AI-Driven Cloud Resource Optimization
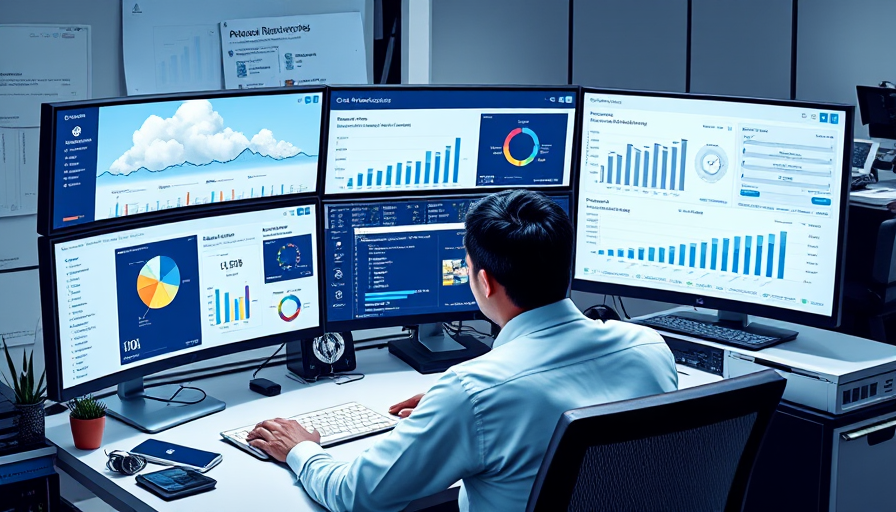
In today's world, organizations are increasingly leveraging Cloud Computing to streamline operations, enhance scalability, and drive innovation. However, managing cloud resources efficiently can be a daunting task, often leading to wasted expenditure and underutilized infrastructure. This is where AI-driven cloud resource optimization comes into play. By integrating Artificial Intelligence with Cloud Management, businesses can achieve significant cost savings, improved performance, and a more agile IT Environment.
What is AI-Driven Cloud Resource optimization?
AI-driven cloud resource optimization utilizes advanced Artificial Intelligence algorithms to analyze usage patterns, predict future demands, and automatically adjust resources accordingly. This process involves several key components:
- data Collection: AI systems gather data from various sources such as application logs, monitoring Tools, user behavior analytics, and performance metrics.
- Pattern Recognition: Machine Learning algorithms analyze this data to identify usage patterns, detect anomalies, and predict future demand.
- Resource Adjustment: Based on these predictions, the system automatically adjusts cloud resources, ensuring that only the necessary amount of compute power, storage, and network bandwidth is allocated.
By harnessing the power of Machine Learning and predictive analytics, businesses can transform their cloud resource management strategies, making them more dynamic and responsive to changing needs.
The Benefits of AI-Driven Cloud Resource optimization
1. Cost efficiency
One of the primary advantages of AI-driven cloud resource optimization is the reduction in Operational Costs. Traditional Cloud Management often results in over-provisioning resources to handle peak loads, leading to unnecessary expenses during off-peak times. AI algorithms can identify underutilized resources and suggest right-sizing or scaling down options, thereby eliminating these wasted expenditures.
Example: A financial services company might use AI to analyze its transaction processing workloads. During regular business hours, the system detects high CPU usage and automatically scales up resources. Outside of business hours, IT scales down, ensuring that the company only pays for what IT uses.
2. Performance Improvement
By dynamically adjusting resources based on real-time demand, organizations can ensure optimal performance for their applications. This leads to better user experiences, reduced latency, and increased reliability.
Example: An e-commerce platform experiences spikes in traffic during holiday seasons. AI-driven optimization can predict these spikes and preemptively allocate additional compute resources, ensuring that the website remains responsive and reliable even under heavy load.
3. scalability
AI-driven solutions enable seamless scalability by automatically provisioning additional resources during peak times and de-provisioning them when not needed. This ensures that the infrastructure can handle varying workloads efficiently without manual intervention.
Example: A streaming service like Netflix uses AI to manage its content delivery networks (CDNs). During popular events or new content releases, the system scales up CDN capacity to handle increased viewer demand, then scales down afterward to save costs.
4. Resource Utilization
AI can help in better utilization of resources by identifying idle or underutilized instances and suggesting actions like stopping, resizing, or terminating them. This not only saves cost but also frees up resources for other critical workloads.
Example: A software development company might have multiple virtual machines (VMs) running continuously for development and testing purposes. AI can identify VMs that are idle for extended periods and suggest shutting them down during non-working hours, thereby saving on compute costs.
5. predictive maintenance
AI algorithms can predict potential failures or performance degradation in cloud resources by analyzing historical data and real-time metrics. This allows for proactive maintenance, reducing downtime and improving overall system reliability.
Example: A healthcare provider uses AI to monitor its cloud-based electronic health record (EHR) system. The AI detects unusual patterns in storage usage and predicts a potential disk failure, allowing the IT team to replace the disk before IT causes any disruption in service.
6. compliance and security
AI can help ensure compliance with regulatory standards by continuously monitoring resource usage and detecting anomalies that might indicate security breaches or non-compliance issues. This Proactive approach enhances the overall security posture of cloud environments.
Example: A financial institution uses AI to monitor its cloud Environment for suspicious activities, such as unauthorized access attempts or data exfiltration. The AI system can automatically alert security teams and initiate remediation actions, ensuring compliance with industry Regulations like GDPR or PCI-DSS.
How Does IT Work?
AI-driven cloud resource optimization works through a combination of Machine Learning models, data analytics, and Automation. Here’s a detailed breakdown of the process:
1. data Collection
The first step involves gathering data from various sources within the cloud Environment. This data can include:
- Application Logs: Detailed records of application activities, errors, and performance metrics.
- Monitoring Tools: Real-time data from monitoring Tools like AWS CloudWatch, Azure Monitor, or Google Stackdriver.
- User Behavior Analytics: Insights into user interactions with applications, helping to understand demand patterns.
- Performance Metrics: Key performance indicators (KPIs) such as CPU usage, memory consumption, disk I/O, and network throughput.
2. Pattern Recognition
Machine Learning algorithms analyze the collected data to identify usage patterns, detect anomalies, and predict future demand. This involves:
- Time-Series analysis: Examining historical data to identify trends and seasonal variations.
- anomaly detection: Using statistical methods or Machine Learning models to detect unusual activities that might indicate issues or opportunities for optimization.
- Predictive Modeling: Employing Techniques like regression analysis, decision trees, or neural networks to forecast future resource requirements.
3. Resource Adjustment
Based on the predictions and insights generated from data analysis, the AI system automatically adjusts cloud resources. This can include:
- Auto-Scaling: Dynamically scaling up or down compute instances based on current and predicted load.
- Right-Sizing: Recommendations for resizing VMs or containers to match actual resource needs.
- load balancing: Distributing traffic across multiple resources to ensure even utilization and high availability.
4. continuous improvement
AI-driven optimization is an iterative process that involves continuous monitoring, learning, and adjustment. The system continuously collects new data, refines its models, and improves its predictions over time.
Example: A retail company uses AI to optimize its cloud resources during Black Friday sales. Initially, the AI predicts a certain level of traffic based on historical data. As the event unfolds, the AI adjusts its predictions in real-time, scaling up resources as needed and providing insights into customer behavior that can inform future planning.
Real-World applications
Many leading organizations have already started implementing AI-driven cloud resource optimization to great effect:
Netflix
The streaming giant uses AI to optimize its content delivery networks (CDNs). By analyzing viewer data and predicting demand, Netflix ensures smooth video playback and minimizes latency. This not only improves the user experience but also reduces bandwidth costs.
Amazon Web Services (AWS)
AWS employs Machine Learning models to recommend the right instance types and sizes through services like AWS Trusted Advisor and AWS Compute Optimizer. These Tools help users save on cloud costs by identifying underutilized resources and suggesting optimal configurations.
Microsoft Azure
Azure cost management and Billing use AI to provide insights into spending patterns and recommend cost-saving measures. The platform's Machine Learning algorithms analyze usage data to identify areas where resources can be optimized, helping organizations reduce their cloud expenditure.
Google cloud platform (GCP)
GCP's Recommendations feature uses AI to suggest ways to optimize resource usage. For example, IT can recommend the use of preemptible VMs for non-critical workloads, significantly reducing costs without compromising performance.
best practices for Implementing AI-Driven Cloud Resource optimization
1. Start with a Pilot Project
Begin by implementing AI-driven optimization in a small, controlled Environment to measure its effectiveness. This allows you to gather data, identify potential issues, and refine your approach before rolling IT out more widely.
Example: A manufacturing company might start by optimizing the cloud resources used for its IoT data processing workloads. By monitoring the results of this pilot project, they can assess the benefits and challenges before expanding the optimization to other areas of their IT infrastructure.
2. Leverage Existing Tools
Utilize existing Cloud Management Tools that offer built-in AI capabilities, such as AWS Trusted Advisor, Azure cost management, or Google Cloud Recommendations. These Tools provide valuable insights and recommendations based on your current usage patterns.
Example: A startup might use Azure cost management to identify underutilized VMs and receive recommendations for right-sizing or shutting down unnecessary resources. This can help them save costs without requiring extensive technical expertise.
3. Regular Monitoring and Adjustments
Continuously monitor the performance and cost savings achieved through optimization and make necessary adjustments. AI-driven systems should be regularly reviewed and updated to ensure they remain effective as your organization's needs evolve.
Example: A healthcare provider might use AI to optimize its cloud-based Telemedicine platform. By continuously monitoring usage patterns and adjusting resources, they can ensure that the platform remains responsive during peak times while minimizing costs during off-peak periods.
4. Integrate with DevOps Practices
Incorporate AI-driven optimization into your DevOps pipeline to automate the process of resource management. This ensures that optimization happens in real-time, reducing manual intervention and increasing efficiency.
Example: A financial services company might integrate AI-driven optimization with its CI/CD pipeline to automatically scale resources based on deployment activities. This ensures that new applications or updates are deployed without performance bottlenecks.
5. Train Your Team
Ensure that your IT team is well-versed in the principles of AI and cloud resource management. Providing training and resources can help them better understand how to implement and manage AI-driven optimization effectively.
Example: An e-commerce platform might invest in training programs for its DevOps team, focusing on AI and Machine Learning Techniques relevant to cloud resource management. This can enhance their ability to optimize the platform's performance and cost efficiency.
6. Implement Governance and compliance
Establish governance policies and compliance measures to ensure that AI-driven optimization aligns with your organization's security and regulatory requirements. This includes monitoring for anomalies, ensuring data privacy, and maintaining audit trails.
Example: A financial institution might implement governance policies to ensure that AI-driven optimizations comply with Regulations like GDPR or PCI-DSS. This can involve setting up Automated Compliance checks and regular audits to verify adherence to these standards.
AI-driven cloud resource optimization is not just a trend but a necessity for modern businesses aiming to maximize efficiency and reduce costs. By embracing this technology, organizations can achieve a more agile and responsive IT Environment, ensuring they stay competitive in an ever-evolving digital landscape.
The integration of AI with Cloud Management offers numerous benefits, from cost savings and performance improvements to enhanced scalability and better resource utilization. Real-world examples from companies like Netflix, AWS, Azure, and Google Cloud demonstrate the tangible advantages of adopting AI-driven optimization strategies.
To successfully implement AI-driven cloud resource optimization, IT is essential to follow best practices such as starting with a pilot project, leveraging existing Tools, regular monitoring, integrating with DevOps practices, training your team, and implementing governance and compliance measures. By doing so, organizations can unlock the full potential of their cloud investments and drive innovation in an increasingly competitive market.