AI's Next Leap: How MCP & A2A Are Powering Smarter AI Agents
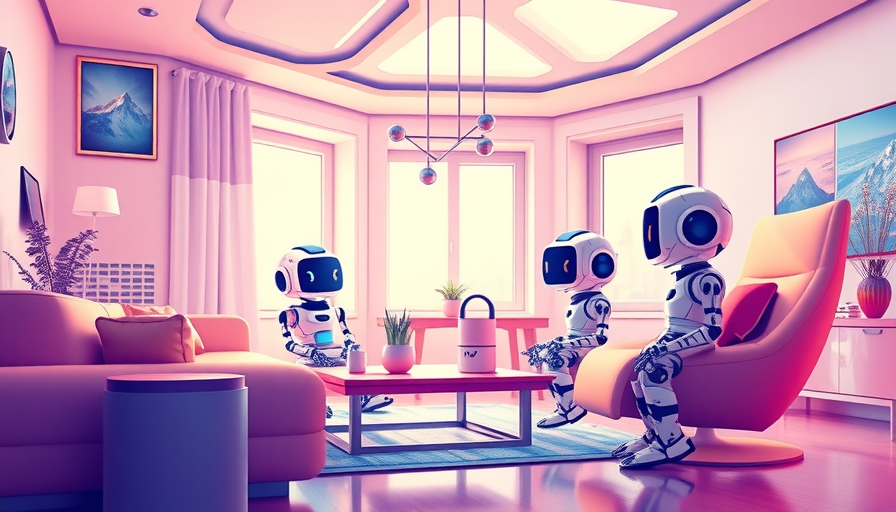
The landscape of artificial intelligence (AI) is rapidly evolving, with new technologies and protocols emerging to enhance the capabilities of AI agents. Two groundbreaking innovations, Model Context Protocol (MCP) and Agent 2 Agent Protocol (A2A), are at the forefront of this transformation. These protocols are revolutionizing how AI agents interact, learn, and perform, paving the way for smarter and more efficient AI systems.
Understanding Model Context Protocol (MCP)
Model Context Protocol (MCP) is a cutting-edge framework designed to improve the contextual understanding of AI models. By providing a structured way to integrate contextual information, MCP enables AI agents to make more informed decisions. This protocol ensures that AI models can better comprehend the nuances of data, leading to more accurate and relevant outputs.
The Importance of Context in AI
Context is crucial in AI because it provides the background information needed to interpret data accurately. Without context, AI agents may misinterpret data, leading to inaccurate or irrelevant responses. For example, consider an AI agent tasked with analyzing customer feedback. Without context, the agent might struggle to understand the sentiment behind the feedback, leading to inaccurate insights.
Imagine a customer leaves feedback saying, The product was great, but the delivery was late. Without context, an AI agent might focus solely on the positive sentiment of great and overlook the negative sentiment of late. However, with contextual understanding, the agent can recognize that the customer is expressing mixed feelings, providing a more nuanced analysis.
How MCP Enhances Contextual Understanding
MCP addresses this challenge by providing a framework for integrating contextual information into AI models. This protocol allows AI agents to understand the context behind data, making their responses more accurate and contextually appropriate. For instance, an AI agent using MCP can analyze customer feedback in the context of the customer's purchase history, interaction with customer service, and overall satisfaction, providing a more nuanced understanding of the feedback.
Technical Aspects of MCP
MCP works by creating a contextual layer that sits between the data input and the AI model. This layer processes the data to extract contextual information, which is then fed into the AI model along with the raw data. The contextual layer can include various types of information, such as:
- Temporal Context: Information about the time and sequence of events. For example, in a customer service scenario, the temporal context might include the time of day the customer contacted support, the duration of the interaction, and the sequence of events leading up to the contact.
- Spatial Context: Information about the location and environment. For instance, in a logistics scenario, the spatial context might include the geographical location of a delivery, the weather conditions, and the traffic patterns.
- User Context: Information about the user or entity interacting with the AI agent. This could include demographic information, preferences, and past interactions.
Example: MCP in Healthcare
In the healthcare sector, MCP can significantly enhance the accuracy of diagnoses and treatment plans. Consider an AI agent tasked with diagnosing a patient's condition. With MCP, the agent can consider the patient's medical history, lifestyle factors, and environmental conditions. For example, if a patient presents with symptoms of a cough and fever, the AI agent can use MCP to consider factors such as the patient's recent travel history, exposure to allergens, and current medications. This contextual information allows the agent to provide a more accurate diagnosis and personalized treatment plan.
Key Benefits of MCP
-
Enhanced Contextual Awareness: MCP allows AI agents to understand the context behind data, making their responses more accurate and contextually appropriate. For example, an AI agent analyzing medical data can consider the patient's medical history, lifestyle, and environmental factors, providing a more comprehensive diagnosis.
-
Improved Decision-Making: With better contextual understanding, AI agents can make more informed decisions, leading to better outcomes. For instance, an AI agent in the finance sector can consider market trends, economic indicators, and historical data to make more accurate investment decisions.
-
Seamless Integration: MCP can be easily integrated into existing AI systems, enhancing their capabilities without requiring a complete overhaul. This makes it a practical solution for organizations looking to improve their AI systems without significant disruption.
The Power of Agent 2 Agent Protocol (A2A)
Agent 2 Agent Protocol (A2A) is another game-changer in the AI realm. This protocol facilitates direct communication and collaboration between AI agents, enabling them to share information and work together more effectively. A2A breaks down the silos that traditionally isolate AI agents, fostering a more collaborative and efficient AI ecosystem.
The Need for AI Collaboration
In many scenarios, a single AI agent may not have all the information or capabilities needed to complete a task. This is where collaboration becomes crucial. By working together, AI agents can share insights, data, and capabilities, leading to more effective and efficient task completion.
Example: A2A in Manufacturing
Consider a manufacturing plant where multiple AI agents are responsible for different aspects of the production process. One AI agent might be tasked with monitoring the machinery, while another is responsible for quality control, and yet another handles inventory management. Without collaboration, these agents might operate in silos, leading to inefficiencies and potential errors.
With A2A, these agents can communicate and collaborate in real-time. For instance, if the machinery monitoring agent detects a potential issue, it can immediately alert the quality control agent to inspect the affected products and the inventory management agent to adjust the production schedule. This collaboration ensures that issues are addressed quickly and efficiently, minimizing downtime and waste.
How A2A Facilitates Collaboration
A2A provides a standardized way for AI agents to communicate and collaborate. This protocol allows AI agents to share information, request assistance, and coordinate their actions, leading to more effective task completion. For example, consider a scenario where an AI agent tasked with diagnosing a medical condition needs additional information. Using A2A, the agent can request this information from another AI agent specializing in medical data analysis, leading to a more accurate diagnosis.
Technical Aspects of A2A
A2A works by establishing a communication layer that enables AI agents to exchange messages and data. This layer supports various types of communication, including:
- Request-Response: One AI agent sends a request to another agent, which responds with the required information or action. For example, an AI agent might request data from another agent or ask it to perform a specific task.
- Publish-Subscribe: AI agents can publish messages to a shared channel, and other agents can subscribe to receive these messages. This allows agents to share information and updates in real-time.
- Peer-to-Peer: AI agents can communicate directly with each other, exchanging messages and data without the need for a centralized server. This enables fast and efficient communication, even in decentralized systems.
Example: A2A in Logistics
In the logistics industry, A2A can significantly improve the efficiency of delivery operations. Consider a scenario where multiple AI agents are responsible for different aspects of the delivery process, such as route planning, vehicle maintenance, and customer communication. With A2A, these agents can collaborate to optimize delivery routes, ensure vehicles are well-maintained, and keep customers informed about delivery status.
For instance, if an AI agent detects a traffic jam on the planned delivery route, it can use A2A to alert the route planning agent to find an alternative route and the customer communication agent to inform the customer about the delay. This collaboration ensures that deliveries are completed on time and customers are kept informed, improving overall satisfaction.
Key Benefits of A2A
-
Enhanced Collaboration: A2A allows AI agents to communicate directly, sharing insights and data to achieve common goals. For instance, AI agents in a manufacturing plant can collaborate to optimize production processes, leading to increased efficiency and reduced downtime.
-
Increased Efficiency: By working together, AI agents can complete tasks more quickly and accurately, improving overall efficiency. For example, AI agents in a logistics company can collaborate to optimize delivery routes, leading to faster and more efficient deliveries.
-
Scalability: A2A supports the scalability of AI systems, making it easier to add new agents and expand capabilities. This is crucial for organizations looking to grow their AI systems over time.
The Synergy of MCP and A2A
When combined, MCP and A2A create a powerful synergy that takes AI capabilities to the next level. MCP ensures that AI agents have a deep understanding of the context, while A2A enables them to collaborate and share this understanding. This combination results in smarter, more efficient, and more effective AI agents.
Real-World Applications
-
Healthcare: AI agents powered by MCP and A2A can provide more accurate diagnoses and personalized treatment plans by understanding patient context and collaborating with other medical AI agents. For example, an AI agent analyzing a patient's symptoms can consider the patient's medical history and lifestyle factors (thanks to MCP) and collaborate with other AI agents specializing in different medical fields (thanks to A2A) to provide a comprehensive diagnosis.
Detailed Example: Diagnosing a Complex Medical Condition
Imagine a patient presents with symptoms of fatigue, weight loss, and joint pain. An AI agent using MCP can consider the patient's medical history, including past diagnoses, medications, and lifestyle factors, to gain a deeper understanding of the symptoms. However, the agent might still need additional insights to make an accurate diagnosis.
Using A2A, the agent can collaborate with other AI agents specializing in different medical fields. For instance, it can request input from an AI agent specializing in rheumatology to assess the joint pain, an AI agent specializing in endocrinology to evaluate the weight loss, and an AI agent specializing in hematology to analyze blood test results. By combining these insights, the AI agents can provide a comprehensive diagnosis and recommend a personalized treatment plan.
-
Finance: In the financial sector, AI agents can make better investment decisions and detect fraud more effectively by leveraging contextual data and collaborating with other AI systems. For instance, an AI agent analyzing market trends can consider economic indicators and historical data (thanks to MCP) and collaborate with other AI agents specializing in different financial areas (thanks to A2A) to make more accurate investment decisions.
Detailed Example: Detecting Fraudulent Transactions
Consider an AI agent tasked with detecting fraudulent transactions in a financial institution. Using MCP, the agent can consider the context of each transaction, including the customer's transaction history, geographical location, and typical spending patterns. This contextual understanding allows the agent to identify anomalies that might indicate fraud.
However, detecting complex fraud schemes often requires collaboration with other AI agents. Using A2A, the agent can collaborate with AI agents specializing in different types of fraud, such as identity theft, money laundering, and credit card fraud. By sharing information and insights, these agents can detect complex fraud schemes that a single agent might miss.
-
Customer Service: AI-driven customer service can become more intuitive and responsive, providing personalized support by understanding customer context and collaborating with other AI agents. For example, an AI agent handling a customer inquiry can consider the customer's purchase history and interaction with customer service (thanks to MCP) and collaborate with other AI agents specializing in different areas of customer service (thanks to A2A) to provide a more personalized and effective response.
Detailed Example: Resolving a Complex Customer Inquiry
Imagine a customer contacts a company's customer service with a complex inquiry about a recent purchase. An AI agent using MCP can consider the customer's purchase history, past interactions with customer service, and overall satisfaction to gain a deeper understanding of the inquiry. However, resolving the inquiry might require input from multiple departments.
Using A2A, the AI agent can collaborate with other AI agents specializing in different areas of customer service. For instance, it can request input from an AI agent specializing in order management to check the status of the purchase, an AI agent specializing in technical support to address any product issues, and an AI agent specializing in billing to resolve any payment-related questions. By combining these insights, the AI agents can provide a comprehensive and personalized response to the customer's inquiry.
The future of AI is bright, and MCP and A2A are leading the way. By enhancing contextual understanding and fostering collaboration, these protocols are powering smarter AI agents that can tackle complex tasks with ease. As these technologies continue to evolve, we can expect even more innovative applications and improvements in AI capabilities. Stay tuned for the next leap in AI, powered by MCP and A2A.