solving that considers societal impacts alongside technological advancements.
Key Principles of Ethical AI Engineering
To ensure the responsible development and deployment of AI systems, several key principles must be followed:
-
- Definition: fairness in AI means creating systems that treat all users equally and do not perpetuate or amplify existing biases.
- Importance: Biased AI algorithms can lead to discriminatory outcomes, such as unfair lending practices or biased hiring decisions.
- Examples:
- Biased facial recognition: Studies have shown that facial recognition systems are less accurate for people of color and women. To address this, developers can use diverse datasets that include a wide range of ethnicities and genders during training.
- algorithmic bias in Lending: AI algorithms used by banks to assess creditworthiness may inadvertently discriminate against certain demographic groups. Regular bias audits can help identify and mitigate these issues.
-
- Definition: transparency involves making AI processes understandable to those who are affected by them.
- Importance: Transparent AI systems build trust with users and stakeholders by providing clarity on how decisions are made.
- Examples:
- Explainable AI (XAI): Techniques like LIME (Local Interpretable Model-Agnostic Explanations) and SHAP (SHapley Additive exPlanations) can help explain the decisions made by complex AI models.
- Documentation: Clearly documenting the decision-making process of AI models, including the data sources used and the algorithms employed, ensures that stakeholders can audit and verify the system's behavior.
-
- Definition: accountability in AI Engineering means holding developers and organizations responsible for the outcomes of their AI systems.
- Importance: Accountable AI systems ensure that issues are addressed promptly and that users have recourse if they are harmed by AI decisions.
- Examples:
- AI Incident Reporting: Organizations can establish procedures for reporting and addressing AI incidents, such as biased or inaccurate predictions.
- Ethical Review Boards: Companies can create ethical review boards to oversee AI development and ensure compliance with Ethical Standards.
-
- Definition: privacy in AI involves protecting user data and ensuring that AI systems comply with relevant Regulations.
- Importance: Protecting user data is essential for maintaining trust and complying with legal requirements.
- Examples:
- data Anonymization: Techniques like differential privacy can help anonymize data used by AI systems, reducing the risk of privacy breaches.
- User Consent: Obtaining explicit user consent before collecting and using personal data ensures that users are informed and in control of their information.
-
- Definition: security in AI Engineering involves protecting AI systems from malicious attacks and ensuring the integrity of the data they process.
- Importance: Secure AI systems prevent unauthorized access, data breaches, and other cyber threats.
- Examples:
- encryption: Encrypting sensitive data both at rest and in transit protects IT from unauthorized access.
- Access Controls: Implementing strict access controls ensures that only authorized personnel can interact with AI systems.
-
Human Oversight
- Definition: Human oversight involves ensuring that AI systems operate within acceptable parameters and that critical decisions are reviewed by humans.
- Importance: Human-in-the-loop approaches help mitigate risks associated with autonomous decision-making by AI.
- Examples:
- Medical Diagnosis: In healthcare, AI systems can assist in diagnosing diseases, but a human doctor should review the AI's recommendations before making a final diagnosis.
- autonomous vehicles: self-driving cars should have a human driver ready to take control in case of unexpected situations.
-
Inclusivity
- Definition: Inclusivity in AI Engineering means designing systems that consider the needs and perspectives of diverse user groups.
- Importance: inclusive AI systems are more likely to be accepted and beneficial to all users, regardless of their background.
- Examples:
- Accessible design: Ensuring that AI interfaces are accessible to people with disabilities, such as providing text-to-speech functionality for visually impaired users.
- Diverse Stakeholder Involvement: Involving stakeholders from different backgrounds in the development process can help identify and address potential biases or accessibility issues.
-
- Definition: continuous improvement involves regularly reviewing and updating AI systems to address new challenges and emerging ethical issues.
- Importance: Ethical AI practices are not a one-time effort but an ongoing commitment that ensures AI systems remain fair, transparent, and accountable over time.
- Examples:
best practices for Ethical AI Engineering
Implementing ethical AI engineering practices requires a comprehensive approach that incorporates various best practices:
-
Conduct Regular Bias Audits
- Why IT Matters: Bias audits help identify and mitigate biases in AI models, ensuring fairness and inclusivity.
- How to Do IT:
- Diverse Datasets: Use diverse datasets that represent a wide range of user groups during the training and testing phases.
- Bias Detection Tools: Utilize Tools like IBM's AI fairness 360 or Microsoft's Fairlearn to detect biases in your models.
- Stakeholder Involvement: Involve stakeholders from different backgrounds in the audit process to ensure that potential biases are identified and addressed.
-
Document decision-making Processes
- Why IT Matters: Transparent documentation builds trust with users and stakeholders by providing clarity on how AI decisions are made.
- How to Do IT:
- Detailed Documentation: Clearly document the data sources, algorithms, and decision-making processes used in your AI models.
- Explainable AI (XAI): Implement XAI Techniques like LIME or SHAP to explain the decisions made by complex AI models.
- Regular Updates: Keep documentation up-to-date as AI systems evolve and new features are added.
-
Implement Robust security Measures
- Why IT Matters: Secure AI systems prevent unauthorized access, data breaches, and other cyber threats.
- How to Do IT:
- encryption: Encrypt sensitive data both at rest and in transit to protect IT from unauthorized access.
- Access Controls: Implement strict access controls to ensure that only authorized personnel can interact with AI systems.
- Regular security Audits: Conduct regular security audits to identify and address potential vulnerabilities.
-
Ensure compliance with privacy Regulations
- Why IT Matters: Complying with privacy Regulations like GDPR and CCPA helps protect user data and avoid legal penalties.
- How to Do IT:
- data Anonymization: Use Techniques like differential privacy to anonymize data used by AI systems.
- User Consent: Obtain explicit user consent before collecting and using personal data, ensuring that users are informed and in control of their information.
- privacy Impact Assessments: Conduct regular privacy impact assessments to identify and mitigate potential risks to user data.
-
Promote Human Oversight
- Why IT Matters: Human oversight helps mitigate risks associated with autonomous decision-making by AI, ensuring that critical decisions are reviewed by humans.
- How to Do IT:
- Human-in-the-Loop Approaches: Incorporate human-in-the-loop approaches in your AI systems, such as requiring human approval before implementing certain actions.
- training and Education: Provide training and education for human overseers to ensure they understand the AI system's capabilities and limitations.
- Clear Guidelines: Establish clear guidelines for when human oversight is required and how IT should be implemented.
-
Engage Stakeholders Early On
- Why IT Matters: Engaging stakeholders from diverse backgrounds early in the development process helps ensure that AI systems meet the needs of all users and avoid discriminatory practices.
- How to Do IT:
- Diverse Teams: Assemble development teams with members from different backgrounds, including underrepresented groups.
- User Feedback: Collect and incorporate user feedback throughout the development process to identify and address potential issues.
- Ethical Review Boards: Create ethical review boards to oversee AI development and ensure compliance with Ethical Standards.
-
Continuously Monitor and Update Systems
- Why IT Matters: continuous monitoring and updating help maintain fairness, transparency, and accountability over time, addressing new challenges and emerging ethical issues.
- How to Do IT:
- Regular Audits: Conduct regular audits of AI models to identify and mitigate biases or other ethical issues.
- User Feedback: Collect and act on user feedback to improve AI systems and address any concerns or issues that arise.
- Version Control: Implement version control for your AI models, documenting changes and updates over time.
case studies in Ethical AI Engineering
-
healthcare: Bias in Medical Algorithms
- Issue: A medical algorithm used to predict patient outcomes was found to be less accurate for Black patients than for white patients.
- Solution: The developers conducted a bias audit, identified the issue, and retrained the model using a more diverse dataset that included a broader range of ethnicities. They also implemented regular audits to monitor for biases in future updates.
-
Finance: algorithmic bias in Lending
- Issue: An AI algorithm used by a bank to assess creditworthiness was found to disproportionately reject loan applications from women and minorities.
- Solution: The bank established an ethical review board to oversee AI development, conducted regular bias audits, and implemented fairness-aware machine learning Techniques to mitigate biases in the lending process.
-
Transportation: Ethical Considerations in autonomous vehicles
- Issue: Developers of self-driving cars needed to address ethical dilemmas, such as how the vehicle should behave in situations where an accident is unavoidable.
- Solution: The company involved ethicists and stakeholders from diverse backgrounds in the development process, conducted extensive Simulations and real-world tests, and established clear guidelines for human oversight and intervention.
Ethical AI Engineering is essential for creating responsible and trustworthy AI systems. By adhering to key principles such as fairness, transparency, accountability, privacy, security, human oversight, inclusivity, and continuous improvement, developers can ensure that their AI solutions benefit society without causing harm. Embracing these best practices and learning from real-world case studies can help build ethical AI systems that users can trust.
As AI continues to evolve, IT is crucial for developers, organizations, and policymakers to work together to promote ethical AI engineering practices. By doing so, we can harness the power of AI to create a more just, equitable, and sustainable future for all.