Engineering Robust AI Systems
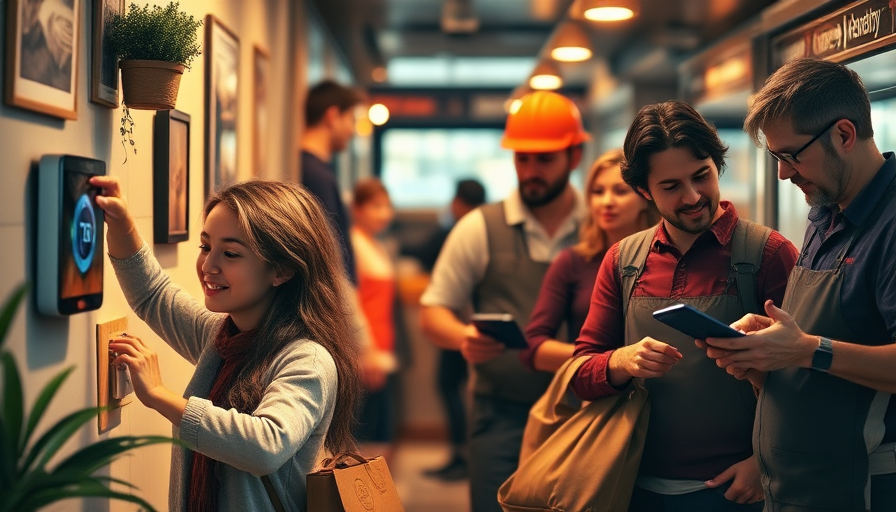
artificial intelligence (AI) has emerged as a transformative force across numerous industries, offering automated solutions that enhance efficiency, accuracy, and decision-making processes. However, developing robust AI systems is a multifaceted challenge that demands careful planning, rigorous execution, and ongoing maintenance. This comprehensive guide will delve into the best practices and strategies for Engineering robust AI systems to ensure they perform reliably under a wide range of conditions.
Understanding Robust AI Systems
A robust AI system is engineered to maintain its performance and integrity even when confronted with unexpected inputs or variations in data. These systems are particularly critical in applications where failures can have severe consequences, such as healthcare diagnostics, autonomous vehicles, financial trading platforms, and cybersecurity. To achieve robustness, developers must consider several fundamental factors:
- data Quality: The bedrock of any AI system is the quality of its input data. High-quality, diverse datasets ensure that the model can generalize well across different scenarios and handle real-world variability.
- Model Validation: Continuous validation and testing are essential to identify weaknesses, improve performance, and ensure the model's reliability.
- adaptability: The ability to adapt to new data or changing conditions without significant retraining is a key characteristic of robust AI systems.
Importance of Robustness in AI
Robustness in AI is crucial for several reasons:
- reliability: Ensures that the system performs consistently under various conditions.
- safety: Critical in applications where failures can lead to harm, such as medical diagnoses or autonomous driving.
- Trust: Builds user confidence by demonstrating consistent and accurate performance.
- efficiency: Reduces the need for frequent retraining and updates, saving time and resources.
best practices for Engineering Robust AI Systems
1. Start with Quality data
The first step in building a robust AI system is ensuring that your data is clean, accurate, and representative of the real-world scenarios the system will encounter. data quality is paramount as IT directly influences the model’s performance.
data Collection
- Diverse Sources: Collect data from multiple sources to capture a wide range of scenarios.
- Relevance: Ensure the data is relevant to the problem you are trying to solve.
data Cleaning
- Handling Missing Values: Use Techniques like imputation or deletion to handle missing data.
- Removing Duplicates: Eliminate duplicate records to avoid bias and inconsistency.
- Outlier Detection: Identify and handle outliers that can skew model performance.
Example: healthcare data
In a healthcare setting, collecting diverse medical records from different hospitals and clinics ensures that the AI model can generalize well across various patient populations. Cleaning this data involves handling missing values in patient histories, removing duplicate entries, and identifying outliers in test results.
2. Employ Cross-Validation Techniques
Cross-validation helps to assess how well a model generalizes to an independent dataset. Techniques such as k-fold cross-validation can provide more reliable estimates of model performance and help identify overfitting issues.
K-Fold Cross-Validation
- Process: Divide the data into k subsets (folds). Train the model on k-1 folds and validate IT on the remaining fold. Repeat this process k times, each time using a different fold for validation.
- Benefits: Provides a more comprehensive evaluation of model performance by using different subsets of data for training and validation.
Example: fraud detection
In fraud detection, k-fold cross-validation can be used to ensure that the model generalizes well across different types of transactions. By training on various subsets of transaction data and validating on unseen data, the model can detect fraudulent activities more accurately.
3. Incorporate Adversarial Testing
Adversarial testing involves creating adversarial examples that challenge the AI system's assumptions and boundaries. This helps in identifying vulnerabilities and ensuring the system can handle edge cases effectively.
Types of Adversarial Attacks
- Fast Gradient Sign Method (FGSM): Adds small perturbations to input data to fool the model.
- Projected Gradient Descent (PGD): An iterative version of FGSM that applies multiple small perturbations.
- Black-Box Attacks: Use queries to the model to generate adversarial examples without knowing the internal workings.
Example: autonomous vehicles
In autonomous vehicles, adversarial testing can involve adding subtle changes to road signs or traffic lights to see if the AI system misinterprets them. This helps in identifying vulnerabilities and improving the robustness of the self-driving system.
4. Regular Monitoring and Updating
AI systems should be continuously monitored for performance degradation or anomalies. Regular updates and retraining with new data ensure the model remains relevant and accurate over time.
Monitoring Techniques
- Performance Metrics: Track key performance indicators (KPIs) like accuracy, Precision, recall, and F1 score.
- anomaly detection: Use statistical methods or Machine Learning models to detect unusual patterns or outliers in the system's behavior.
- Logging and Alerts: Implement logging mechanisms to record system behavior and set up alerts for critical events.
Example: Financial Trading
In financial trading, continuous monitoring of AI models can help detect performance degradation due to changing market conditions. Regular updates and retraining with new market data ensure that the model remains accurate and effective.
5. Ensure scalability
As AI systems grow, they need to scale efficiently without compromising on performance. Designing scalable architectures that can handle increased loads is crucial for long-term robustness.
scalability Strategies
- Distributed computing: Use distributed computing frameworks like Apache Spark or Hadoop to process large datasets.
- Cloud Services: Leverage cloud platforms like AWS, Azure, or Google Cloud for scalable computing resources.
- microservices architecture: Break down the system into smaller, independent services that can scale individually.
Example: e-commerce Recommendation Systems
In e-commerce, recommendation systems need to handle a large number of users and products. Using distributed computing frameworks and cloud services ensures that the system can scale efficiently during peak shopping seasons without degrading performance.
Strategies for Ensuring Robustness
1. Use Ensemble Methods
Ensemble methods combine multiple models to improve overall prediction accuracy and robustness. Techniques like bagging, boosting, and stacking can enhance the reliability of AI systems.
Bagging
- Process: Train multiple models on different subsets of the data and combine their predictions through voting or averaging.
- Example: Random Forest is a popular bagging technique that combines decision trees to improve predictive performance.
Boosting
- Process: Train models sequentially, with each new model focusing on the errors made by previous models.
- Example: Gradient Boosting Machines (GBM) and XGBoost are boosting algorithms that build strong predictors by combining weak learners.
Stacking
- Process: Combine multiple models by training a meta-model on their predictions.
- Example: Stacking can be used to combine the outputs of neural networks, decision trees, and support vector machines to improve overall accuracy.
2. Implement Robust training Procedures
Robust training procedures include regularization Techniques that prevent overfitting and ensure the model generalizes well across different datasets.
Regularization Techniques
- L1 Regularization (Lasso): Adds a penalty equal to the absolute value of the magnitude of coefficients.
- L2 Regularization (Ridge): Adds a penalty equal to the square of the magnitude of coefficients.
- Elastic Net: Combines L1 and L2 regularization.
Example: Image Classification
In image classification, regularization Techniques can help prevent overfitting by penalizing complex models that fit noise in the training data. This ensures that the model generalizes well to new, unseen images.
3. Focus on Explainability and transparency
Explainable AI (XAI) ensures that the decision-making process of AI models is transparent and understandable. This helps in building trust and identifying potential biases or errors in the system.
XAI Techniques
- LIME (Local Interpretable Model-Agnostic Explanations): Explains individual predictions by approximating the model locally with an interpretable model.
- SHAP (SHapley Additive exPlanations): Provides a unified measure of feature importance based on game theory.
- Counterfactual Explanations: Explain model decisions by showing what changes in input would lead to different outcomes.
Example: Credit Scoring
In credit scoring, XAI Techniques can help explain why an applicant was denied a loan. This transparency builds trust with customers and ensures that the decision-making process is fair and unbiased.
4. Adopt Secure Development Practices
security is a critical aspect of robustness. Implementing Secure Coding practices, regular security audits, and encryption can protect AI systems from malicious attacks and data breaches.
Secure Coding Practices
- Input Validation: Validate all inputs to prevent injection attacks.
- Authentication and Authorization: Ensure that only authorized users can access the system.
- encryption: Use strong encryption algorithms to protect sensitive data.
Example: healthcare AI Systems
In healthcare, secure development practices are crucial to protect patient data from unauthorized access. Implementing input validation, authentication mechanisms, and encryption ensures that patient information remains confidential and secure.
case studies: Examples of Robust AI Systems
Several industries have successfully implemented robust AI systems to improve their operations:
healthcare
AI models are used for diagnosing diseases with high accuracy, ensuring patient safety. For example, AI-powered diagnostic Tools can analyze medical images to detect conditions like cancer or heart disease more accurately than human radiologists.
Example: Cancer Detection
An AI system developed by Google Health uses deep learning algorithms to analyze mammograms and detect breast cancer with high accuracy. The system was trained on a diverse dataset of mammograms from different hospitals, ensuring robustness across various patient populations.
autonomous vehicles
self-driving cars rely on robust AI systems to navigate complex environments safely. These systems use sensor data from cameras, LIDAR, and radar to make real-time decisions about steering, acceleration, and braking.
Example: Waymo
Waymo's autonomous vehicles use a combination of deep learning and classical Computer Vision Techniques to perceive their Environment and make safe driving decisions. The system is continuously validated through extensive testing on public roads and simulation environments.
Finance
fraud detection algorithms help financial institutions identify and prevent fraudulent activities effectively. These systems analyze transaction patterns to detect anomalies that indicate potential fraud.
Example: PayPal
PayPal uses Machine Learning models to detect and prevent fraudulent transactions in real-time. The system is continuously updated with new data to adapt to evolving fraud Techniques, ensuring robustness and accuracy.
cybersecurity
AI-driven cybersecurity systems can detect and mitigate threats more effectively than traditional methods. These systems use behavioral analysis and anomaly detection to identify suspicious activities.
Example: Darktrace
Darktrace's AI-powered cybersecurity platform uses unsupervised Machine Learning algorithms to detect and respond to cyber threats in real-time. The system adapts to the unique patterns of behavior within an organization, ensuring robustness against a wide range of attack vectors.
Conclusion
Engineering robust AI systems requires a combination of best practices, rigorous testing, and continuous monitoring. By focusing on data quality, employing cross-validation techniques, incorporating adversarial testing, and ensuring scalability, developers can create AI models that perform reliably under various conditions. Regular updates, secure development practices, and explainable AI further enhance the robustness and trustworthiness of these systems.
Incorporate these strategies into your AI development process to build resilient systems capable of handling real-world challenges effectively. As AI continues to evolve, prioritizing robustness will be key to ensuring that these technologies deliver consistent, reliable, and safe outcomes across diverse applications.