Deep Learning Applications in Image and Voice Recognition: The Current State of the Art
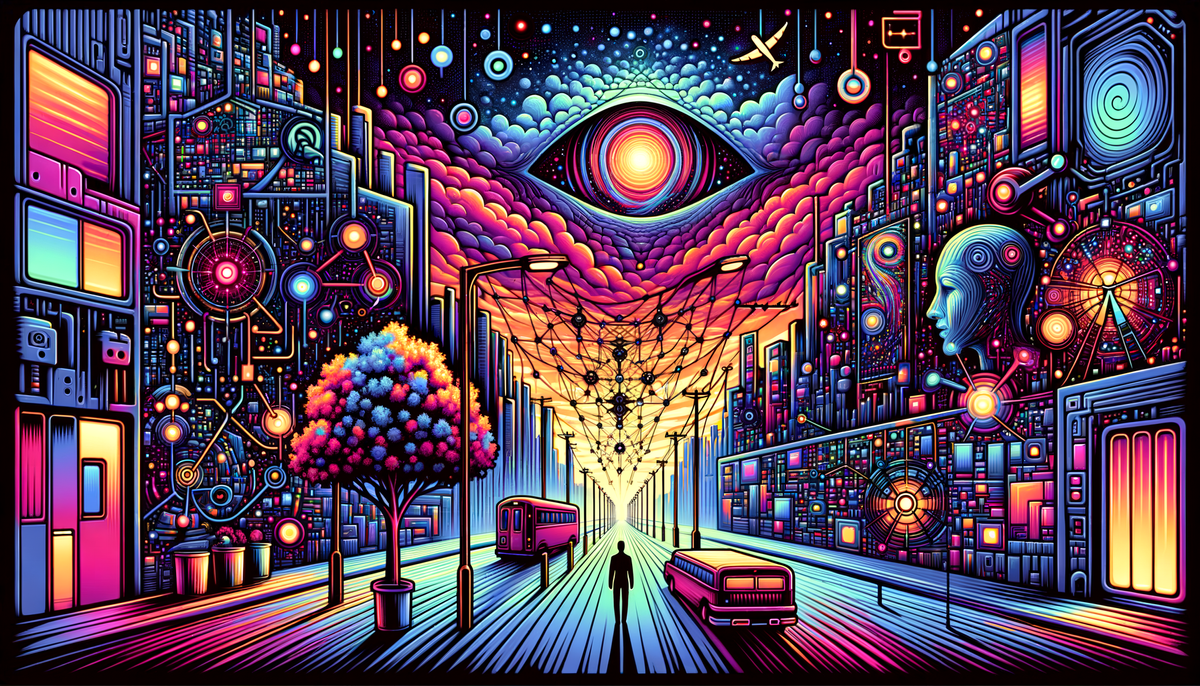
Today, we are witnessing a massive shift and undeniable surge in the area of Artificial Intelligence (AI) applications. AI is largely driven by Deep Learning methods, with its intriguing applications in various domains, like image and voice recognition, proving to be revolutionary in many ways.
Deep Learning, a subsector of machine learning, employs complex algorithms to mull over high-volume, diverse data to reach actionable insights. In the past decade, Deep Learning has significantly catalyzed advancements in the domains of image and voice recognition technologies. IT has resulted in increasing accuracy levels, thereby creating an impactful footprint in these areas.
Current State of the Art in Image Recognition
Image recognition is widely leveraged technology in an array of applications, including autonomous cars, facial recognition, Medical Imaging, and surveillance systems. The objective that drives the current image recognition technology is not just accurate object identification but also understanding the contextual interactions within the image.
The latest developments in image recognition heavily rely on convolutional Neural Networks (CNNs), a specialized design of the Deep Learning framework pertinent for processing grid-like data, such as a picture. CNNs possess the ability to automatically and adaptively learn image features from large training datasets, ensuring high image recognition accuracy. Today, CNN-accelerated image recognition is remarkably deployed in Facebook's automatic photo tagging and Google's image search.
Current State of the Art in Voice Recognition
Voice recognition is instrumental in diverse applications like Speech-to-Text processing, voice biometric access, Voice Assistants, and more. Deep Learning is pushing the boundaries in voice recognition technology by improving accuracy and user experience.
Currently, voice recognition hinges on recurrent Neural Networks (RNNs) and Transformers. RNNs have created a niche for themselves in processing sequential data like speech. They have the capacity to learn patterns in time series data, drawing meaningful insights. The transformer model that undergirds Google's voice assistant improves speech recognition by understanding the contextual relationship of words in a conversation.
Frameworks like Google's SpeakEasy AI and Amazon’s Alexa have reached phenomenal accuracy levels, making voice recognition a core part of their offerings. Deep Learning has further mitigated the challenge of accent recognition, demonstrating state-of-the-art results.
future prospects and Conclusion
The impressive stride of Deep Learning applications in image and voice recognition technologies promises immense future prospects. With the evolution of newer algorithms, greater computational power, and additional data, one can expect more sophisticated models, capable of achieving more nuanced tasks.
AI, powered by Deep Learning, is redefining the global technology landscape. Its applications in image and voice recognition have impacted industries and consumers alike, paving the way for innovative solutions, truly embodying the current state of the art.