AI in Pharmaceuticals: Revolutionizing Drug Discovery and Development
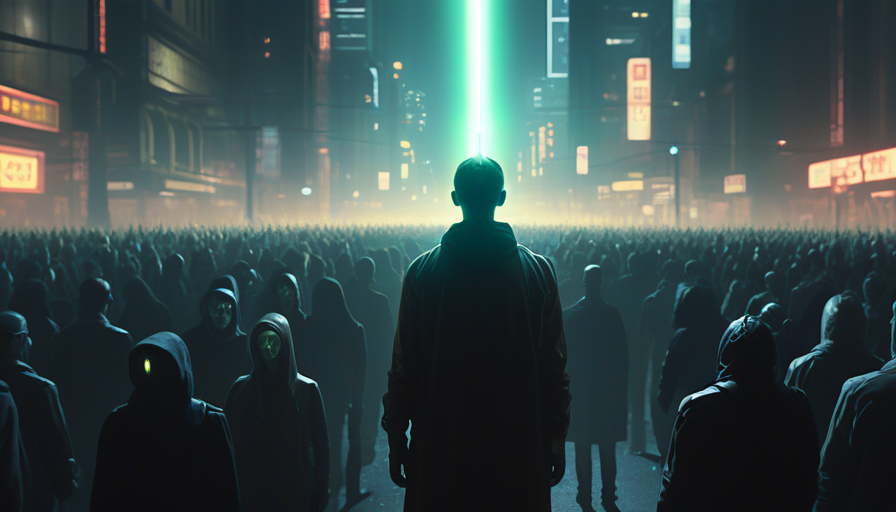
The pharmaceutical industry stands at the cusp of transformation, driven by advancements in Artificial Intelligence (AI). The traditional drug discovery and development process has been notoriously lengthy, costly, and fraught with uncertainties. Historically spanning over a decade and requiring billions of dollars, IT involves complex stages from target identification to clinical trials. However, AI is ushering in a new era marked by efficiency, Precision, and unprecedented speed. This blog post explores how AI is revolutionizing the pharmaceutical landscape, particularly focusing on drug discovery and development.
Understanding drug discovery and Development
Before delving into AI’s impact, IT's essential to grasp what drug discovery and development entail. These processes traditionally consist of several phases:
- Target Identification: Identifying a biological target associated with a disease.
- Lead Compound Identification: Finding molecules that can interact with the target effectively.
- Preclinical Testing: Assessing safety and efficacy in laboratory settings.
- clinical trials (Phases I-III): Conducting human trials to establish safety, dosage, and effectiveness.
- Regulatory Approval: Gaining approval from regulatory bodies such as the FDA.
Each phase is integral yet time-consuming, with high failure rates at each step, making Innovation crucial for efficiency gains.
AI's Role in drug discovery
Accelerating Target Identification
AI algorithms excel at parsing vast datasets to identify potential drug targets. machine learning models can analyze genetic information and disease pathways more swiftly than traditional methods. For instance, AI Tools can sift through genomic data to pinpoint genes or proteins involved in diseases like cancer or Alzheimer’s.
Genomic analysis: AI-driven genomic analysis involves using machine learning algorithms to interpret complex genetic data. These algorithms can identify patterns and correlations that might be missed by human researchers. For example, AI can analyze single-cell RNA sequencing data to understand how different cell types respond to various stimuli, providing insights into disease mechanisms.
Protein-Protein Interactions: Understanding protein-protein interactions is crucial for identifying potential drug targets. AI models can predict these interactions based on structural and functional data, helping researchers identify key proteins involved in disease pathways.
Enhancing Lead Compound Identification
Once a target is identified, the next step is finding lead compounds that can modulate this target. Traditional methods involve high-throughput screening of thousands of compounds—a process both costly and time-intensive. AI accelerates this through predictive modeling, where machine learning algorithms are trained on existing chemical libraries to predict new molecules' interactions with biological targets.
Virtual Screening: Virtual screening uses Computational Models to simulate the interaction between a compound and its target. This approach allows researchers to screen millions of compounds in silico, identifying those most likely to bind effectively to the target. AI enhances this process by improving the accuracy of Predictive Models and reducing False positives.
De Novo Drug design: De novo drug design involves creating new molecules from scratch based on desired properties. AI algorithms can generate novel chemical structures that are optimized for specific targets. This approach is particularly useful when existing compounds have limitations or side effects.
Improving Drug design
AI is not just about finding leads but also optimizing them. Generative Adversarial Networks (GANs) and Reinforcement Learning can be employed to design new molecules with desired properties, such as increased binding affinity or reduced toxicity. This iterative process of drug design significantly enhances the efficiency and effectiveness of pharmaceutical research.
Generative Models: Generative models like GANs can create new molecular structures by learning from existing data. These models can generate a vast array of potential compounds, which can then be screened for desired properties. This approach allows researchers to explore chemical space more comprehensively than traditional methods.
Reinforcement Learning: Reinforcement Learning involves training AI models to make decisions based on feedback. In drug design, Reinforcement Learning algorithms can optimize molecular structures by iteratively improving their properties. This approach enables the creation of highly effective and specific compounds tailored to particular targets.
Preclinical Testing
Preclinical testing is a critical phase where potential drugs are evaluated for safety and efficacy in laboratory settings. AI enhances this process by providing more accurate predictions and reducing the need for extensive animal testing.
In Silico Modeling: In silico modeling uses computational Simulations to predict how a compound will behave in biological systems. AI algorithms can improve the accuracy of these models, providing more reliable predictions about a drug's safety and efficacy. This approach reduces the reliance on animal testing and accelerates the preclinical phase.
Toxicology prediction: Predicting toxicity is essential for ensuring that potential drugs are safe for human use. AI models can analyze molecular structures to predict toxic effects based on known data. This allows researchers to identify and eliminate compounds with high toxicity early in the development process, saving time and resources.
AI in clinical trials
clinical trials are the final stage before a drug reaches the market, involving rigorous testing in human subjects. AI enhances this phase by improving trial design, patient recruitment, and data analysis.
Trial design and optimization
AI can optimize clinical trial designs by identifying the most effective protocols and endpoints. machine learning algorithms can analyze historical trial data to predict outcomes and suggest improvements.
Adaptive Trial Designs: Adaptive trial designs use real-time data to modify trial parameters as IT progresses. AI models can help design adaptive trials that are more flexible and responsive, improving efficiency and reducing costs. For example, AI can adjust dosing regimens or patient cohorts based on interim results, ensuring that the trial remains on track.
Endpoint Selection: Selecting appropriate endpoints is crucial for evaluating a drug's efficacy. AI algorithms can analyze clinical data to identify the most relevant endpoints, ensuring that trials provide meaningful insights into a drug's effectiveness.
Patient Recruitment and Retention
Recruiting and retaining patients for clinical trials is often challenging and time-consuming. AI can streamline this process by identifying suitable candidates and predicting dropout rates.
Patient Matching: AI models can analyze patient data to identify individuals who are most likely to benefit from a particular trial. This approach ensures that trials include the right participants, improving outcomes and reducing costs. For example, AI can match patients based on genetic profiles, medical history, and other relevant factors.
Retention Strategies: Predicting dropout rates is essential for maintaining trial integrity. AI algorithms can analyze patient data to identify those at risk of dropping out and suggest retention strategies. This approach helps ensure that trials remain fully enrolled and provide reliable results.
data analysis
clinical trials generate vast amounts of data, which must be analyzed to draw meaningful conclusions. AI enhances this process by providing more accurate and efficient data analysis Tools.
real-time monitoring: real-time monitoring uses AI algorithms to analyze trial data as IT is collected. This approach allows researchers to identify trends and anomalies in real time, enabling quicker decision-making and adjustments. For example, AI can detect adverse events or efficacy signals early, allowing for timely interventions.
predictive analytics: predictive analytics involves using machine learning models to forecast future outcomes based on historical data. In clinical trials, predictive analytics can help researchers anticipate trial results, identify potential issues, and optimize study designs. This approach ensures that trials are conducted efficiently and provide valuable insights.
case studies
IBM Watson Health
IBM’s Watson for drug discovery leverages Natural Language Processing (NLP) and machine learning to analyze vast scientific literature and datasets. This AI platform assists researchers by identifying novel insights into disease mechanisms and suggesting potential therapeutic candidates.
Literature analysis: Watson can process millions of research papers, patents, and clinical trial reports to extract relevant information. By understanding the context and relationships within this data, Watson helps researchers identify new targets and compounds that have been overlooked in traditional approaches.
Knowledge Graphs: Watson uses knowledge graphs to visualize complex relationships between different entities, such as genes, proteins, and diseases. These visualizations provide researchers with a comprehensive view of disease mechanisms, enabling them to develop more targeted therapies.
DeepMind's AlphaFold
DeepMind’s AlphaFold, an AI system that predicts protein structures with high accuracy, has been transformative in understanding diseases at a molecular level. Accurate protein models help identify drug targets more effectively, potentially accelerating the development of treatments for complex conditions like cystic fibrosis and certain cancers.
Protein Structure prediction: AlphaFold uses Deep Learning algorithms to predict the three-dimensional structure of proteins based on their amino acid sequences. This approach provides researchers with detailed insights into how proteins function and interact, enabling the development of more effective drugs.
drug discovery: By understanding protein structures, researchers can design compounds that specifically target disease-causing proteins. AlphaFold's predictions have already led to significant advancements in drug discovery, including the identification of new targets for diseases like Alzheimer's and Parkinson's.
BenevolentAI
BenevolentAI uses AI to accelerate drug discovery by analyzing vast amounts of biomedical data. The company has successfully identified potential treatments for various diseases, including COVID-19.
drug repurposing: BenevolentAI uses machine learning models to identify existing drugs that could be repurposed for new indications. By analyzing clinical trial data and scientific literature, the company can quickly identify promising candidates for further investigation.
COVID-19 research: During the COVID-19 pandemic, BenevolentAI used its AI platform to analyze biomedical data related to SARS-CoV-2. The company identified baricitinib, an existing rheumatoid arthritis drug, as a potential treatment for COVID-19. This discovery was later validated in clinical trials, demonstrating the power of AI in accelerating drug development.
Ethical Considerations
While AI offers numerous benefits for pharmaceutical research, IT also raises ethical considerations that must be addressed to ensure responsible use.
data privacy and security
AI relies on large datasets, which often contain sensitive patient information. Ensuring data privacy and security is crucial for maintaining public trust and compliance with Regulations.
data Anonymization: Anonymizing patient data ensures that individuals cannot be identified from the information used in AI models. This approach protects patient privacy while allowing researchers to analyze valuable data.
Secure Storage: Secure storage solutions, such as encrypted databases and access controls, protect sensitive data from unauthorized access. Ensuring robust security measures is essential for maintaining data integrity and confidentiality.
bias and fairness
AI models can inadvertently perpetuate biases present in the training data, leading to unfair outcomes. Addressing bias and ensuring fairness are critical for ethical AI use in pharmaceutical research.
Bias Detection: Detecting biases in AI models involves analyzing the data used for training and identifying any patterns that could lead to unfair outcomes. This approach ensures that AI models are fair and unbiased, providing accurate results for all patients.
inclusive data: Including diverse patient populations in clinical trials and datasets helps ensure that AI models are representative of the broader population. This approach reduces the risk of bias and improves the generalizability of research findings.
transparency and accountability
transparency and accountability are essential for building trust in AI-driven pharmaceutical research. Ensuring that AI models are transparent and accountable helps maintain public confidence and regulatory compliance.
Explainable AI: Explainable AI involves developing models that can provide clear explanations for their decisions. This approach ensures that researchers and regulators understand how AI models arrive at their conclusions, Enhancing transparency and accountability.
Regulatory compliance: Ensuring compliance with regulatory guidelines is crucial for ethical AI use in pharmaceutical research. Regulators must establish clear standards for AI-driven drug development, including requirements for data privacy, bias detection, and transparency.
Future Directions
The future of AI in pharmaceutical research holds immense potential for transforming drug discovery and development. emerging technologies and advancements are poised to further enhance the efficiency and effectiveness of this field.
Advanced machine learning Techniques
Advanced machine learning Techniques, such as deep Reinforcement Learning and Generative Adversarial Networks (GANs), offer new possibilities for drug discovery and development.
Deep Reinforcement Learning: Deep Reinforcement Learning involves training AI models to make decisions based on feedback from complex environments. This approach can optimize molecular structures and trial designs, leading to more effective drugs and efficient trials.
Generative Adversarial Networks (GANs): GANs consist of two Neural Networks that compete against each other to generate new data. In drug discovery, GANs can create novel molecular structures with desired properties, expanding the chemical space explored by researchers.
integration with Other technologies
Integrating AI with other emerging technologies, such as CRISPR Gene Editing and nanotechnology, holds promise for developing innovative therapies and diagnostics.
CRISPR Gene Editing: Combining AI with CRISPR Gene Editing enables precise genetic modifications that can correct disease-causing mutations. AI models can identify optimal targets for CRISPR interventions, Enhancing the effectiveness of gene therapy approaches.
nanotechnology: nanotechnology involves manipulating matter at the atomic or molecular scale to create materials and devices with unique properties. Integrating AI with nanotechnology allows researchers to design more effective drug delivery systems and diagnostic Tools, improving patient outcomes.
personalized medicine
AI-driven personalized medicine tailors treatments to individual patients based on their genetic profiles, medical history, and other relevant factors. This approach ensures that patients receive the most effective therapies, minimizing side effects and improving outcomes.
Genomic analysis: AI models can analyze genomic data to identify mutations and variations associated with specific diseases. This information enables researchers to develop targeted therapies tailored to individual patients' genetic profiles.
Precision Medicine: Precision medicine involves using detailed patient data to guide treatment decisions. AI-driven Precision medicine leverages machine learning algorithms to analyze complex datasets, providing personalized recommendations for diagnosis and treatment.
AI is revolutionizing pharmaceutical research by accelerating drug discovery, Enhancing clinical trial efficiency, and enabling personalized medicine. While ethical considerations must be addressed, the potential benefits of AI in this field are vast. As technology continues to advance, integrating AI with other emerging technologies holds promise for developing innovative therapies and diagnostics that improve patient outcomes and transform healthcare.