Data Privacy in AI Applications
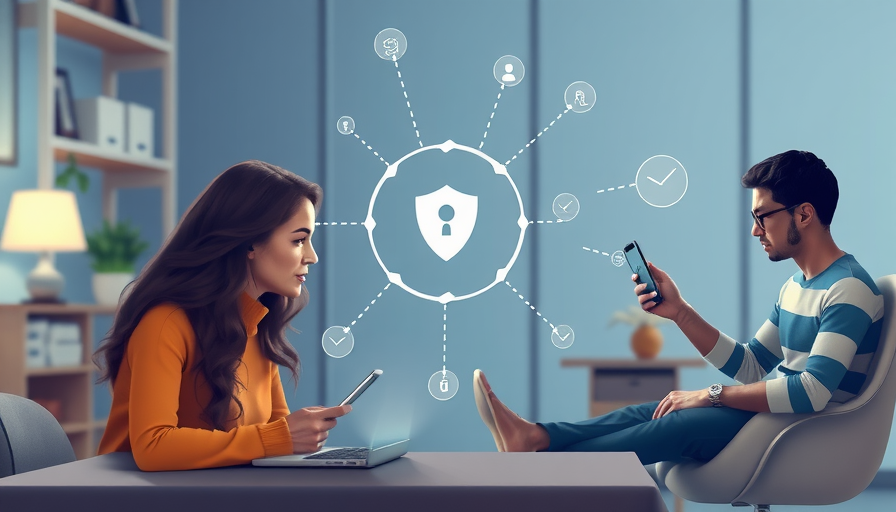
Artificial Intelligence (AI) has emerged as a transformative force across various industries, from healthcare to finance, enabling smarter decision-making, automating complex tasks, and providing personalized user experiences. However, the widespread adoption of AI technologies has raised significant concerns regarding data privacy. As AI systems often handle vast amounts of sensitive personal information, ensuring that this data remains secure is paramount. This comprehensive blog post will delve into the critical issue of data privacy within AI applications, exploring the risks, best practices, potential solutions, and real-world examples to illustrate these concepts.
Understanding Data Privacy in AI
data privacy refers to the protection of personal information collected, processed, and stored by AI systems. IT encompasses the measures taken to ensure that individuals' data is used responsibly, ethically, and securely. In the context of AI applications, data privacy involves several key aspects:
- data Collection: Understanding what data is being gathered and ensuring IT is done transparently.
- data Storage: Securing the data once IT has been collected to prevent unauthorized access.
- data Processing: Ensuring that data is processed in a way that respects user privacy and complies with relevant Regulations.
- data Sharing: Controlling how and with whom data is shared, if at all.
As AI technologies become more integrated into our daily lives, the importance of data privacy cannot be overstated. Let's examine some of the key risks associated with data privacy in AI applications.
Risks Associated with Data Privacy in AI
-
data Breaches: AI systems often handle sensitive information, making them attractive targets for cyberattacks. A data breach can result in the unauthorized access and exposure of personal data, leading to identity theft, financial loss, and reputational damage.
-
Unauthorized Access: Poorly secured AI applications can lead to unauthorized access to personal data. This can occur through various means, such as weak passwords, Phishing attacks, or exploits in the AI software itself.
- Example: In 2019, a security flaw in a popular smart home device allowed hackers to access and control users' cameras and microphones remotely.
-
Misuse of data: Even if data is secure, there's a risk that IT could be misused by the organization or third parties. This can include selling data without consent, using data for purposes not disclosed to the user, or making incorrect decisions based on biased AI algorithms.
- Example: Facebook's Cambridge Analytica scandal involved the misuse of personal data from millions of users, which was harvested without consent and used for political advertising.
-
Inference Attacks: In some cases, even anonymized data can be re-identified through inference attacks, where attackers use auxiliary information to deduce the identity of individuals in a dataset.
- Example: Researchers have demonstrated that IT's possible to re-identify individuals in supposedly anonymous datasets by cross-referencing them with publicly available information.
-
Bias and Discrimination: AI algorithms can inadvertently perpetuate or even amplify existing biases present in their training data, leading to discriminatory outcomes.
- Example: facial recognition systems have been shown to perform poorly on people of color due to biases in the training data, leading to incorrect identifications and potential harm.
best practices for Ensuring Data Privacy
To mitigate these risks, organizations should adopt a set of best practices for ensuring data privacy in AI applications:
-
Transparent data Collection: Clearly inform users about what data is being collected, how IT will be used, and who will have access to IT. This can be achieved through concise and easily understandable privacy policies.
-
Strong encryption: Use robust encryption methods to protect data both at rest (stored data) and in transit (data being transmitted). This ensures that even if data is intercepted, IT cannot be easily read or used.
- Example: End-to-end encryption is used by messaging apps like WhatsApp to ensure that only the communicating users can read the messages.
-
Regular Audits: Conduct regular security audits and vulnerability assessments to identify and address potential weaknesses in AI systems.
- Example: Banks often undergo regular penetration testing to identify and fix security vulnerabilities in their online banking platforms.
-
Access Controls: Implement strict access controls to ensure that only authorized personnel can access sensitive data. This can include role-based access control (RBAC) and multi-factor authentication (MFA).
- Example: healthcare organizations use access controls to restrict who can view or modify patient records, ensuring that only authorized staff can access this sensitive information.
-
compliance with Regulations: Ensure that AI applications comply with relevant data protection Regulations, such as the General data Protection regulation (GDPR) in Europe, the California Consumer privacy Act (CCPA), and others.
- Example: Companies like Microsoft have implemented comprehensive compliance programs to ensure their products adhere to various global data protection laws.
-
data Minimization: Collect and store only the data that is necessary for the intended purpose. This reduces the amount of sensitive information that could be exposed in a breach and helps minimize potential misuse.
- Example: A fitness app might collect only basic health metrics like steps taken or calories burned, rather than detailed medical history.
-
pseudonymization and anonymization: Use Techniques like pseudonymization (replacing personal data with artificial identifiers) and anonymization (removing personally identifiable information) to protect user privacy.
- Example: Anonymized datasets are often used in research to protect the identities of participants while still allowing valuable insights to be gained.
Potential solutions
In addition to best practices, several technical solutions can help enhance data privacy in AI applications:
-
differential privacy: Implement Techniques like differential privacy to add noise to data, making IT harder for attackers to infer personal information while still enabling useful analysis.
- Example: Apple uses differential privacy in its iOS operating system to collect usage statistics from users without compromising individual privacy.
-
Federated learning: Use Federated learning methods to train AI models without exchanging raw data between parties. This approach allows for collaborative model training while keeping data decentralized and secure.
- Example: Google's Gboard keyboard app uses Federated learning to improve its predictive text feature without transferring users' typing habits to central servers.
-
Homomorphic encryption: Employ homomorphic encryption, a form of encryption that allows computations to be carried out on ciphertext, generating an encrypted result which, when decrypted, matches the result of operations performed on the plaintext.
- Example: Medical research institutions could use homomorphic encryption to analyze patient data across multiple hospitals without ever decrypting or transferring sensitive information.
-
zero-knowledge proofs: Use zero-knowledge proofs to verify information without revealing the underlying data. This can be useful for authentication and authorization purposes while preserving privacy.
- Example: Zcash, a cryptocurrency, uses zero-knowledge proofs to ensure that transactions are valid without disclosing the sender, receiver, or amount.
-
differential privacy in Machine Learning: Apply differential privacy Techniques within Machine Learning algorithms to add noise during training and inference processes thereby protecting individual data points.
- Example: Google's RAPPOR system uses randomized aggregatable reporting to collect user statistics while preserving individual anonymity.
-
Blockchain technology: Use Blockchain technology to create secure, transparent, and tamper-proof records of data transactions, ensuring that data access and usage can be audited and verified.
- Example: MedRec, a Blockchain-based system for managing electronic health records, aims to give patients control over their medical data while ensuring its integrity and security.
-
privacy-Preserving Machine Learning: Implement Machine Learning models specifically designed with privacy-preserving Techniques in mind, such as secure multiparty computation (SMC) and homomorphic encryption.
- Example: The OpenMined project focuses on developing open-source Tools for privacy-preserving Machine Learning, enabling researchers to collaborate on sensitive data without compromising individual privacy.
Real-World Examples
To illustrate the importance of data privacy in AI applications, let's examine a few real-world examples:
-
healthcare: In healthcare, AI is used for various purposes, such as predicting disease outbreaks, personalizing treatment plans, and improving diagnostic accuracy. However, patient data is highly sensitive and must be protected to maintain confidentiality and trust.
- Example: A hospital might use AI to analyze electronic health records (EHRs) to predict which patients are at risk of developing certain conditions. To protect patient privacy, the hospital could use differential privacy Techniques to add noise to the data before performing the analysis.
-
Finance: In the finance industry, AI is employed for fraud detection, credit scoring, and algorithmic trading. However, financial data is highly sensitive, and breaches can result in significant financial loss and reputational damage.
- Example: A bank might use Federated learning to train a fraud detection model across multiple branches without sharing raw transaction data. This allows the bank to improve its model's accuracy while preserving customer privacy.
-
retail: In retail, AI is used for personalized recommendations, inventory management, and customer behavior analysis. However, consumer data must be protected to maintain trust and comply with Regulations.
- Example: An e-commerce platform might use homomorphic encryption to analyze customer purchase history without decrypting the data. This allows the platform to provide personalized recommendations while preserving customer privacy.
-
Social Media: Social media platforms use AI for content moderation, user engagement, and Targeted advertising. However, user data is highly sensitive, and breaches can result in significant harm.
- Example: A social media platform might use zero-knowledge proofs to verify users' identities without revealing their personal information. This allows the platform to prevent fake accounts while preserving user privacy.
data privacy in AI applications is a complex issue that requires a multi-faceted approach. By understanding the risks, adopting best practices, and exploring potential solutions, organizations can ensure that their AI systems protect user data effectively. As AI continues to evolve, IT's crucial to stay informed about emerging threats and innovations to maintain robust data privacy standards.
data privacy is not just a technical challenge but also an ethical one. Organizations must prioritize transparency, accountability, and respect for user rights when developing and deploying AI applications. By doing so, they can build trust with their users and contribute to a more secure and responsible AI ecosystem.