AI in Predictive Maintenance: Transforming Equipment Upkeep for the Future
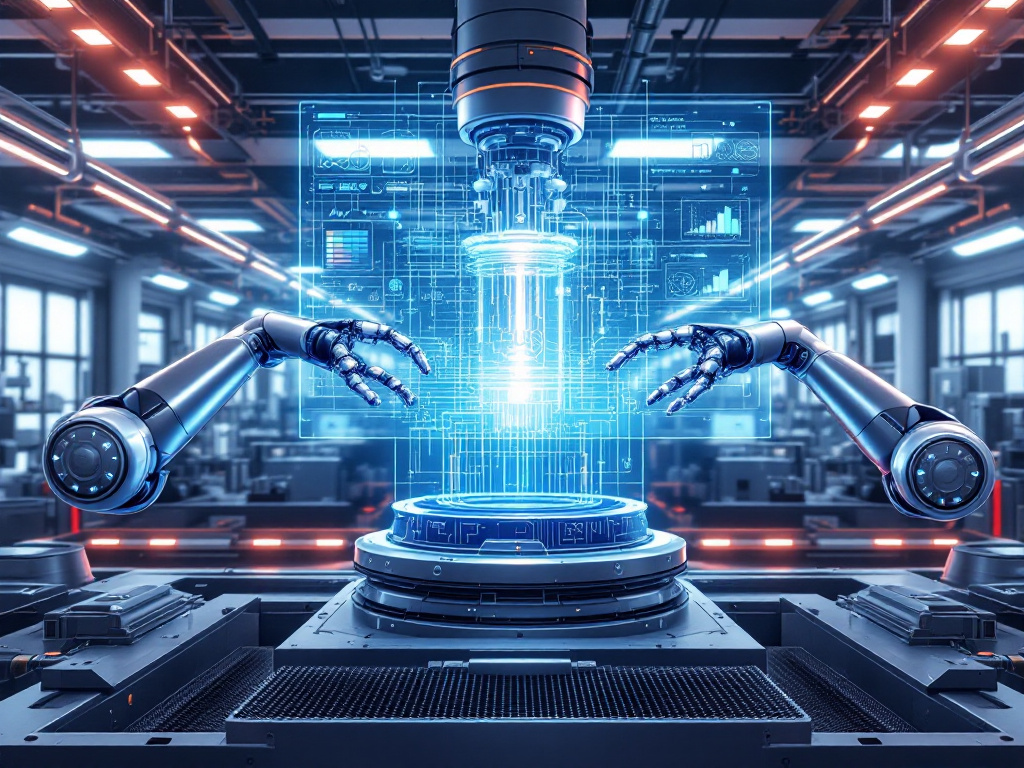
In today's rapidly evolving industrial landscape, the integration of artificial intelligence (AI) into maintenance operations has transformed the way we predict and prevent equipment failures. AI-driven predictive maintenance represents a proactive approach that leverages advanced data analytics and machine learning algorithms to forecast potential issues before they occur, ensuring optimal equipment performance and minimizing downtime.
The Evolution of Maintenance Strategies
Traditional maintenance strategies have often been reactive, focusing on fixing issues as they arise. However, this approach can lead to unexpected failures, increased costs, and reduced equipment lifespan. Predictive maintenance, on the other hand, utilizes real-time data and advanced analytics to anticipate equipment failures, allowing for timely interventions and proactive measures.
Reactive vs. Preventive vs. Predictive Maintenance
-
Reactive Maintenance: This involves fixing equipment only after it breaks down. While it may seem cost-effective in the short term, it can lead to significant downtime and higher repair costs in the long run. For example, a manufacturing plant that waits for a conveyor belt to fail before replacing it may face production delays and increased repair expenses. Reactive maintenance is often suitable for non-critical assets where the cost of maintenance outweighs the cost of failure. However, for critical equipment, this approach can be detrimental to overall operational efficiency.
-
Preventive Maintenance: This strategy involves scheduling regular maintenance tasks to prevent failures based on historical data and manufacturer recommendations. Although it reduces the risk of unexpected breakdowns, it may not be the most efficient use of resources. For instance, changing the oil in a machine every three months regardless of its condition can lead to unnecessary maintenance activities and costs. Preventive maintenance is effective for equipment with well-understood failure modes and clear maintenance intervals but may not account for variable operating conditions.
-
Predictive Maintenance: By continuously monitoring equipment performance and analyzing data, predictive maintenance can identify patterns and anomalies that indicate potential failures. This allows for proactive interventions, reducing downtime and extending equipment life. For example, sensors on a manufacturing line can detect unusual vibrations in a motor, alerting maintenance teams to perform repairs before a failure occurs. Predictive maintenance is ideal for critical equipment where the cost of failure is high, and real-time monitoring can provide significant benefits.
The Role of AI in Predictive Maintenance
AI plays a crucial role in enhancing the accuracy and efficiency of predictive maintenance systems. By analyzing vast amounts of data from sensors and monitoring systems, AI algorithms can detect early warning signs of equipment degradation or malfunction, enabling timely interventions.
Key Components of AI in Predictive Maintenance
-
Data Collection and Analysis: AI solutions require continuous access to historical and current data to evaluate machine performance. This data includes information about specific machine performance, operating conditions, and any deviations from the norm. For example, temperature sensors on a production line can provide real-time data on equipment heat levels, which AI algorithms can analyze to detect overheating issues. Data collection involves various sensors, including temperature, vibration, pressure, and flow sensors, which continuously monitor equipment parameters.
-
Machine Learning Algorithms: These algorithms analyze data to identify patterns and anomalies indicative of impending failures. They can learn over time, improving their accuracy and effectiveness in predicting equipment issues. For instance, an AI system can learn from past data that a specific motor tends to fail after operating at high speeds for extended periods, allowing it to predict future failures under similar conditions. Machine learning algorithms include supervised learning, unsupervised learning, and reinforcement learning, each with its unique applications in predictive maintenance.
-
Real-Time Monitoring: AI-powered systems can monitor equipment performance in real-time, allowing for immediate detection of potential problems and prompt corrective actions. For example, an AI system monitoring a fleet of trucks can detect unusual engine temperatures in real-time, alerting drivers to take immediate action to prevent breakdowns. Real-time monitoring enables continuous assessment of equipment health, ensuring that issues are addressed promptly before they escalate.
Benefits of AI-Powered Predictive Maintenance
Implementing AI in predictive maintenance offers several advantages for industries:
-
Cost Savings: By addressing potential equipment failures before they occur, AI-driven predictive maintenance can reduce the need for costly emergency repairs and minimize downtime. For example, a manufacturing plant can avoid the high costs of replacing a failed conveyor belt by performing timely repairs based on AI predictions. Cost savings are achieved through reduced maintenance costs, lower repair expenses, and minimized production downtime.
-
Increased Reliability: Proactive maintenance interventions ensure that equipment operates at optimal levels, reducing the risk of unexpected breakdowns and improving overall reliability. For instance, an AI system can predict when a critical component in a power plant is likely to fail, allowing for timely maintenance and preventing power outages. Increased reliability leads to consistent equipment performance and reduced operational disruptions.
-
Extended Equipment Life: By preventing premature failures and optimizing maintenance schedules, AI-powered predictive maintenance can extend the lifespan of equipment, maximizing return on investment. For example, an AI system can predict when a wind turbine's blades need maintenance, extending their operational life and reducing replacement costs. Extended equipment life results in lower capital expenditures and improved asset utilization.
-
Improved Operational Efficiency: Analyzing real-time data and predictive analytics allows AI algorithms to prioritize tasks and allocate resources more effectively, enhancing operational efficiency and productivity. For instance, an AI system can optimize the maintenance schedule for a fleet of delivery trucks, ensuring that vehicles are serviced at the most efficient times to minimize disruptions. Improved operational efficiency leads to better resource utilization, reduced waste, and increased productivity.
Industry Applications
AI-driven predictive maintenance has applications across various industries, including:
-
Manufacturing: AI can optimize production processes, improve overall equipment effectiveness, and reduce downtime in manufacturing operations. For example, an AI system can monitor the performance of assembly line robots, predicting when components are likely to fail and scheduling maintenance to prevent production delays. In manufacturing, AI-powered predictive maintenance can enhance product quality, reduce waste, and improve overall equipment effectiveness (OEE).
-
Energy Grids: AI-based optimization algorithms can analyze data from smart meters, weather forecasts, and energy consumption patterns to predict electricity demand and supply fluctuations, preventing unplanned outages. For instance, an AI system can predict when a transformer is likely to fail due to high demand, allowing for proactive maintenance and preventing power disruptions. In energy grids, AI can improve grid stability, reduce energy losses, and enhance the integration of renewable energy sources.
-
Logistics and Fleet Management: AI technologies provide real-time insights into vehicle performance, driver behavior, and operational processes, enabling fleet managers to make data-driven decisions and optimize their operations. For example, an AI system can monitor the performance of delivery trucks, predicting when maintenance is needed to prevent breakdowns and ensuring timely deliveries. In logistics and fleet management, AI can improve route optimization, reduce fuel consumption, and enhance vehicle maintenance schedules.
Detailed Examples of AI in Predictive Maintenance
Manufacturing
In a manufacturing setting, AI-powered predictive maintenance can significantly enhance operational efficiency and reduce downtime. For instance, consider a manufacturing plant that produces automotive components. The plant uses AI to monitor the performance of its assembly line robots. Sensors installed on the robots collect data on parameters such as temperature, vibration, and motor current. This data is continuously analyzed by AI algorithms to detect any anomalies that may indicate potential failures.
If the AI system detects unusual vibrations in one of the robots, it can alert the maintenance team to investigate the issue. The AI algorithm may identify that the vibrations are caused by a worn-out bearing, which, if left unaddressed, could lead to a complete failure of the robot. By predicting this failure in advance, the maintenance team can schedule a timely repair during a planned maintenance window, avoiding unplanned downtime and ensuring continuous production.
Energy Grids
In the energy sector, AI-driven predictive maintenance can improve the reliability and efficiency of energy grids. For example, consider a power utility company that uses AI to monitor the performance of its transformers. Sensors installed on the transformers collect data on parameters such as temperature, voltage, and current. This data is analyzed by AI algorithms to detect any anomalies that may indicate potential failures.
If the AI system detects abnormal temperature readings in one of the transformers, it can alert the maintenance team to investigate the issue. The AI algorithm may identify that the high temperatures are caused by a faulty cooling system, which, if left unaddressed, could lead to a transformer failure and subsequent power outage. By predicting this failure in advance, the maintenance team can schedule a timely repair, preventing unplanned outages and ensuring reliable power supply to customers.
Logistics and Fleet Management
In the logistics and fleet management sector, AI-powered predictive maintenance can enhance vehicle reliability and reduce maintenance costs. For example, consider a logistics company that uses AI to monitor the performance of its fleet of delivery trucks. Sensors installed on the trucks collect data on parameters such as engine temperature, oil pressure, and tire pressure. This data is analyzed by AI algorithms to detect any anomalies that may indicate potential failures.
If the AI system detects unusual engine temperature readings in one of the trucks, it can alert the fleet manager to investigate the issue. The AI algorithm may identify that the high engine temperatures are caused by a clogged radiator, which, if left unaddressed, could lead to engine overheating and subsequent breakdown. By predicting this failure in advance, the fleet manager can schedule a timely repair, preventing unplanned downtime and ensuring that deliveries are made on time.
The integration of AI in predictive maintenance is revolutionizing equipment upkeep, offering a proactive approach to identifying and addressing potential issues before they escalate. By leveraging advanced data analytics and machine learning algorithms, AI-powered predictive maintenance systems can enhance operational efficiency, reduce costs, and extend equipment life, ensuring a more reliable and sustainable future for industries. As AI technologies continue to evolve, their application in predictive maintenance will become even more critical, enabling organizations to achieve unprecedented levels of operational excellence and competitive advantage.