Ethical AI Engineering Practices: Building Trust and Responsibility
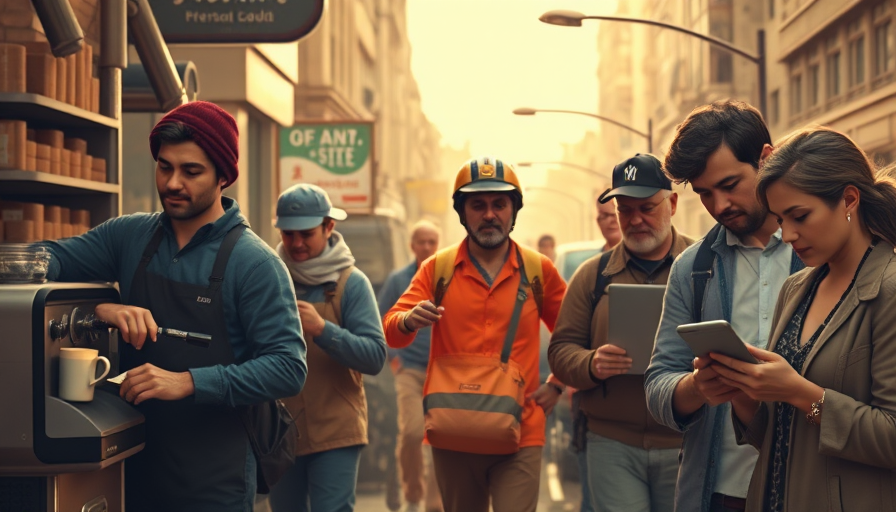
In today's rapidly evolving technological landscape, ethical considerations in artificial intelligence (AI) Engineering have become more crucial than ever. As AI continues to permeate various aspects of our lives, from healthcare to finance, ensuring that these systems are developed responsibly is paramount. This comprehensive guide delves into the importance of ethical AI Engineering practices, providing detailed explanations, real-world examples, and actionable insights for developers, engineers, and organizations.
What Are Ethical AI Engineering Practices?
Ethical AI Engineering practices refer to a set of guidelines and principles designed to ensure that AI systems are fair, transparent, accountable, and beneficial to society. These practices aim to mitigate biases, protect user privacy, and promote the responsible use of AI technologies. By integrating ethical considerations into every stage of the AI development lifecycle, organizations can build trust with users and stakeholders.
Key Principles of Ethical AI
-
fairness: Ensuring that AI algorithms do not discriminate against any group based on race, gender, age, or other protected characteristics.
- Example: A lending algorithm should not deny loans to applicants based on their race or ethnicity. To achieve fairness, developers can use Techniques like re-sampling, re-weighing, or applying prejudice remover algorithms to mitigate biases in the training data.
-
transparency: Making sure that AI decision-making processes are understandable and explainable to stakeholders.
- Example: In healthcare, an AI system used for diagnosing diseases should provide clear explanations for its recommendations. This can be achieved through Techniques like LIME (Local Interpretable Model-agnostic Explanations) or SHAP (SHapley Additive exPlanations), which help interpret the predictions of complex models.
-
accountability: Establishing clear responsibilities for the development and deployment of AI systems.
- Example: Organizations should have designated individuals or teams responsible for overseeing AI projects, ensuring that ethical considerations are addressed throughout the development process. This includes conducting regular audits and impact assessments.
-
privacy: Protecting user data and ensuring that AI does not infringe on individuals' privacy rights.
- Example: An AI-powered recommendation system should anonymize user data and obtain explicit consent before collecting and using personal information. differential privacy Techniques can be employed to add noise to the data, preserving privacy while maintaining utility.
-
Robustness and security: Ensuring that AI systems are secure and can withstand adversarial attacks.
- Example: A self-driving car's AI should be robust against adversarial examples, such as small perturbations in the input data designed to fool the model. Techniques like adversarial training can help improve the system's resilience.
-
Beneficence and Non-Maleficence: Ensuring that AI systems promote well-being and do not cause harm.
- Example: An AI chatbot providing mental health support should be designed with the user's best interests in mind, avoiding suggestions that could exacerbate their condition. Regular evaluations by mental health professionals can help ensure the system's beneficence.
-
autonomy: Respecting and preserving human autonomy when interacting with AI systems.
- Example: An AI-driven decision support system should not override human judgments but rather provide recommendations that users can accept or reject. clear communication of the system's limitations is essential to preserve user autonomy.
The Importance of Ethical AI Engineering
As AI becomes more integrated into our daily lives, IT is essential to prioritize ethical considerations. Unethical practices can lead to severe consequences, including financial loss, reputational damage, legal repercussions, and harm to individuals or communities. By adopting ethical AI Engineering practices, organizations can build trust with their users and stakeholders, fostering a more responsible and sustainable AI ecosystem.
Real-World Examples of Ethical AI Failures
Several high-profile incidents highlight the risks associated with unethical AI Engineering:
-
Biased Algorithms: facial recognition systems developed by companies like Amazon and IBM have been shown to disproportionately misidentify people of color, leading to potential wrongful arrests and reinforcing racial biases in law enforcement.
- Mitigation: To address this issue, developers can use diverse and representative datasets for training facial recognition models and regularly audit the system's performance across different demographic groups.
-
privacy Breaches: AI-driven data collection practices by companies like Cambridge Analytica compromised the privacy of millions of users, enabling political manipulation and eroding public trust in technology.
- Mitigation: Organizations should implement differential privacy Techniques, obtain explicit user consent, and adhere to data minimization principles to protect user privacy.
-
Autonomous Weapons: The development of AI-controlled weapons without sufficient ethical oversight raises serious concerns about the potential for autonomous systems to cause harm or be used in unauthorized ways.
- Mitigation: International cooperation is essential to establish clear guidelines and Regulations for the responsible development and deployment of autonomous weapons, ensuring human oversight and accountability.
-
job displacement: The Automation of jobs through AI can lead to significant job displacement, exacerbating economic inequalities and social unrest.
- Mitigation: Policymakers should invest in reskilling and upskilling programs, promoting lifelong learning, and fostering a just transition for workers affected by Automation.
These examples underscore the need for robust ethical guidelines in AI Engineering.
Implementing Ethical AI Engineering Practices
To incorporate ethical considerations into your AI projects, follow these best practices:
-
Conduct Regular Audits: Perform regular audits to identify and mitigate biases in AI algorithms.
- Example: A financial institution can conduct periodic bias audits of its credit scoring algorithm to ensure IT does not disproportionately disadvantage certain demographic groups.
-
Diverse Teams: Foster diverse teams to bring different perspectives and reduce the risk of biased outcomes.
- Example: A tech company developing a language processing AI can include team members from various linguistic backgrounds to ensure the system understands and generates text in multiple languages accurately.
-
transparency Reports: Publish transparency reports detailing how your AI systems work and how decisions are made.
- Example: A social media platform can release a transparency report explaining its content moderation algorithms, including the data sources used for training and the criteria for flagging or removing content.
-
User Consent: Obtain explicit user consent for data collection and usage.
- Example: A healthcare AI application can provide users with clear and concise explanations of how their data will be collected, stored, and used, obtaining their informed consent before proceeding.
-
accountability Mechanisms: Establish clear accountability mechanisms for AI development and deployment.
- Example: A self-driving car manufacturer can implement a chain of responsibility, ensuring that specific individuals are accountable for the safety and ethical considerations of the vehicle's AI system.
-
ethical guidelines and Frameworks: Adopt established ethical guidelines and frameworks, such as those proposed by the European Commission or the IEEE Global Initiative on Ethics of Autonomous and Intelligent Systems.
-
Stakeholder Engagement: Engage with stakeholders, including users, communities, and experts, to gather input and address concerns related to AI ethics.
-
Ethical training: Provide ethical training for developers, engineers, and other stakeholders involved in AI projects.
-
Impact Assessments: Conduct AI impact assessments to evaluate the potential consequences of AI systems on individuals, communities, and society at large.
-
continuous monitoring: Implement continuous monitoring mechanisms to track the performance and ethical implications of AI systems post-deployment.
Ethical AI Engineering in Specific Industries
healthcare
In healthcare, ethical AI Engineering practices are crucial for ensuring patient safety, data privacy, and equitable access to care. Some key considerations include:
-
Bias Mitigation: Addressing biases in medical AI algorithms that could lead to disparities in diagnosis or treatment.
- Example: A diagnostic AI system should be trained on diverse datasets representing various demographic groups to avoid reinforcing existing health inequalities.
-
transparency and Explainability: Ensuring that AI-driven clinical decisions are transparent and explainable to healthcare professionals and patients.
- Example: An AI system recommending a treatment plan should provide clear explanations for its suggestions, allowing clinicians to understand the underlying rationale.
-
privacy Protection: Safeguarding patient data and ensuring compliance with Regulations like HIPAA or GDPR.
- Example: A Telemedicine platform can use end-to-end encryption and differential privacy Techniques to protect patient data during consultations.
Finance
In finance, ethical AI Engineering practices help promote fair lending, fraud detection, and Risk Management while mitigating biases and ensuring transparency. Some key considerations include:
-
fairness in Lending: Ensuring that AI-driven credit scoring models do not discriminate against applicants based on protected characteristics.
- Example: A lending algorithm should be regularly audited for bias, with mitigation Techniques applied as needed to ensure fair treatment of all applicants.
-
transparency in decision-making: Providing clear explanations for AI-driven financial decisions, such as loan approvals or credit limit adjustments.
- Example: A bank can use explainable AI Techniques like LIME or SHAP to help customers understand the factors influencing their credit score.
-
Robustness and security: Protecting financial AI systems from adversarial attacks and ensuring their resilience against data manipulations.
Criminal Justice
In the criminal justice system, ethical AI Engineering practices are essential for promoting fairness, accountability, and public trust. Some key considerations include:
-
Bias Mitigation: Addressing biases in Predictive Policing, risk assessment, and sentencing algorithms that could lead to unfair treatment of individuals or communities.
- Example: A risk assessment tool used in pretrial decisions should be regularly audited for bias, with mitigation Techniques applied to ensure fair outcomes.
-
transparency and accountability: Ensuring that AI-driven criminal justice decisions are transparent and accountable, with clear responsibilities established for their development and deployment.
- Example: Law enforcement agencies can publish transparency reports detailing the algorithms used in Predictive Policing, including their data sources and decision-making processes.
-
Human Oversight: Preserving human autonomy and judgment in critical decisions, such as sentencing or parole determinations.
- Example: An AI-driven sentencing recommendation system should provide recommendations rather than final decisions, with ultimate authority resting with judicial officials.
Employment
In the employment sector, ethical AI Engineering practices help promote fair hiring, employee monitoring, and workplace decision-making while mitigating biases and ensuring transparency. Some key considerations include:
-
fairness in Hiring: Ensuring that AI-driven recruitment algorithms do not discriminate against job applicants based on protected characteristics.
- Example: A resume screening algorithm should be regularly audited for bias, with mitigation Techniques applied to ensure fair treatment of all applicants.
-
transparency in decision-making: Providing clear explanations for AI-driven employment decisions, such as hiring or promotion recommendations.
- Example: An HR software can use explainable AI Techniques like LIME or SHAP to help managers understand the factors influencing a candidate's suitability for a role.
-
privacy Protection: Safeguarding employee data and ensuring compliance with relevant privacy Regulations.
- Example: A company using an AI-driven performance monitoring system can implement differential privacy Techniques to protect employee data while maintaining the system's utility.
Ethical AI Engineering practices are not just a buzzword; they are essential for building trust, ensuring fairness, and promoting the responsible use of technology. By adopting these principles, organizations can create AI systems that benefit society while mitigating potential risks. Whether you are an AI engineer, developer, or policymaker, prioritizing ethical considerations is crucial for the sustainable development of AI.
The Path Forward
To foster a more ethical and responsible AI ecosystem, stakeholders must collaborate and take proactive steps:
- Policy and regulation: Governments should develop clear guidelines and Regulations for ethical AI Engineering, promoting transparency, accountability, and fairness.
- Education and Awareness: Educational institutions should incorporate AI ethics into their curricula, fostering a new generation of responsible AI practitioners.
- research and Development: Invest in research focused on developing ethical AI technologies, such as bias mitigation Techniques, explainable AI, and differential privacy.
- Public Engagement: Encourage public discussions and debates about AI ethics, involving diverse stakeholders in shaping the future of technology.
For more information on ethical AI Engineering practices, stay tuned to our blog and follow us on social media. Together, we can build a more responsible and trustworthy AI-driven world.