AI's Role in Predictive Maintenance for Datacenters
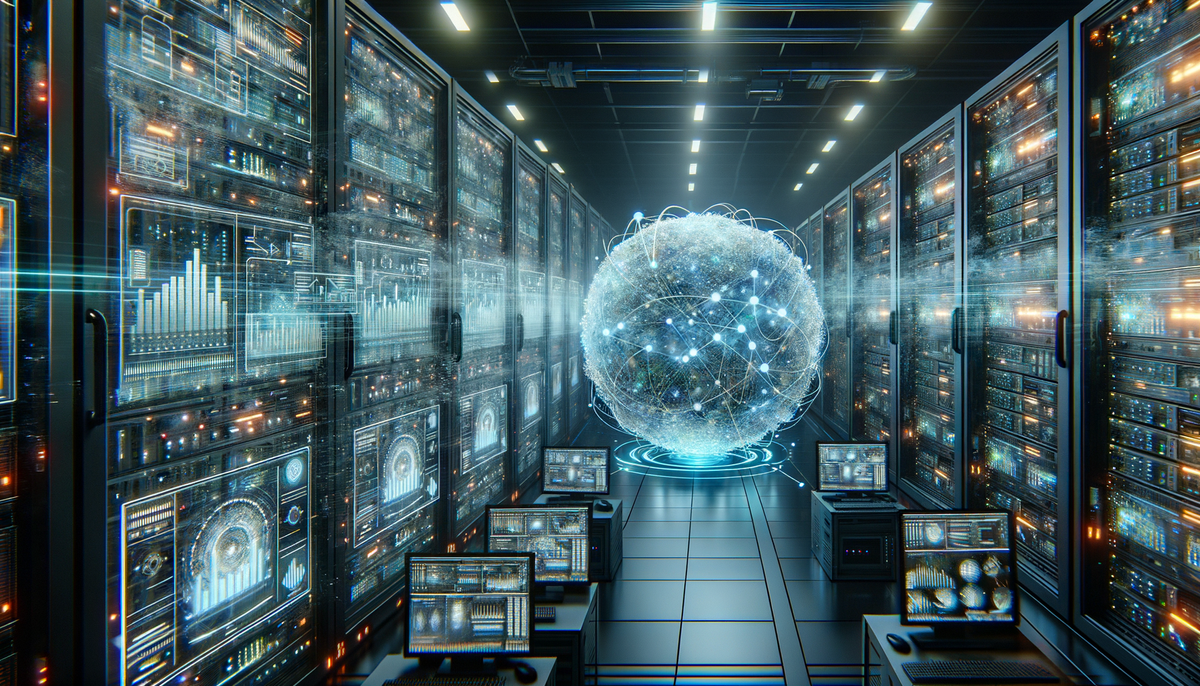
The rapidly evolving digital landscape has placed datacenters at the heart of today’s information-driven economy. As businesses strive to maintain seamless operations, the demand for reliable datacenter infrastructure has intensified. In this context, predictive maintenance powered by Artificial Intelligence (AI) emerges as a game-changer that ensures uptime reliability and operational efficiency.
Understanding Predictive Maintenance
Predictive maintenance is a Proactive approach to managing and maintaining equipment. Unlike traditional maintenance strategies, which often involve routine checks or reactive fixes after a failure, predictive maintenance analyzes historical and real-time data to predict when equipment failures might occur. This predictive capability allows businesses to perform maintenance activities just before issues arise, minimizing downtime and reducing repair costs.
Why Datacenters Need Predictive Maintenance
Datacenters are the backbone of modern IT operations. They house critical hardware systems that store, process, and manage vast amounts of data. The sheer complexity of these infrastructures, which include servers, cooling systems, power supplies, and networking equipment, makes them susceptible to various operational challenges. Unplanned downtime in datacenters can lead to massive financial losses, erode customer trust, and damage brand reputation. Therefore, maintaining high reliability and availability is crucial.
The Role of AI in Transforming Predictive Maintenance
1. data Collection and Management
AI technologies are integral in gathering immense amounts of data from diverse sources within a datacenter Environment. sensors embedded within datacenter equipment continuously monitor various parameters such as temperature, humidity, power consumption, and hardware performance metrics. AI algorithms efficiently process and analyze these data streams, transforming raw data into actionable insights.
2. advanced predictive analytics
AI employs advanced predictive analytics to model and foresee potential failures. Machine learning—a subset of AI—utilizes historical data to train models on what constitutes normal operational behavior. By setting sophisticated baselines, AI systems can detect anomalies indicative of potential failures. These systems adaptively refine their predictions over time to enhance accuracy, ensuring that maintenance teams receive timely alerts before problems escalate.
3. Real-Time Monitoring and Alerts
With AI-powered monitoring systems, datacenter operators gain access to real-time insights into equipment health. AI solutions continuously scrutinize sensor data, identifying subtle changes or trends that may signify emerging issues. This Proactive approach enables the automation of alert systems that notify technicians of potential problems, allowing for swift, informed action.
4. Resource optimization
AI’s Predictive Capabilities allow datacenter operators to optimize their resources more effectively. By precisely predicting when maintenance is needed, resources can be allocated based on priority, reducing the unnecessary expenditure associated with blanket maintenance policies. This approach extends the lifespan of equipment, enhances operational efficiency, and leads to substantial cost savings.
5. Enhanced decision-making
AI technologies provide actionable insights and optimization recommendations, aiding decision-makers in crafting more informed strategies concerning equipment life-cycle management, energy management, and overall operational planning. With AI, datacenter operators can make data-driven decisions that align with organizational goals and sustainability metrics.
Benefits of AI-Driven Predictive Maintenance in Datacenters
1. Reduced Downtime
One of the most tangible benefits of AI in predictive maintenance is the significant reduction in unplanned downtime. By foreseeing potential points of failure, maintenance teams can address issues before they cause system outages, maintaining continuity of service and Enhancing customer satisfaction.
2. cost efficiency
Implementing AI-driven predictive maintenance reduces the costs associated with emergency repairs and operational disruptions. AI enables precise scheduling of maintenance activities, thus minimizing the expenses linked to overtime labor and expedited service charges.
3. Extended equipment lifespan
AI helps in monitoring equipment health and identifying factors that could degrade performance. Preventive actions can be taken well in advance, which not only preserves equipment integrity but also extends its operational lifespan.
4. Improved Energy efficiency
energy management is a critical component for datacenters, which consume large amounts of electricity. AI can identify underperforming or energy-inefficient equipment that may detract from sustainability efforts. By optimizing the performance of cooling systems and computational resources, AI contributes to improved energy efficiency.
5. Scalable solutions
AI technologies provide scalable solutions that cater to datacenters of all sizes. As businesses grow and their IT infrastructure evolves, AI-driven predictive maintenance can easily adapt to new requirements and complexities, facilitating smooth Scalability.
challenges and Considerations
Despite the advantages, integrating AI into predictive maintenance for datacenters is not without challenges. Initial implementation can be complex and resource-intensive, requiring investments in sensor technologies and data management systems. Additionally, ensuring data security and privacy is critical, particularly in an Environment where sensitive data is handled.
Datacenter operators must also address potential skill gaps. The adoption of AI technology necessitates expertise in data science, machine learning, and IT operations, underscoring the importance of training and talent acquisition initiatives.
future trends in AI and Predictive Maintenance
As AI continues to evolve, its role in predictive maintenance is expected to grow. emerging technologies such as edge computing, which processes data closer to the source, could enhance Real-time analytics capabilities in datacenters. Furthermore, developments in AI explainability and interpretability aim to offer clearer insights into the decision processes of AI models, increasing trust in AI-driven maintenance frameworks.
Another key trend is the integration of AI with Internet of Things (IoT) devices. As more IoT devices are incorporated into datacenters, they will serve as additional data sources, enriching the AI's understanding and further refining predictive maintenance strategies.
Conclusion
AI's integration into predictive maintenance represents a pivotal advancement in datacenter management. Through advanced data analytics, real-time monitoring, and predictive insights, AI significantly enhances operational efficiency and reliability. As businesses continue to rely heavily on datacenters for their critical operations, the deployment of AI-driven predictive maintenance solutions promises to provide a robust foundation for future growth and stability, shaping the next generation of digital infrastructure.
By embracing AI, datacenters can transform from being a reactive Environment to a predictive, efficient, and agile operation, securing their role as indispensable assets in the digital age.